A Canadian Context for Research Data Management
5 Research Data Sharing and Reuse in Canada: Practice and Policy
Meghan Goodchild; Shahira Khair; Amber Leahey; Kaitlin Newson; and Lee Wilson
Learning Outcomes
By the end of this chapter you should be able to:
- Understand practices, policies, and services guiding research data sharing and reuse in Canada.
- Identify elements of Canadian digital research infrastructure, including data storage options like data repositories and long-term preservation platforms, as well as services that support access and use of these infrastructures.
- Using case studies, identify supports and barriers to data sharing and reuse throughout the research data lifecycle, along with areas in need of future development.
Introduction
Canadian researchers from all disciplines and sectors are producing unprecedented amounts of data (Baker et al., 2019). With the advancement of open science and open data policies from journal publishers, research funders, disciplinary groups, and institutions, researchers are becoming more acutely aware of the need to manage their data in accordance with related policies on data deposit and sharing. These policies support broader goals around research transparency, reproducibility, and reuse (the Alliance Research Data Management Working Group [Alliance RDM WG], 2020). (See chapter 12, “Planning for Open Science Workflows,” for an overview of open science and open data.)
A major value proposition for data sharing and reuse is the acceleration of scientific progress and prevention of unnecessary expensive data collection. Data sharing also enables research results to be reproduced, which can improve the integrity and trustworthiness of published findings. When a researcher’s data is easy to discover and access, this increases the visibility and impact of their research. Additionally, sharing data, research environments, and tools enables and enhances collaboration, leading to greater interoperability and research efficiencies.
In order to maximize the benefits of data sharing and reuse, research data outputs should be guided by the FAIR principles of Findability, Accessibility, Interoperability, and Reusability, discussed in chapter 2, (Wilkinson et al., 2016), and supported by a foundation of a TRUST-ed (Transparency, Responsibility, User focus, Sustainability and Technology) digital research infrastructure and support services (Lin et al., 2020). Therefore, data sharing is an integral component of conducting high-quality research, requiring ongoing Research Data Management (RDM) practices. RDM services in Canada are emerging across disciplines, institutionally, and at the regional and national levels to support researchers in data sharing and reuse.
In this chapter, you’ll learn about policies and practices, digital research infrastructure, and tools and services for research data sharing and reuse in Canada. We’ll review policies and practices, Canadian infrastructure, tools, and services that support the research data lifecycle; and support services around data curation and preservation. Then we’ll consider case studies that highlight data sharing and reuse practices and highlight disciplinary challenges.
Policies and Practices in Canada
Research Funding Agencies
Funding agencies and governments around the world have recognized the need for national RDM policies to support access to publicly funded data. Funding agency mandates that require data sharing influence researcher behaviour and the demand for RDM infrastructure and services (the Alliance RDM WG, 2020). The Canadian Tri-Agency RDM Policy (2021) is driving a culture change for data deposit and sharing, as it outlines requirements for researchers to “deposit into a digital repository all research data, metadata and code that directly support the research conclusions in journal publications and pre-prints that arise from agency-supported research” (Government of Canada, 2021), implementation forthcoming. Grant recipients are expected to provide access to their data in accordance with the FAIR principles and disciplinary standards while respecting ethical, cultural, legal, and commercial requirements. Indigenous data sovereignty (discussed in depth in chapter 3) recognizes the inherent rights of Indigenous communities to govern the collection, ownership, and use of their data and may result in distinct practices regarding the sharing of research data.
Funder Policies
Local and Regional
Canadian research institutions may set their own requirements for data management and sharing, according to internal policies governing research practices and intellectual property. They must also publicly post a strategy indicating how RDM practices will be supported (Government of Canada, 2022).
National
- Tri-Agency RDM Policy (2021)
- Grant applicants must include a Data Management Plan for certain funding applications (phased implementation beginning in spring 2022)
- Grant recipients should deposit into a digital repository all research data, metadata, and code that directly support the research conclusions in journal publications and pre-prints that arise from agency-supported research. Deposit will be expected at the time of publication (implementation forthcoming).
- Although sharing data is not required, the Agencies expect researchers to provide appropriate access to the data where ethical, cultural, legal, and commercial requirements allow and in accordance with the FAIR principles and the standards of their disciplines. Whenever possible, these data, metadata, and code should be linked to the publication with a persistent identifier (PID).
- Tri-Agency Statement of Principles on Digital Data Management (2016)
- Data should be collected and stored using software and formats that ensure secure storage, preservation of, and access to the data beyond the duration of the research project.
- Tri-Agency Open Access Policy on Publications (2015)
- Researchers funded by the Canadian Institute of Health Research (CIHR) should deposit specific types of data (e.g., bioinformatics) into an appropriate public database.
