Considering Types of Data
13 Sensitive Data: Practical and Theoretical Considerations
Dr. Alisa Beth Rod and Kristi Thompson
Learning Outcomes
By the end of this chapter you should be able to:
- Define the following terms: de-identification, identifying information, sensitive data, Statistical Disclosure Risk Assessment.
- Recognize that defining risk levels for sensitive data (i.e., low, medium, high, very high) depends on the research context.
- Understand Canadian policies and ethics regulations related to research data.
Pre-assessment
Introduction
What are sensitive data? The Sensitive Data Toolkit for Researchers (Sensitive Data Expert Group of the Portage Network, 2020a) defines sensitive data as “information that must be safeguarded against unwarranted access or disclosure” and gives several examples. However, defining sensitive data this way raises the question: Why should this information be safeguarded? Looking at their examples can help us figure this out, as they include things like personal health information and other information deemed to be confidential, some geographic information (e.g., locations of endangered species), or data protected by institutional policy. What these examples have in common is risk — that people will have their confidentiality violated, that endangered species will be disturbed or hunted, that a policy will be broken. So, you might say that sensitive data are data that cannot be shared without potentially violating the trust of or risking harm to an individual, entity, or community.
In this chapter, we’ll talk about working with sensitive data within Canadian federal and provincial policy landscapes. (Indigenous data have ethical and ownership implications and are covered in their own chapter.) We’ll conclude by outlining options for safe preservation, sharing, and appropriate archiving of sensitive data.
Human Participant Data
In Canada, at the federal, provincial, and institutional levels, various legal, policy, and regulatory frameworks govern sensitive data involving humans. In most cases, these regulatory requirements are designed at a high level to protect human participants’ privacy and confidentiality. In this way, the regulatory frameworks related to sensitive data are relevant to the category of human participant data.
The Privacy Policy Landscape in Canada
It’s not always easy to know which privacy laws are applicable in each situation. The most important privacy regulations for research data are typically located at the provincial or territorial level of governance because universities fall outside of the scope of the two main federal-level privacy laws (Office of the Privacy Commissioner of Canada, 2018). However, some sensitive information, such as medical records, may be collected by university-affiliated researchers in partnership with private or public organizations, so these may fall under the federal Privacy Act, which applies to governmental organizations, or under the Personal Information Protection and Electronic Documents Act (PIPEDA), which applies to private sector commercial entities. The Canadian government has a helpful tool to determine which legislation applies to scenarios involving different types of sensitive information.
At the national level, Canada’s three federal funding agencies (also the agencies, Tri-Council, or Tri-Agency) have a policy statement on the ethical conduct for research involving humans (Tri-Council Policy Statement: Ethical Conduct for Research Involving Humans –TCPS 2) which stipulates the parameters related to privacy, justice, respect, and concern for the welfare of participants. The TCPS 2 also provides oversight for the governance of Research Ethics Boards (REBs), which are responsible for reviewing proposed research projects that rely on human participants. Contrary to the U.S., which has a federal law (HIPPA) that governs medical information, in Canada, the management of health records or clinical data is legislated at the provincial and territorial level. All provinces and territories have at least one privacy law that can be applied to research.
Traditionally, Canadian and other Western legal systems enshrine the rights of individuals (and by extension, corporations) to privacy, ownership over information, and protection from direct harm by research. However, data can be used to harm groups or communities — for example, to stigmatize racialized groups or sexual and gender minorities. (See Ross, Iguchi and Panicker, 2018.) Such harms are not adequately addressed in existing Canadian legislation and policy. An alternative model is ownership, control, access, and possession (OCAP®), a research protocol developed to protect First Nations interests, stewarded by the First Nations Information Governance Centre. OCAP® is “a set of specifically First Nations — not Indigenous — principles” and is not intended to be used in other contexts (First Nations Information Governance Centre, n.d.). However, the principles of considering community interests first can be applied to research with marginalized communities generally. To read more about Indigenous models of ethical research, please see the chapter “Indigenous Data Sovereignty.”
Several provinces are updating privacy laws, which will affect the management of research data involving human participants. For example, Québec adopted Law 25, (also referred to as the Privacy Legislation Modernization Act), to strengthen consent requirements, oversight, and compliance. Law 25 is modelled after the European Union’s General Data Protection Regulation (GDPR), widely considered the most protective privacy legislation in the world. Potential impacts of Law 25 include requiring consent for each specific secondary use of the research data, introducing the “right to be forgotten” (Wolford, 2018), and requiring a formal privacy impact assessment prior to transferring an individual’s data outside of Québec (Office of the Privacy Commissioner of Canada, 2020). We’ll discuss consent in more detail later in the section titled, “Consent Language and TCPS 2.” For now, the explanation of this legal shift is that, previously, researchers could ask for a “blanket” consent to use participants’ information (e.g., a sample from a patient in a clinical trial could be used for other studies without detailing those specific studies). However, Law 25 does not allow a blanket consent for future research. It requires a researcher to get consent every time the researcher wants to use the sample for a new purpose. Although Law 25 is specific to Québec, it provides a model regarding privacy law reform and could have sweeping implications for research data involving human participants.
