6.1 Introduction to Sampling Distributions and the Central Limit Theorem
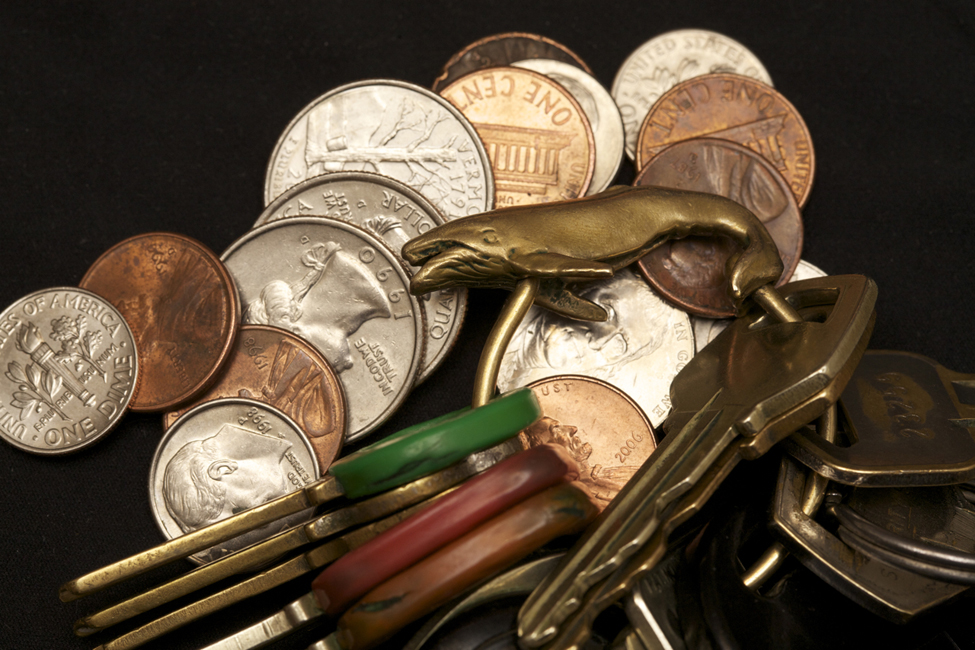
Why are we so concerned with means? Two reasons are that they give us a middle ground for comparison, and they are easy to calculate. In this chapter, we will study means, proportions and their relationship to the central limit theorem.
The central limit theorem is one of the most powerful and useful ideas in all of statistics. The central limit theorem basically says that if we collect samples of size [latex]n[/latex] from a population with mean [latex]\mu[/latex] and standard deviation [latex]\sigma[/latex], calculate each sample’s mean, and create a histogram of those means, then, under the right conditions, the resulting histogram will tend to have an approximate normal bell shape.
Watch this video: Central limit theorem | Inferential statistics | Probability and Statistics | Khan Academy by Khan Academy [9:45]
Attribution
“Chapter 7 Introduction” in Introductory Statistics by OpenStax is licensed under a Creative Commons Attribution 4.0 International License.