- SSHRC Research Data Archiving Policy (1990)
- Research data must be preserved and made available for use within two years of project completion (Government of Canada, 2016).
International
Many public research funders in other countries that support Canadian research require researchers to share underlying datasets of published research, including:
- U.S. funders including National Institutes of Health (NIH) and National Science Foundation (NSF)
- UK Research and Innovation funders
- European Commission Horizon 2020
Several private research funding sources have their own data sharing expectations (e.g., Wellcome Trust, Bill & Melinda Gates Foundation).
Other Policies and Practices
Journal publishers have also been driving the adoption of RDM practices. When they require a data availability statement, research data is more likely to be shared online. When policies are less stringent, such as recommending data archiving, there’s only a slight increase in archiving rates over having no policy (Vines et al., 2013). Data sharing and availability differ by discipline. For example, the fields of biological science, earth science, medical science, and physical science have a higher rate of data sharing (Stuart et al., 2018), whereas data were less readily available in materials for energy and catalysis, psychology, optics and photonics, and forestry (Tedersoo et al., 2021).
Over the past 20 years, data sharing rates have improved (Tedersoo et al., 2021), but studies show that results are not always fully reproducible from shared data, often because of inadequate documentation and metadata (Rieseberg et al., 2021). There have been increasing efforts to mitigate this issue. For example, the Journal of Molecular Ecology encourages authors to use the open access database GEOME to establish permanent links between genetic data and geographic and ecological metadata to make the data deposited FAIR (Rieseberg et al., 2021). The Public Library of Science (2022) announced an “Accessible Data” pilot feature for certain articles to emphasize links to datasets in specific repositories, in order to increase sharing and discovery of research data and to highlight the benefits of open science models. The American Journal of Political Science, in partnership with the Odum Institute for Research in Social Science, provides data curation and verification services to ensure that datasets reproduce the results of corresponding articles (Jacoby et al., 2017). Therefore, policies alone are not sufficient but may require technical and discipline-specific solutions to ensure that the data shared can be accessible and reusable.
Infrastructure, Tools, and Services
A range of infrastructures are necessary to support the production, sharing, and reuse of data over its lifecycle. These work together to make data FAIR beyond the timespan of a research project.
There are three types of research data storage: active, repository, and archival. Figure 1 outlines active storage during the research phase, repository storage during the access and publishing phase, and archival storage during the preservation phase, which requires additional processing to support long-term accessibility.
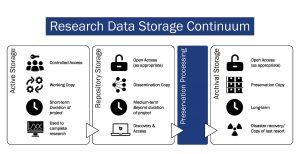
Table 1 details active, repository, and archival storage and provides examples used in Canada. Table 2 outlines different research infrastructures that facilitate sharing, reuse, and access.
Type | Attributes | Examples |
Active Storage
|
|
|
Repository Storage
|
|
|
Archival Storage
|
|
|
Type | Attributes | Examples |
Multi-Disciplinary Repositories
|
|
|
Disciplinary Repositories and Infrastructures
|
|
|
Preservation Services and Tools
|
|
|
Research Data and Software Replication Services and Tools
|
|
|
Data Discovery Services
|
|
|
Interoperability and Standards
|
|
|
Canadian Data Repositories
Data repositories are key to research infrastructure in Canada. National and institutional data repositories are emerging to support researchers with deposit, sharing, and long-term preservation of data to provide open, equitable, and connected RDM services, circumventing expanding commercial interests and reducing reliance on customized solutions, such as research project websites that often require maintenance and resources for the long term. Through federal, provincial, and institutional funding, Canadian repositories are available to researchers at no additional cost and may have a longer lifespan than the research project. Table 3 outlines types of data repositories in Canada, many of which can be discovered through global registries such as the Registry of Research Data Repositories (re3data), FAIRSharing, and OpenDOAR.
Type | Attributes | Examples |
Multi-Disciplinary Repositories
|
|
|
Disciplinary Repositories
|
|
|
Government Repositories
|
|
|
Knowledge Bases
|
|
|
Academic Data Repositories
|
|
|
Support Services
To produce datasets with a high potential for reuse, researchers must use good curation practices as data are cleaned, documented, interrelated, stored, and shared. A range of services provide support for researchers in developing these RDM practices and are detailed in Table 4.
The 2021 Researcher Needs Assessment survey conducted by the Digital Research Alliance of Canada (the Alliance) found researchers have varying levels of access to and awareness of support for research workflows, at local, provincial, and national levels, with the greatest access at the local level (Pérez-Jvostov et al., 2021).
- Internal supports: The first point of support for many researchers is internal to their own research groups. For instance, many research groups employ data managers to support team members with data management and publication. Researchers usually discover and select tools and services based on peer reference (Pérez-Jvostov et al., 2021).