Risk and Harm
Risk of violating confidentiality is created when information can isolate individuals in a dataset as distinct and can be matched to external information to identify them through reasonable effort. The level of harm this may inflict on a research participant depends on the population and topic of the data. Generally, the highest levels are easier to identify and define (e.g., if someone’s personal health information were made public). Children are considered a vulnerable population because they can’t give their own consent, so research involving children holds a high potential for causing severe harm if information were breached. Topics considered socially taboo also place research participants at higher risk of critical harm. Although the definitions of socially taboo topics vary across cultures and can be situational, the following are considered extremely sensitive, which raises the level of potential harm that a research participant could experience if their data were breached:
- drug or alcohol use (including cigarettes)
- details of sexual activity/STD status
- private family issues
- relationship/domestic violence
- loss or death in the family
- victimization status
- criminal/delinquent behaviour
- health-related questions/medical conditions/mental health questions
Vulnerable populations, such as the following, have a higher potential for harm from a data breach regardless of the research topic:
- Indigenous Communities
- racialized communities
- lower-income groups
- children/teens
- politically oppressed communities
Research involving humans from vulnerable populations and/or focusing on sensitive topics may require additional safeguards in terms of data storage and security. When research participants give permission for their identifying information to be shared (e.g., oral history interviews in which participants want to share their stories) or when information is collected from public sources where there is no reasonable expectation of privacy (e.g., lists of board members) then data may be shared without concern for disclosure. Otherwise, data need to be assessed for disclosure risk and shared only if risk falls below an acceptable threshold. There are a common set of curatorial and statistical measures to quantitatively assess and reduce the risk of a breach of confidentiality. The first step includes an analysis of the uniqueness of each individual’s data within the larger dataset.
Identifiers
Many people consent to have their information used for research purposes but don’t want their identities disclosed. Research data may contain direct identifiers, such as contact information of participants, student numbers, or other directly identifying information. Research data without direct identifiers still may have the potential to violate confidentiality due to indirect identifiers or quasi-identifiers — personal details that could in combination lead to disclosing the identity of an individual. These data can include surveys or interviews of participants who have consented to have the information they provide used for research purposes. Human participant data can also include information extracted from medical records, tax-filer records, social media, or any other sources of information on people.
Direct Identifiers
Direct identifiers place study participants at immediate risk of being re-identified. These include things like names and phone numbers but also less obvious details. For example, the U.S. Health Insurance Portability and Accountability Act (HIPAA) treats any geography containing less than 20,000 people as a direct identifier. Exact dates linked to individuals, such as birth dates, are also considered personally identifying.
The HIPAA has a list of 18 personal identifiers, while a set of guidelines from the British Medical Journal includes a list of 14 direct and 14 indirect identifiers based on international guidelines. From these and other sources, we have compiled the following list of direct identifiers for Canadian research. These should always be removed from data before public release unless research participants have agreed to have their identities shared (partial exceptions noted):
- Full or partial names or initials
- Dates linked to individuals, such as birth, graduation, or hospitalization (year alone or month alone may be acceptable)
- Full or partial addresses (large units of geography, such as city, fall under indirect identifiers and need to be reviewed)
- Full or partial postal codes (the first three digits may be acceptable)
- Telephone or fax numbers
- Email addresses
- Web or social media identifiers or usernames, such as X handles (formerly Twitter)
- Web or Internet protocol numbers, precise browser and operating system information (these may be collected by some types of survey software or web forms)
- Vehicle identifiers, such as licence plates
- Identifiers linked to medical or other devices
- Any other identifying numbers directly or indirectly linked to individuals, such as social insurance numbers, student numbers, or pet ID numbers
- Photographs of individuals or their houses or locations, or video recordings containing these; medical images or scans
- Audio recordings of individuals (Han et al., 2020)
- Biometric data
- Any unique and recognizable characteristics of individuals (e.g., mayor of Kapuskasing or Nobel prize winner)
In addition, any shared digital files, such as photographs or documents, should be checked for embedded information, such as username or location. (see Henne, Koch and Smith, 2014.)
Indirect Identifiers
It’s clear why direct identifiers pose a risk to confidentiality — if you have someone’s address or insurance number, it may be possible to violate their confidentiality. But what are indirect identifiers and why are they a problem? Indirect identifiers (also known as quasi-identifiers) are characteristics that do not identify individuals on their own but may, in combination, reveal someone’s identity. A variable should be considered a potential identifier (direct or indirect) only if it could be matched to information from another source to reveal someone’s identity.
It’s not possible to compile an exhaustive list of quasi-identifiers, but the following should always be considered:
- age (can be a direct identifier for the very elderly)
- gender identity
- income
- occupation or industry
- geographic variables
- ethnic and immigration variables
- membership in organizations or use of specific services
These variables need to be considered alongside any contextual information about the dataset — for example, survey documentation or published research may make it clear that the participants in the research lived in a particular area or worked in a particular profession.
The remaining variables in a dataset are nonidentifying information (not likely to be recognized as coming from specific individuals or not showing up in external databases). This can include opinions, ratings on Likert scales, temporary measures (such as resting heart rate), and others. These are not part of the confidentiality assessment but still need to be considered in the overall risk assessment. An issue with non-identifying variables is the level of sensitivity of the data — a dataset with confidential health information or a survey that asks sensitive questions about past behaviours needs to be treated with more care than a dataset of product ratings.