- Higher education institutions: These provide formal services and support through offices of research, academic libraries, and research computing services (Pérez-Jvostov et al., 2021). The Tri-Agency’s requirement for institutional RDM strategies will help to coalesce cross-campus support.
- Shared support models: These can improve efficiency while meeting the demands of researchers and increasing access and equity. They are often coordinated by regional or national consortia. Case Study 1 is an example of a national community of practice as a support network for institutional repository administrators.
- Disciplinary services and supports: These serve the needs of specific research communities and are often advanced nationally and internationally through research organizations and publishers. They are vital for the adoption of standard practices and tools in related disciplines since they are tailored to specific research workflows.
Category | Services |
Data Management Planning (DMP)
|
The Alliance supports the infrastructure and oversees the development of the DMP Assistant tool. Academic libraries and offices of research work together to support local researchers in developing DMPs in compliance with the Tri-Agency’s RDM Policy.
|
Data Discovery and Access
|
Academic libraries support data discovery and access through reference services and database licensing. Some of these services are shared among institutions (e.g., Odesi, Abacus Dataverse repository). National and provincial organizations enable access and use of population data for research. Due to the sensitive subject matter, support often requires entering into an agreement with the service provider (e.g., CRDCN and StatCan Data Centres, ICES, Population Data BC). The Alliance supports a national discovery service Lunaris to increase exposure to Canadian data repositories and datasets. Exploratory work supports access to common datasets on high-performance computing infrastructures (e.g., bioinformatics datasets).
|
Computing and Storage
|
Local research computing and IT departments may offer services to researchers to support data management computing and active storage infrastructure. Increasing support for data management on active storage is important for the Alliance and its national federation of partners, and researchers can receive support through the Alliance’s national help desk.
|
Data Curation and Publication
|
A range of workflows and related guidance have been developed to assist curators, including:
To support open publishing, academic libraries provide curation support to researchers depositing data and other scholarly objects to institutional repositories or other digital asset management systems. Borealis enables local services through a distributed support model, where infrastructure is centrally hosted, but researchers receive support for curation from a local administrator (see Case Study 1 below). The Alliance provides curation support to researchers using the nationally accessible FRDR. They also support researchers in developing and deploying research gateways on advanced research computing infrastructure. Other repositories act as trusted resources for stewarding research data and provide services supporting their platforms (e.g., Canadian Astronomy Data Centre, Ocean Networks Canada, Polar Data Catalogue). Commercial publishers, including Springer Nature and Elsevier, offer services supporting dataset curation and publication. Others have partnerships with third-party repositories to support authors in publishing datasets underlying their publications (e.g., the partnership between Wiley and Dryad).
|
Training
|
Researchers receive training from services developed within their disciplinary communities and institutions (Pérez-Jvostov et al., 2021), often led by peer researchers and support specialists who act as “data stewards” who develop activities that advance awareness, understanding, development, and adoption of RDM tools, best practices, and resources. Key events in Canada include:
|
Emerging Service Areas
|
Services supporting data sharing and reuse in Canada are developing in response to the needs of researchers related to RDM. Emerging service areas include support for the following:
|
Case Study 1: Developing a National Dataverse Repository Service and Community in Canada
Background
The Dataverse Project is open-source research data repository software that allows users to share, cite, explore, and analyze research data. It is developed at the Institute for Quantitative Social Science at Harvard University, with collaborators from all over the world. Borealis, the Canadian Dataverse Repository, is based on Dataverse software and began as a regional research data repository for the Ontario Council of University Libraries, growing over the past ten years into a national, bilingual service with over 60 institutions subscribing. The infrastructure is hosted at the University of Toronto, with data files stored securely on the Ontario Library Research Cloud. Borealis offers one repository option for researchers who may not have a disciplinary repository available to them and who may benefit from the flexible data sharing features (e.g., open to restricted), exploration tools in the browser, and preservation-friendly actions and storage.
Analysis
Although Borealis is centrally hosted, academic libraries and institutions manage their collections, thereby supporting local researchers in depositing and sharing datasets. Since local capacity varies across institutions and regions (Goddard et al., 2018), cultivating a community of practice is crucial for capacity building across institutions and for collaborative development of resources and training materials to support researchers. Alongside efforts to develop technical infrastructure, the Borealis team has been collaborating with the Alliance’s Dataverse North Expert Groupon community-building initiatives, including creating bilingual resources, developing outreach and training materials for admins and users, hosting monthly community meetings, and maintaining an email list to openly share knowledge, expertise, and researcher needs (Goodchild & Huck, 2022).
Discussion
Creating spaces and supports for the community to flourish is essential for the viability of Borealis. Feedback provides insight to set priorities for technical and service developments, and community involvement in the development of user guides, admin guides, and other projects ensures that resources meet the needs of the research community. The overall goal of the community — to encourage successful sharing and reuse of research data — aligns with national efforts to strengthen digital research infrastructure and the RDM community in Canada (the Alliance RDM WG, 2020).
Considerations for Data Sharing
Data sharing requires planning. At the project outset, as part of a Data Management Plan, researchers must consider software and tools needed to collect, analyze, and document data; appropriate storage and backup procedures; how data will be deposited and (if possible) shared; and how they will manage data to ensure ethical and legal requirements are met.
Disciplinary differences, including attitude and culture, can influence data sharing and reuse. Certain research fields have traditions of data sharing and reuse and have adopted standards and tools to support this work. Especially within the humanities, where outputs do not always fit within traditional definitions for research data, researchers may consider different approaches to encourage sharing. Services and tools are often developed with disciplinary needs in mind and may be difficult to adopt or repurpose for other disciplines or contexts. Although general tools and services can help, they often lack disciplinary context that would make reuse and adoption possible. Other disciplinary considerations include the following:
- file formats (open vs. proprietary, standard tools and software within the discipline)
- metadata standards for documentation and dataset discovery
- active data storage, data transfer tools, and repository storage to support disciplinary needs (e.g., big data, sensitive data)
- repository selection based on features and user community
- availability of data curation support:
- data quality review
- data documentation for reuse
- data transformation (e.g., cleanup, anonymization, de-identification)
- terms of access and licensing for reuse
- data exploration and visualization tools
- the benefits of sharing different types of data
The following case studies examine research projects or disciplinary considerations in the fields of digital humanities (Case Study 2), health sciences (Case Study 3), and natural sciences (Case Study 4), highlighting issues faced by researchers and exploring solutions and lessons learned.
Case Study 2: Digital Humanities
Background
Queen’s University Library hosts the Diniacopoulos Collection Virtual Exhibit, the culmination of a research project that presents virtual reality movies and scaled 3D models of Greek and Egyptian archaeological artifacts from the Classics Department collection. The virtual exhibit is built in WordPress using Object2VR software to create an interactive experience for users to rotate and examine objects in virtual 3D in the browser.
Analysis
Researchers wanted to share and preserve the data from this project for future use as the field of virtual reality continues to grow. Web-based viewers and content management systems require ongoing maintenance of software and tools with an unknown lifespan, revealing considerations around sustainability and long-term access. Challenges were encountered in selecting a repository, given the size of the dataset (60 GB), the large number of files (6500+), and the complex folder structure, and that there are few options and best practices for this field. Additionally, including documentation and disciplinary metadata was crucial to ensure that the data could be reused and understood outside of the original context.
Discussion
The research team deposited the dataset into the Queen’s Dataverse Collection (Jones, Bevan, & Monette, 2017), part of Borealis, to benefit from the support of the Queen’s University Library, as well as features such as comprehensive metadata fields and the ability to assign a Digital Object Identifier (DOI) that could be linked from the virtual exhibit. The Borealis team supported the deposit of large zip archive folders for each artefact. Debate continues around the understanding of research data in the humanities, and further investigation is needed through dataset metrics and citations to determine whether there are challenges around the reuse of this contextual data and whether improved tools and platforms would better manage, share, and preserve these types of digital humanities projects.
Case Study 3: Sharing Sensitive Data
Background
Sensitive data refers to any data that may cause harm if made public. Typically, this refers to data collected about human subjects and can include sensitive, confidential, or personal information related to an individual’s health, ethnicity, political views, and/or geographic location, to name a few. Research data involving human subjects must be managed in accordance with Research Ethics Board (REB) guidelines and approval. Many institutions provide security standards and protection guidelines for managing sensitive and confidential data.
In Canada, research funded by the three federal research funding agencies (the agencies) involving human subjects is guided by the Tri-Council Policy Statement (TCPS 2): Ethical Conduct for Research Involving Humans (GoC Panel, 2023). Researchers must adhere to the policy, which covers issues related to consent, privacy, fairness, and equity, in relation to different types of human research, including clinical trials, genetic research, and research involving First Nations, Inuit and Métis. Research involving Indigenous peoples may not be subject to TCPS 2 guidelines depending on circumstances and terms agreed to or governing the research data that is considered under the control of the participants or community groups (see chapter 3, “Indigenous Data Sovereignty”; review the OCAP® principles for a model dealing with data on First Nations). The handling and use of sensitive data may be governed by other legal and ethical frameworks of the research program (e.g., CIHR, SSHRC) or institution, or at the provincial (e.g., FIPPA, PHIDA) or federal (e.g., Privacy Act, PIPEDA) level.