A set of records that has the same values on all quasi-identifiers is called an equivalence class. An equivalence class of 1 represents an individual who is unique in the dataset. Such a person may be at risk of being identified and is called a sample unique. If a study contains a complete sample of some population (e.g., everyone employed at a particular place) then this person is also a population unique on those characteristics (and their identity may be obvious to anyone familiar with the population). Correspondingly, members of a large equivalence class — one with 10, 20, or 50 members — are indistinguishable from each other and may not be identified based on their quasi-identifiers, so are not considered to be at risk of re-identification.
Now you know what quasi-identifiers are and that they can be used to narrow down the identity of survey respondents. So, what do you do about them? You could just delete them from the data the same as you do with direct identifiers, but doing so will seriously impact the ability of future researchers to use a dataset. Instead, you need to assess the quasi-identifiers to determine the level of risk.
As a first pass, a data curator might look at the variables in isolation and consider them in context with other information about the data. Quasi-identifying variables containing groups with small numbers of respondents (e.g., a religion variable with three responses of “Buddhism”) may be high risk. Unusual values (e.g., more than six children) can also pose high risk. These can be assessed by running frequencies on the data. However, the size of identifiable groups both in the survey and in the general population needs to be considered. There may be only one person from Winnipeg in your random-digit dialing survey, but if your survey doesn’t narrow it down any further, that person is safe.
A commonsense approach to data de-identification is to describe a person using only the values of the demographic variables in a dataset:
“I’m thinking of a person living in Ontario who is female, married, has a university degree, and is between the ages of 40 and 55.”
This person does not appear to be at risk — unless contextual information provides additional clues. For example, if this were a survey of professional hockey referees.
Unusual combinations of values for variables can pose difficulties. Age, education, and marital status may not seem to be identifying — but what if the dataset contains someone in the Under-17 age group who gave their marital status as divorced or their education as university graduate? That person could be recognizable and is an example of a hidden extreme value that would not show up if you ran frequencies on all the variables in the dataset. The more indirect identifiers in the data, the higher the probability of there being hidden unusual combinations, and the harder it is to check for them. What’s needed is a formal way of assessing the quasi-identifiers and quantifying the level of risk. This process is called statistical disclosure risk assessment.
Statistical Disclosure Risk Assessment
There are different techniques to gauge and limit the risk of re-identification, but the best known is k-anonymity, a mathematical approach to demonstrating that a dataset has been anonymized. It was first proposed by computer scientists in 1998 (Samarati and Sweeney) and has formed the basis of formal data anonymization efforts since then. The concept is that it should not be possible to isolate fewer than k individual cases in your dataset based on any combination of identifying variables — k is a number set by the researcher; in practice, it’s usually 5.
Imagine a survey of workers at a tool and die factory has three demographic variables: age group, gender, and ethnic group. If an individual in the dataset is not a visible minority, is male, and is between 25 and 30, then for the data to have k-anonymity with k=5, there must be at least four other individuals in the dataset with the same set of characteristics. This also must be true for every other individual in the dataset; each person must have at least four data twins.
In Figure 1, cases 1, 6, and 13 form an equivalence class of k=3. Each case in the equivalence class has two data twins. Even if an attacker knew that an individual was in the dataset and was able to match their characteristics against the data, they would not be able to tell which of the three cases was the target individual. Case 14 has no data twins — it is a sample unique.
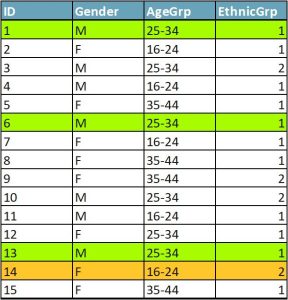
To achieve k-anonymity with a k of at least 5 in a dataset, use data reduction techniques, including global data reduction and local suppression. Global data reduction is making changes to variables across datasets, such as grouping responses into categories (e.g., age in 10-year increments). Local suppression means deleting individual cases or responses (e.g., deleting the “marital status” response of the participant under 17 years old rather than regrouping the otherwise nonrisky variables “marital status” and “age”).
It’s easy to check k-anonymity using standard statistical software, even though most packages don’t have built-in functions for doing so. The resource “De-identification Guidance” from the Digital Research Alliance of Canada (the Alliance) provides code for doing this in R and Stata.
k-anonymity is intended to guarantee data anonymization — that every record in the anonymized data will be indistinguishable from k minus 1 other records in the same dataset. However, research participants aren’t usually told that no one will know which line of the data file holds their confidential information. They are told their answers will be kept confidential. Even if a person’s record isn’t unique in the data, it may still be possible to figure out some confidential information about them.
Within a few years of k-anonymity being published as a solution to the privacy dilemma, researchers pointed to a serious possible flaw: the homogeneity attack. When values of sensitive attributes are the same for all members of an equivalence class (set of data twins), an attacker may be able to infer the attributes of survey respondents without identifying them. Let’s return to that sample survey of workers. Figure 2 shows you the demographic variables and one sensitive attribute, a question about whether the workers are in favour of forming a union. Cases 1, 6, and 13 still form an equivalence class with k=3. So even if you know which people match those characteristics, you can’t tell which person matches which case. But these three people answered the union question the same way. You now know how all of them answered this question. Confidentiality has been violated.