In 2021, the Tri-Council provided guidelines for researchers titled Guidance on Depositing Existing Data in Public Repositories (Government of Canada Panel on Research Ethics, 2021), which state that researchers may deposit and share data in a repository if they have received consent from participants to do so and/or if they receive approval from an REB. Researchers must be in compliance with the TCPS 2 before deposit and sharing and must seek REB approval before collection or reuse of human subject research.
Analysis
Infrastructure and support services for sensitive data storage, deposit, and sharing continues to be a major gap in Canada. The complexity around sensitive data requires intersection with a number of units on campus, including REB guidelines, contracts and legal support, RDM practices, and infrastructure and workflows to manage sensitive data throughout the lifecycle.
For health sciences research, there are several avenues to publish or share sensitive data with various considerations. De-identification or anonymizing the dataset involves removing identifiable data from a dataset. However, some datasets cannot be de-identified without compromising the usefulness of the data. Data may be shared through closed-access portals with data sharing/transfer agreements. One potential downside of this arrangement is the administrative overhead and potential need for a custom portal.
Discussion/Conclusions
There are ongoing efforts to improve tools, infrastructure, workflows, and resources around sensitive data management and sharing. Software, such as Research Electronic Data Capture (REDCap), has grown in popularity as a tool to capture data for clinical research and create databases and projects that are compliant with legal guidelines, secure, and easy to use (Patridge & Bardyn, 2018). The Alliance’s Sensitive Data Repository Project has led to the creation of a zero-knowledge encryption tool to facilitate secure deposit and controlled access to sensitive data within the FRDR platform. For the next phase of the project, the Alliance RDM team is leading the collaborative participation of institutions in policy framework development, which aims to clarify and streamline the workflow of sensitive data deposit and sharing. The Alliance’s Sensitive Data Expert Group has released resource documents to provide guidance around RDM practices in the context of research ethics frameworks, including the Sensitive Data Tool Kit:
- Part 1: Glossary of Terms for Sensitive Data used for Research Purposes
- Part 2: Human Participant Research Data Risk Matrix
- Part 3: Research Data Management Language for Informed Consent
Researchers need continued leadership for national solutions to ensure equitable access to support, tools, and infrastructure for sensitive data management and sharing.
Case Study 4: Supporting Canada’s Large Data Producers: SuperDARN and the Federated Research Data Repository
Background
The Super Dual Auroral Radar Network (SuperDARN) is a network of three dozen scientific radars deployed around the world by universities and government laboratories in ten countries. SuperDARN Canada (headquartered at the University of Saskatchewan) operates five radars in Canada, which produce valuable data that researchers can use to understand space weather, radio communication, and physics of the Earth’s upper atmosphere. However, due to the high-quality captures and rapid collection rates of the radars, SuperDARN is generating data at a massive scale, and storing this data in a manner that is secure, discoverable, and accessible is challenging. In 2018, SuperDARN Canada began meeting with the team at the FRDR.
Analysis
The size, scale, scope of the data, and complexity of SuperDARN’s organizational framework as an international research partnership presented numerous challenges. SuperDARN’s data collection began in 1993, and the data exist in both raw and processed forms. SuperDARN Canada and the FRDR team had to consider which format of the data (approximately 80 TB of raw data or approximately 10 TB of processed data for each algorithmic version) would be best to publish and, of the processed data, which algorithm generation to choose — the older algorithm that has widespread use or the newer one. Versioning datasets to update the outdated algorithm would mean doubling the size of the collection.
Data are collected across time, regions, and instruments via radar installations operating in the Northern and Southern Hemispheres, so the teams had to consider how to subdivide the data into publishable units that would be best suited for discovery, reuse, and usage tracking and reporting. They also had to consider the size of a dataset and number of files and take into account web browser limitations. And while the files are small, depending on how the data were organized, datasets had the potential to be many terabytes.
Since raw and processed data were available only as binary file types, the FRDR curation team could not perform quality checks on the files. The complexity of data also meant that without extensive documentation, the datasets would be useful only to a small number of users involved with the research.
Discussion/Conclusions
Format
The team decided to publish the raw form of the data dating back to 1993.
Curation
The FRDR curation team worked with SuperDARN Canada to review the datasets and develop README files that capture detailed descriptive and technical metadata required for reuse of the data by the broader researcher community. Links to associated publications and documentation were added, and datasets were linked to analysis and visualization software developed by SuperDARN.
Lessons Learned
In addition to the solutions discussed above, the following lessons were learned from this project:
- Consultation on data publication needs can take time and is an ongoing process. It took several years from the initial conversation to when the first datasets were ingested; and beyond publication, FRDR and SuperDARN Canada still meet periodically.