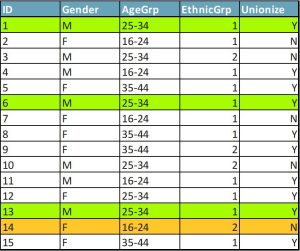
Extensions of k-anonymity, such as p-sensitive k-anonymity and l-diversity (Domingo-Ferrer and Torra, 2008), have been developed to deal with the attribute disclosure issue. However, implementing these is difficult and tends to degrade the research value of the dataset. Let’s consider one of the simpler variants.
A dataset has l-diversity when each group of records sharing a combination of demographic attributes has at least l different values for each confidential variable. In our example dataset, every group of data twins would need to include both “yes” and “no” responses to the union question, since 2 would be the maximum possible value for l for this question. And this would have to be true for some value of l for every confidential answer in the dataset. Now imagine a typical survey with dozens of questions — and each needs to be considered for l-diversity for each equivalence class. So, techniques like l-diversity are only practical to implement in datasets with very few variables.
The greatest threat of a homogeneity attack occurs when a dataset is a complete sample of some population. Imagine the dataset from the workplace survey is only a 25% sample of the population. This means there may be other people who hold the same values on quasi-identifiers as cases 1, 6, and 13, but they’re not in the dataset, so their views on forming a union are unknown. Assuming there’s no way of knowing whether an individual is in the data, being in this equivalence class no longer reveals anyone’s opinions. This is a reasonable assumption where a dataset is a small sample of a larger population and k-anonymity has been satisfied. Conversely, if a dataset is a complete sample of a population or contains a large fraction of it, it needs to be treated with extreme care — it’s almost impossible to be certain that such a dataset has been de-identified.
Hidden Identifiers
When testing for risk, consider the size of a dataset (number of participants and number of variables). With large datasets, attackers may be able to use machine learning approaches. Personal rankings and ratings are considered nonidentifiers; however, Zhang et al. (2012) describe a case where an artificial learning system was trained on a large collection of profiles containing movie rankings and was able to infer with some reliability which accounts had multiple users. It’s easy to imagine other attacks using related approaches — for example, comparing public book reviews on a site such as Goodreads to survey responses that include rankings of books used for trauma-informed book-based therapy. Thompson and Sullivan (2020) demonstrated another approach where unexpected variables could be used to potentially re-identify survey respondents, this time using an attack incorporating geographic information. They demonstrated that a variable showing distance from the nearest major city could be combined with the information that a survey respondent lived on a First Nations reserve to pinpoint the location of some respondents. This would be difficult to do by hand but was easy with a computer.
Cases like these demonstrate why there can never be a simple rules-based mechanic for de-identifying datasets. You’ll always need to consider the data in context with external information or data sources that may overlap with your data population and share some of the same information. Risk of re-identification happens when external information that an attacker may reasonably have access to can be linked to information in an archived dataset, and each dataset needs to be considered individually.
De-identifying Qualitative Data
We usually use statistical methods of anonymizing data on structured data, such as data in a spreadsheet. However, qualitative data are often stored and analyzed in unstructured formats (e.g., interview, focus group, or oral history transcripts in text, audio, or video format, or ethnographic observations detailed in field notes, etc.). It’s still possible to anonymize unstructured qualitative data types, and there are software programs and digital tools that may facilitate or automate this process to certain extents (for an excellent overview, see this panel talk on de-identifying qualitative data, available at: https://www.youtube.com/watch?v=MbKw3LR2rVo).
Sometimes a research participant inadvertently identifies themselves when responding to interview questions or discussing their lived experiences. For example, in a study in which a librarian interviews other librarians at universities, if someone names their institution (McGill) and job title (Research Data Management specialist) in their response, this combined information can reveal their identity. The challenge with qualitative data is that identifying information will not be contained in predetermined categories (e.g., age, religion, gender) so you may not be able to predict how much identifying information is in a dataset prior to data collection and analysis.
A researcher could delete identifying information, similar to approaches with structured data, but contextual information is often vital in qualitative studies, so more often a researcher will assign categorical codes to replace identifying information. The Finnish Social Science Data Archive (FSD) recommends using square brackets to denote cases where de-identification in a transcript has occurred, to avoid commonly used punctuation (Finnish Social Science Data Archive, 2020). For example, a researcher may replace a name with [Participant 1]. Or a specific location, such as Pohénégamook (a small village in Québec), may be replaced with [village]. If geographic context is important, then the code can be changed to reflect a general area rather than a specific village, such as [the Bas-Saint-Laurent region].
When redacting qualitative information or replacing detailed information with categories, document these decisions and the category definitions in a codebook that accompanies the dataset. For example, the researcher may decide to remove names of villages when the population of the village is less than 1,000 inhabitants. This requires a well-documented justification and definition for potential future reuse of the dataset.
Interview transcripts should be anonymized even if the researcher doesn’t intend to publish the data. This reduces the risk of harm in the case of a breach. Anonymization should be irreversible, and when anonymizing, researchers should consider both potential harm to participants if identifiable information were made public as well as the researcher’s ability to analyze the data at the necessary level of nuance. If the purpose of a research project is to analyze a sensitive topic, it might not make sense to de-identify the data, and the data may require additional safeguards.
Consent Language and TCPS 2
When curating human participant data, you must know what safeguards participants were offered and under what conditions any approving REBs permitted the research to take place. In Canada, ethical guidelines for human research participants are outlined in the TCPS 2 policy. At most institutions, the REB will scrutinize consent language more than any other component of an application to ensure participants’ privacy and confidentiality are preserved and that participants are informed about the scope and manner of their participation in the research. In accordance with TCPS 2 guidelines, consent forms should contain the following information:
- that their participation is voluntary
- that participants may withdraw from the research even after the study is underway
- a concise description of the study as well as the potential risks and benefits to participants, all in plain language (e.g., avoiding jargon) — especially important in studies that involve vulnerable populations; socially taboo topics; coercion (e.g., an incentive); and/or deception, in which a participant is not fully aware of the purpose of the research
- whether the data will be available to other researchers or to the public, under what conditions, in which specific repository,* and in what format or including what information (e.g., whether it may contain direct or quasi-identifiers)
*REBs may require that researchers identify the repository that will host or publish a data deposit containing human participant data. For example, an REB may require that data are stored or published only in repositories with servers located in Canada or only in a repository with access control (i.e., the ability to restrict access to specific individuals).
Consent forms should include language regarding the eventual deposit or publication of human participant data because researchers may intend to or be required to (e.g., by funders or journal mandates) make their data available following the publication of related research. Otherwise, if a researcher needs or wants to share data, they may be obligated to re-consent participants (amend the consent forms and ask participants to again consent to the study), which may prove difficult or impossible if all direct identifiers have been permanently anonymized.
Some resources provide a template or suggested language for consent forms and REB applications regarding the storing and sharing of human participant data. Digital Research Alliance of Canada (the Alliance) has a Sensitive Data Toolkit for Researchers (Sensitive Data Expert Group of the Portage Network, 2020b) with language you can use in consent forms to explain the following to participants: the difference between anonymity and confidentiality; barriers to withdrawing from the study; parameters for data reuse, including oversight processes (e.g., setting up data-use agreements or requiring potential future research projects to obtain REB permission prior to access to the data); whether the data could be used for other purposes outside of the original research topic; and whether the data or a version of them will be made available to the public. The following example for publishing data following the completion of a study shows some boilerplate language that can be adapted for use in cases where data is likely to be shared. The following example for publishing data following the completion of a study shows some boilerplate language that can be adapted for use in cases where data may need to be shared. The Sensitive Data Toolkit for Researchers (Sensitive Data Expert Group of the Portage Network, 2020b), has many additional examples of this type of language for different circumstances.
Funding agencies and publishers often ask researchers to make their data accessible upon completion of their study. Making research data available to others allows qualified researchers to reproduce scientific findings and stimulates exploration of existing datasets. To ensure confidentiality and anonymity, any shared data would be stripped of any information that could potentially identify a participant.
For additional resources and sample consent language, please refer to the extensive guides provided by the Inter-university Consortium for Political and Social Research (ICPSR) and the Finnish Social Science Data Archive.
The Qualitative Data Repository (QDR), based out of Syracuse University in New York, also has informed consent guidance related to qualitative studies, such as interviews or oral histories, where direct identifiers may be retained in the published dataset (Qualitative Data Repository, n.d.b). The QDR also has templates for the publication of archival materials and for getting consent from participants to release de-identified or identifiable data (Qualitative Data Repository, n.d.a). The following is from QDR for the deposit of potentially identifiable information:
Data generated from the information you provide in our interaction may be shared with the research community (most likely in digital form via the internet) to advance scholarly knowledge. Due to the nature of the information, full de-identification of those data might not be possible. As a result, other measures will be taken before sharing. I plan to deposit the data at REPOSITORY X, or at a similar social science domain repository. Your data will BE MADE AVAILABLE UNDER THE FOLLOWING ACCESS CONDITIONS. Despite my taking these measures it is not possible to predict how those who access the data will use them.
The Data Curation Network offers a comprehensive guide to curating human participant data, including how to review consent language. The data curation primer on human participants provides guidance on questions to ask as a repository owner or curator, including the consenting process, consent language, and whether there are gaps between the dataset and the consent language.
Other Categories of Sensitive Data
Human participant data are often considered to be the same thing as “sensitive data,” but some categories of sensitive data do not involve human participants and are equally important. When researchers collaborate with industry partners to develop technologies and inventions, data may be considered “trade secrets” and must be safeguarded according to contractual obligations (Government of Canada, 2021). Although in theory the pursuit of profits as a primary goal is antithetical to academic endeavors, these partnerships provide resources and infrastructure that would otherwise not be available via universities or public funding sources. For example, COVID-19 vaccines were rapidly developed because of partnerships between university researchers and private pharmaceutical companies.
Here are some other categories of sensitive data:
- intellectual property
- dual-use data
- data subject to import/export control
- third-party licensed data
- locations of endangered species
Intellectual property concerns may arise when data are associated with a pending patent application or research that could be patented or with other copyrighted information. Rights holders can decide whether to grant access or reuse of the data. When intellectual property is connected with potential revenue, it’s not typically released openly or shared. Here are some important considerations regarding intellectual property: who owns the data, the terms of use (or license) for the data, and any conditions for using or reusing the data. Chapter 12, “Planning for Open Science Workflows,” discusses intellectual property considerations in more detail.
Dual-use means data developed for civilian purposes may be used in military applications. For example, when facial recognition technology is developed for a smart phone, the underlying dataset could be used to train similar machine learning models to track political dissidents or deploy weaponized drones. Technical information about critical infrastructure is another example of sensitive data that are defined as dual-use. Canada has regulations and assessment procedures to determine whether research is dual-use and the subsequent level of safeguarding required.