- Consistent communication is important, particularly when decisions require longer timeframes; setting regular meetings and documenting discussions and decisions ensures that everybody remains on the same page and that threads do not get lost.
- Sustainability and future planning are key. When working with SuperDARN, FRDR needed to think about the data publication needs associated with the collection and the commitment going forward.
Future of Data Sharing in Canada
There are a number of developments that could better support Canadian researchers with maximizing the benefits of data sharing. A few suggested areas are provided below, including improving access and inclusion, enhancing research platforms that support the lifecycle of data, developing tools and technologies to automate curation workflows, and improving integration and interoperability between systems and platforms.
Access and Inclusion
Systemic barriers to the inclusion of all researchers and disciplines in accessing and using data sharing tools and services must be removed to support more equitable adoption of data sharing policies and practices. New ways of thinking about data sharing are needed to transform infrastructures that support all types of research data; both in terms of formats and standards, but also the related conceptual models and workflows.
As data sharing workflows mature, attention must be paid to ensuring equitable publishing models are created. Given the high cost of storage, particularly for large datasets, we need to balance sustainability and equity.
Examples
- greater customization of data repositories and flexible tools and standards
- web accessibility standards within software and platforms
- open access agreements between research institutions, publishers, and repositories
Research Lifecycle Platforms
Typical repository workflows for uploading or downloading data require moving data across platforms and between storage locations. Transferring data in this manner is inefficient and costly, and it may be impossible or infeasible for large datasets due to cost, transfer times, or infrastructure limitations. Additionally, certain datasets rely on specialized software or computational environments for analysis. Research platforms and underlying storage clusters that accommodate the full lifecycle of data are needed, where data can be analyzed and curated and an authoritative version shared.
Examples
- easy-to-use tools for deploying datasets between different storage layers (e.g., moving data to and from repository and active storage)
- all-in-one cloud platforms for data analysis, curation, and sharing
Curation Automation
Making data available is not enough to advance open science, which requires significant resources of time and money to make datasets FAIR. New tools and technologies could reduce this investment and support researchers and curators in producing high-quality outputs.
Examples
- artificial intelligence algorithms that generate high-quality metadata from data
- software for automated data linkage within and across datasets
- software that guides researchers in documenting their datasets, with built-in standards and taxonomies
- software that checks for reproducibility and dataset quality
Integration and Interoperability
As demonstrated by the range of policies, tools, and services supporting the sharing of research data, there is significant momentum to progress these infrastructures. However, many are provided and developed in relative isolation, with only a few pieces of middleware or policy frameworks to connect them. As these infrastructures are developed, interoperability (e.g., connecting policy to platform, platform to service, service to policy, etc.) and integration into the research and publishing workflows will be a central focus to improve ease of use and increase adoption of data sharing practices.
Examples
- policy frameworks for sharing data across jurisdictional boundaries
- Incorporating Data Management Plans into research and sharing infrastructure
- connecting datasets into a broader network of research outputs
Conclusion
Canadian infrastructure, tools, and services that support sharing research data are important, particularly given policies requiring access to publicly funded data. A researcher’s area of study and ethical considerations impact the way data is shared and influence developments of policy and infrastructure that could advance data sharing in Canada.
Reflective Questions
- What are the challenges of sharing research data?
- What are the types of data storage? Provide an example of each.
- What are considerations around data sharing? How do disciplinary differences play a role in sharing?
- What kinds of data services (local, domain-specific, or national) could be developed to address the challenges and barriers identified in this chapter?
Key Takeaways
- Funders and publishers may set requirements that promote research data sharing; however, policies alone are not sufficient to ensure results are reproducible. Technical and discipline-specific solutions may be required to ensure that data is accessible and reusable.
- Storage options, infrastructures, and data repositories in Canada support the production, sharing, and reuse of research data over its lifecycle. Research data storage can be broken down into three types: active, repository, and archival. Canadian research institutions often provide storage infrastructures to their researchers, though availability depends on institutional capacity.
- Support services exist for Canadian researchers developing RDM practices, publishing data, or planning for reuse of data, including their own research groups, higher-education institutions, and services unique to the needs of specific research communities.
- Researchers should consider disciplinary differences and context around data sharing. Certain fields have a tradition of open data sharing and reuse. While some disciplines have adopted standards and tools to support this work, others may need support and tools to address areas such as metadata, file size, data type, and requirements around data sensitivities.
- Data sharing and reuse is supported through integration and interoperability between systems and platforms, including platforms that support the lifecycle and technologies that facilitate curation workflows.