Data that are subject to export/import control (controlled goods), are related to dual-use data in that they are data that have implications for military or intelligence use that may cross the Canadian border (Government of Canada, 2017). There are specific definitions of controlled goods involving weapons that come from the United States. These regulations exist to ensure that researchers are not participating in trafficking weapons or weapons technology whether intentionally or unintentionally.
Third-parties are any entity besides the researcher and the institution. A third party’s use of data requires a license from the data owner. For example, demographers may purchase datasets from Statistics Canada under terms that the data may be used by and shared with only other researchers at the same institution. Data-use agreements stipulate who can access the data, for what purpose(s), and when; where these data may be stored; whether any part of the data can be deposited; and whether the data should be destroyed or retained upon completion of the study. In most cases, these agreements prohibit the researcher from depositing or publishing the underlying dataset used for their research.
Location information about endangered species is a category of sensitive data because of the potential for malicious actors to use the information to harm these species. Consider a project where a researcher places digital geolocation tags on endangered rhinoceroses to track their movements. Poachers who gain access to this data could use it to pinpoint and hunt rhinoceroses, which is an extinction-level threat for this species.
Researchers don’t have to be as concerned about identification of participants when working with these additional categories of sensitive data, but they must be more concerned about safeguarding and cybersecurity measures, legal liabilities and responsibilities, and compliance. Research Data Management (RDM) for these types of data involve encrypted or password protected access (e.g., multifactor authentication, transmitting data securely via a Virtual Private Network (VPN)), secure data storage and backup, avoiding the use of personal devices to interact with the data, and performing a robust security audit to identify potential avenues for a breach.
Preserving and Sharing Sensitive Data
Some digital repositories allow for the deposit of sensitive data. Examples include the Inter-university Consortium for Political and Social Research (ICPSR), the Qualitative Data Repository (QDR), and the Finnish Social Science Data Archive. However, none in Canada currently allow for the deposit of sensitive data.
The Alliance is currently working on a multiyear pilot project to partner with Canadian universities and support the implementation of infrastructure for controlled access to sensitive data. The technology must comply with institutional, provincial, and federal policies and laws and must rely on infrastructure located in Canada. The controlled access project has developed a tool incorporating zero-knowledge encryption so that sensitive datasets can be transferred from a secure repository environment to researchers and vice versa. Zero-knowledge means that the administrators of a system do not have the key to decrypt files on their system. The encryption keys for the data are stored in an independent platform. A researcher who wants access to a sensitive dataset would download the encrypted data from the repository and then receive the password from the key management platform.
Many institutional data repositories at Canadian universities have access to an installation of Dataverse, with many of them using the Borealis Dataverse installation at Scholar’s Portal. Borealis terms of use prohibit sensitive data from being deposited. However, the consortium responsible for the development and maintenance of Borealis has determined that they will defer to REBs to define whether a dataset is sensitive or not. Even though “sensitive” is not a binary – data can be more or less sensitive – defining sensitivity for data deposit may involve complex calculations. Repositories may accept anonymized datasets containing human participants and may not define these as sensitive.
To preserve and share sensitive data, sometimes a researcher will retain data locally but publish a metadata record in their institutional Dataverse collection so other researchers can discover data and procedures for accessing them. Libraries can support this by creating a protected space isolated from the network for secure preservation and backup, where data may be deposited for long-term storage. The library would need to work with the depositing researcher to make sure appropriate access protocols are in place. Suggested deposit language is provided in the following box:
Deposit Form: Terms of Deposit, Retention, Sharing, and Reuse
The depositor grants the library the right to store and securely manage the data, including transforming, moving between platforms, and creating backup copies as necessary for preservation.
- indefinitely or until withdrawn
- until the following date, after which the data must be deleted
Can a record of this dataset be shared in <local archive> so that people can discover these data? If yes, please provide any restrictions on what documentation should be shared.
Indicate how and under what condition these data can be shared with researchers outside the original research team. Note that your original consent form, if applicable, must allow this reuse.
- Data can be shared only with the explicit permission of the following person or persons (e.g., depositor, members of original research team, data review committee, etc.).
- Please identify persons and provide contact information.
- Data can be shared by request if certain conditions are met (e.g., approval by research ethics board, completion of a secure Data Management Plan explaining how data will be kept secure during reuse project, signing of conditions document).
Please detail ethical restrictions for reuse — include, if applicable, a copy of the original consent form with the data deposit.
Conclusion
When calculating risk and harm, researchers must consider institutional, provincial, federal, and funder policies, laws, and regulations as well as disciplinary norms and contractual obligations. Consider also that harm may be experienced by multiple interested parties, including participants, the institution, the researcher, the community, the nation, and any other affiliated entities.
For this reason, many institutions formally classify sensitive data and define levels of risk and harm on a scale (e.g., very high, high, moderate, and low). Institutions must consider local factors and governance in defining levels of risk, which leads to some concerns. For example, many institutions classify research data and enterprise/administrative data in the same scheme, which makes it difficult to know how to apply risk levels to a given context — as in the University of British Columbia (2018), which classifies all electronic information in the same way with only a generic reference to research data. Other universities have guidelines that incorporate specific examples relevant to research, such as the University of Calgary (2015), which includes “identifiable human subject research” as an example of their highest risk level. Harvard University (2020) has a system dedicated to distinguishing levels of risk and harm for research data, including “data that would put the subject’s life at risk” in their highest category, which is defined as “sensitive data that could place the subject at severe risk of harm or data with contractual requirements for exceptional security measures.”
Libraries provide the tools, information, and education so researchers can preserve and share their data ethically and responsibly. But the researcher or principal investigator (PI) is responsible for conducting due diligence related to risks.
Reflective Questions
- In Canada, what is the primary ethical policy related to human participant research data?
- List three direct identifiers and three quasi-identifiers of human participant data.
- A graduate student is conducting fieldwork on an endangered turtle species along the St. Lawrence River in Québec. In a spreadsheet stored locally on their computer, they track turtles and record the following information about their sightings: latitude and longitude, proximity to the nearest industrial site, and number of turtles present. To what extent is this researcher working with sensitive data?
View Solutions for answers.
Key Takeaways
- De-identification is the process of removing from a dataset any information that might put research subjects’ privacy at risk.
- Sensitive data are data that cannot be shared without potentially violating the trust of or risking harm to an individual, entity, or community.
- Identifying information is any information in a dataset that, separately or in combination, could lead to disclosing the identity of an individual.
- Statistical Disclosure Risk Assessment is the process of mathematically assessing quasi-identifiers in a dataset to demonstrate that the data have been anonymized.
- In rating the risk level of a dataset, always consider the following: details within the dataset that have the potential, individually or in combination, to re-identify individuals; information external to the dataset that could be matched to data in the dataset or that reveals additional information about the study population; the level of harm that releasing the data could cause to individuals or communities.
- The most important privacy regulations for research data are located at the provincial/territorial level, as universities fall outside the scope of the main federal privacy laws. The Privacy Act applies to government organizations and the Personal Information Protection and Electronic Documents Act (PIPEDA) applies to private sector commercial entities. Researchers working with these organizations or using data collected by them (e.g., health records) need to be aware of these pieces of legislation. Provinces and territories in Canada have at least one privacy-related law that could be applied to research, so familiarize yourself with the law where you live. At the national level, the Tri-Council (also the agencies or Tri-Agency) policy statement on the ethical conduct for research involving humans (TCPS 2) is the most important framework governing research conduct.
Additional Readings and Resources
Alder, S. (2023, May 16). What is Considered PHI Under HIPAA? The HIPAA Journal. https://www.hipaajournal.com/what-is-considered-phi-under-hipaa/
Government of Canada. (2023). Tri-Council Policy Statement: Ethical Conduct for Research Involving Humans – TCPS 2 (2022). https://ethics.gc.ca/eng/policy-politique_tcps2-eptc2_2022.html
Henne, B., Koch, M., Smith, M. (2014). On the awareness, control and privacy of shared photo metadata. In N. Christin & R. Safavi-Naini. (Eds.), Financial Cryptography and Data Security. FC 2014. Lecture Notes in Computer Science (pp. 77-88). Springer. https://doi.org/10.1007/978-3-662-45472-5_6
Krafmiller, E. & Prasad, R. (2021, June 16). Sharing sensitive data [Conference presentation]. Dataverse Community Meeting 2021, virtual. In Sharing sensitive data. https://www.youtube.com/watch?v=q3irpQ4rOyU,
Portage Network, COVID-19 Working Group. (2020) De-identification guidance. https://doi.org/10.5281/zenodo.4270551
Ross, M. W., Iguchi, M. Y., & Panicker, S. (2018). Ethical aspects of data sharing and research participant protections. American Psychologist, 73(2), 138-145. http://dx.doi.org/10.1037/amp0000240
Sweeney, L. (2000). Simple demographics often identify people uniquely. Carnegie Mellon University, Data Privacy Working Paper 3, Pittsburgh 2000. http://ggs685.pbworks.com/w/file/fetch/94376315/Latanya.pdf
Thorogood, A. (2018). Canada: will privacy rules continue to favour open science? Human Genetics, 137(8), 595–602. https://doi.org/10.1007/s00439-018-1905-0
Reference List
Domingo-Ferrer, J. and Torra, V. (2008). A critique of k-anonymity and some of its enhancements. Third International Conference on Availability, Reliability and Security, ARES, IEEE, 990-993. https://doi.org/10.1109/ARES.2008.97
Finnish Social Science Data Archive. (2020). Anonymisation and personal data. https://www.fsd.tuni.fi/en/services/data-management-guidelines/anonymisation-and-identifiers/
First Nations Information Governance Centre. (n.d). OCAP® FAQs. https://fnigc.ca/ocap-training/
Government of Canada. (2017, January 11). What are controlled goods. https://www.tpsgc-pwgsc.gc.ca/pmc-cgp/quellessont-whatare-eng.html
Government of Canada. (2021, July 12). National security guidelines for research partnerships. https://science.gc.ca/eic/site/063.nsf/eng/h_98256.html
Han, Y., Li, S., Cao, Y., Ma, Q. & M. Yoshikawa. (2020) Voice-indistinguishability: Protecting voiceprint in privacy-preserving speech data release. 2020 IEEE International Conference on Multimedia and Expo (ICME), 1-6. https://doi.org/10.1109/ICME46284.2020.9102875
Harvard University. (2020, April 22). Data Security Levels – Research Data Examples. https://security.harvard.edu/data-security-levels-research-data-examples
Office of the Privacy Commissioner of Canada. (2018). Summary of privacy laws in Canada. https://www.priv.gc.ca/en/privacy-topics/privacy-laws-in-canada/02_05_d_15/
Office of the Privacy Commissioner of Canada. (2020, December 21). Questions and answers – Bill 64. https://www.priv.gc.ca/en/opc-news/news-and-announcements/2020/qa_20200924/