Additional Readings and Resources
Barsky, E., Laliberté, L. W., Leahey, A., and Trimble, L. (2017). Chapter 3. Collaborative Research Data Curation Services: A View from Canada. In L. R. Johnston, Curating research data, volume one: Practical strategies for your digital repository (79-101). Association of College and Research Libraries. https://dx.doi.org/10.14288/1.0340778
Cheung, M., Cooper, A., Dearborn, D., Hill, E., Johnson, E., Mitchell, M., & Thompson, K. (2022). Practices before policy: Research data management behaviours in Canada. Partnership: The Canadian Journal of Library and Information Practice and Research, 17(1), 1–80. https://doi.org/10.21083/partnership.v17i1.6779
The First Nations Information Governance Centre. (2014, May). Ownership, control, access and possession (OCAP™): The path to First Nations information governance. https://achh.ca/wp-content/uploads/2018/07/OCAP_FNIGC.pdf
Garnett, A., Leahey, A., Savard, D., Towell. B., & Wilson, L. (2017). Open metadata for research data discovery in Canada. Journal of Library Metadata, 17(3-4), 201-217. https://doi.org/10.1080/19386389.2018.1443698
Thompson, K., & Kellam, L. M. (Eds.). (2016). Introduction to databrarianship: The academic data librarian in theory and practice. In L. M Kellam & K. Thompson (Eds.), Databrarianship: The academic data librarian in theory and practice. Association of College and Research Libraries. https://scholar.uwindsor.ca/cgi/viewcontent.cgi?article=1047&context=leddylibrarypub
Rice, R., & Southall, J. (2016). The data librarian’s handbook. Facet Publishing.
Reference List
The Alliance Research Data Management Working Group. (2020). The current state of research data management in Canada. https://alliancecan.ca/sites/default/files/2022-03/rdm_current_state_report-1_1.pdf
Baker, D., Bourne-Tyson, D., Gerlitz, L., Haigh, S., Khair, S., Leggott, M., Moon, J., Ridsdale, C., Tourangeau, R., & Whitehead, M. (2019). Research data management in Canada: A backgrounder. Zenodo. https://doi.org/10.5281/zenodo.3574685
Corcho, O., Eriksson, M., Kurowski, K, Ojsteršek, M., Choirat, C. van de Sanden, M., & Coppens, F. (2021). EOSC interoperability framework: Report from the EOSC executive board working groups FAIR and architecture. Publications Office of the European Union. https://data.europa.eu/doi/10.2777/620649
Goddard, L., Barsky, E., Cooper, A., Darnell, A., Davis, C., Doiron, J., & Taylor, S. (2018). Dataverse north working group: Year 1 recommendations. UBC Faculty Research and Publications. https://doi.org/10.14288/1.0386773
Goodchild, M., & Huck, J. (2022, March). Building a shared open research data repository community in Canada. Open Science Framework. osf.io/n8wzt
Government of Canada. (2022). Published institutional research data management strategies. https://www.ic.gc.ca/eic/site/063.nsf/eng/h_98428.html
Government of Canada. (2021). Tri-Agency research data management policy. https://www.science.gc.ca/eic/site/063.nsf/eng/h_97610.html
Government of Canada. (2016). Research data archiving policy. Social Sciences and Humanities Research Council. https://www.sshrc-crsh.gc.ca/about-au_sujet/policies-politiques/statements-enonces/edata-donnees_electroniques-eng.aspx
Government of Canada Panel on Research Ethics. (2021, May 25). Guidance on depositing existing data in public repositories. https://ethics.gc.ca/eng/depositing_depots.html
Government of Canada Panel on Research Ethics. (2023, January 11). Tri-Council policy statement: Ethical conduct for research involving humans – TCPS 2 (2022). https://ethics.gc.ca/eng/policy-politique_tcps2-eptc2_2022.html
Jacoby, W. G., Lafferty-Hess, S., & Christian, T-M. (2017). Should journals be responsible for reproducibility? Inside Higher Ed Blog. https://www.insidehighered.com/blogs/rethinking-research/should-journals-be-responsible-reproducibility
Jones, K., Bevan, G., & Monette, M. (2017). The Diniacopoulos ceramics display, Department of Classics – 2016. https://doi.org/10.5683/SP/T7ZJAF, Borealis, V2, UNF:6:B4MvStefgmeu6++JjcdOQg== [fileUNF]
Lin, D., Crabtree, J., Dillo, I. et al. (2020) The TRUST Principles for digital repositories. Sci Data, 7, 144. https://doi.org/10.1038/s41597-020-0486-7
Patridge, E. F., & Bardyn, T. P. (2018). Research electronic data capture (REDCap). JMLA, 106(1), 142–144. https://doi.org/10.5195/jmla.2018.319
Pérez-Jvostov, F., Iron, K., Khair, S., Sahrakorpi, S., & Zhang, Q. (2021). Researcher needs assessment: Summary of what we heard. Digital Research Alliance of Canada. https://alliancecan.ca/sites/default/files/2022-03/needsassessment_alliance_20220126.pdf
Public Library of Science. (2022, March 29). PLOS launches new feature to promote data sharing and access. The Official PLOS Blog. https://theplosblog.plos.org/2022/03/plos-launches-new-feature-to-promote-data-sharing-and-access/