Qualitative Data Repository. (n.d.a). Templates for researchers. https://qdr.syr.edu/guidance/templates#informed%20consent
Qualitative Data Repository. (n.d.b). Informed consent. https://qdr.syr.edu/guidance/human-participants/informed-consent
Samarati, P. & Sweeney, L. (1998). Protecting privacy when disclosing information: k-anonymity and its enforcement through generalization and suppression. Harvard Data Privacy Lab. https://epic.org/wp-content/uploads/privacy/reidentification/Samarati_Sweeney_paper.pdf
Sensitive Data Expert Group of the Portage Network. (2020a). Sensitive Data Toolkit for Researchers Part 1: Glossary of Terms for Sensitive Data used for Research Purposes. https://doi.org/10.5281/zenodo.4088946
Sensitive Data Expert Group of the Portage Network. (2020b). Sensitive Data Toolkit for Researchers Part 3: Research Data Management Language for Informed Consent. https://doi.org/10.5281/zenodo.4107178
Thompson, K., & Sullivan, C. (2020). Mathematics, risk, and messy survey data. IASSIST Quarterly, 44(4), 1-13. https://doi.org/10.29173/iq979
University of British Columbia. (2018, June 27). Security classification of UBC electronic information. https://cio.ubc.ca/information-security-standards/U1
University of Calgary. (2015, January 1). Information Security Classification Standard. https://www.ucalgary.ca/legal-services/sites/default/files/teams/1/Standards-Legal-Information-Security-Classification-Standard.pdf
Wolford, B. (2018, November 5). Everything you need to know about the ‘Right to be forgotten’. GDPR.EU. https://gdpr.eu/right-to-be-forgotten/
Zhang, A., Fawaz, N., Ioannidis, S., & Montanari, A. (2012). Guess who rated this movie: identifying users through subspace clustering. Proceedings of the Twenty-Eighth Conference on Uncertainty in Artificial Intelligence, 944-953. https://dl.acm.org/doi/10.5555/3020652.3020750
data which cannot be shared without potentially violating the trust of or risking harm to an individual, entity, or community.
sources of information or evidence that have been compiled to serve as input to research.
the Natural Sciences and Engineering Research Council of Canada (NSERC), the Social Sciences and Humanities Research Council of Canada (SSHRC), and the Canadian Institutes of Health Research (CIHR) (the agencies) are Canada’s three federal research funding agencies and the source of a large share of the government money available to fund research in Canada.
Tri-Council Policy Statement: Ethical Conduct for Research Involving Humans. The primary harmonized framework that accounts for Canadian-wide laws and broader ethical paradigms applicable to the rights of human participants in research
an acronym for ownership, control, access, and possession. These four principles govern how First Nations data and information should be collected, protected, used, and shared. OCAP® was created because Western laws do not recognize the community rights of Indigenous Peoples to control their information.
“the data subject shall have the right to obtain from the controller the erasure of personal data concerning him or her without undue delay and the controller shall have the obligation to erase personal data without undue delay” (GDPR.EU, 2018).
information collected by the researcher that can uniquely identify human subjects, and include things like names, phone numbers, social insurance numbers, student numbers, and so on.
any information in a dataset that in combination could lead to disclosing the identity of an individual.
also known as quasi-identifiers, these are characteristics of people that do not uniquely identify individuals on their own but may, in combination, serve to reveal someone’s identity. A characteristic should only be considered quasi-identifying if an attacker could plausibly match that characteristic to information in an external source.
a Likert item is a question on a survey which asks respondents to select a response to indicate how much they agree or disagree with a statement. A Likert scale is developed by adding up or averaging a number of related Likert items.
a set of records in a dataset that has the same values on all quasi-identifiers.
an individual in a dataset whose information does not match any other individual in the dataset on the indirect identifiers.
a person in a population who may be identifiable because of some unique combination of demographic characteristics.
the process of removing from a dataset any information that might put research subjects’ privacy at risk.
a mathematical approach to demonstrating that a dataset has been anonymized.
records in a dataset that have the same values on a set of indirect identifier variables.
deleting individual cases or responses.
making changes to variables across datasets, such as grouping responses into categories.
a method of violating the confidentiality of a group of research subjects that can happen when everyone with a particular set of demographic characteristics also have a particular sensitive characteristic.
one of many privacy-protecting risk assessments based on k-anonymity but more restrictive.
one of many privacy-protecting risk assessments based on k-anonymity but more restrictive.
data generated by research examining social aspects of the human condition using descriptive methods rather than measurement.
a document that describes a dataset, including details about its contents and design.
a term that describes all the activities that researchers perform to structure, organize, and maintain research data before, during, and after the research process.
data about data; data that define and describe the characteristics of other data.
a formal description of what a researcher plans to do with their data from collection to eventual disposal or deletion.
data collected as a part of the process of administering something. Administrative data is used to track people, purchases, registrations, prices, etc.