Rieseberg, L., Warschefsky, E., O’Boyle, B., Taberlet, P., Ortiz-Barrientos, D., Kane, N. C., & Sibbett, B. (2021). Editorial 2021. Molecular Ecology, 30(1), 1-25. https://doi.org/10.1111/mec.15759
Stuart, D., Baynes, G., Hrynaszkiewicz, I., Allin, K., Penny, D., Lucraft, M., & Astell, M. (2018). Whitepaper: Practical challenges for researchers in data sharing. figshare. https://doi.org/10.6084/m9.figshare.5975011
Tedersoo, L., Küngas, R., Oras, E., Köster, K., Eenmaa, H., Leijen, Ä., Pedaste, M., Raju, M., Astapova, A., Lukner, H., Kogermann, K., & Sepp, T. (2021). Data sharing practices and data availability upon request differ across scientific disciplines. Scientific data, 8, 192. https://doi.org/10.1038/s41597-021-00981-0
Vines, T. H., Andrew, R. L., Bock, D. G., Franklin, M. T., Gilbert, K. J., Kane, N. C., Moore, J-S., Moyers, B. T., Renaut, S., Rennison, D. J., Veen, T., & Yeaman, S. (2013). Mandated data archiving greatly improves access to research data. The FASEB Journal, 27(4), 1304-1308. https://doi.org/10.1096/fj.12-218164
Wilkinson, M. D., Dumontier, M., Aalbersberg, I. J., Appleton, G., Axton, M., Baak, A., Blomberg, N., Boiten, J-W., da Silva Santos, L. B., Bourne, P. E., Bouwman, J., Brookes, A. J., Clark, T., Crosas, M., Dillo, I., Dumon, O., Edmunds, S., Evelo, C. T., Finkers, R., Gonzalez-Beltran, A., … Mons, B. (2016). The FAIR guiding principles for scientific data management and stewardship. Scientific data, 3, 160018. https://doi.org/10.1038/sdata.2016.18
the ability of data or tools from non-cooperating resources to work with or communicate with each other with minimal effort using a common language.
guiding principles to ensure that machines and humans can easily discover, access, interoperate, and properly reuse information. They ensure that information is findable, accessible, interoperable, and reusable.
a term that describes all the activities that researchers perform to structure, organize, and maintain research data before, during, and after the research process.
the cycle in which data is collected, processed, analyzed, preserved, and then shared so other researchers can start the cycle anew.
preliminary version of an article that has not undergone a formal peer-review process, but may be shared for comment. Pre-prints may be considered as grey literature.
a formal description of what a researcher plans to do with their data from collection to eventual disposal or deletion.
sources of information or evidence that have been compiled to serve as input to research.
data about data; data that define and describe the characteristics of other data.
a long-lasting reference to a digital object that gives information about that object regardless of what happens to it. Developed to address “link rot,” a persistent identifier can be resolved to provide an appropriate representation of an object whether that objects changes its online location or goes offline (CODATA, CC BY 4.0).
the free, immediate, online availability of information coupled with the rights to use this information fully in the digital environment.
a storage tier that supports data during the active phase of a research project, while data are being created, modified, or accessed frequently.
a storage tier that supports deposit, storage, discovery, and appropriate access to authoritative copies of digital materials in a variety of formats.
a storage tier that supports the series of managed activities needed to support long-term preservation of digital materials.
can be linked to the definition already in the glossary for fixity.
process of converting copies of original files to one of a small number of non-proprietary, widely-used, and preservation-friendly formats during ingest. Normalization standardizes ingested material into a subset of formats stored by an archives, and allows the archives to avoid managing a large number of formats into the future. However, normalization can also alter file sizes and properties. Archives should assess normalization priorities and approaches through researching and defining file format policies (Scholars Portal, n.d.).
the process of grouping data and information about data into a logical whole for use in a digital preservation process.
while their role can vary, data stewards in a research context are individuals tasked with ensuring data are handled systematically and uniformly.
the series of managed activities necessary to ensure continued access to digital materials for as long as necessary.
an academic field concerned with the application of computational tools and methods to traditional humanities disciplines such as literature, history, and philosophy.
a name (not a location) for an entity on digital networks. A DOI provides a system for persistent and actionable identification and interoperable exchange of managed information on digital networks. A DOI is a type of Persistent Identifier (PID) issued by the International DOI Foundation. This permanent identifier is associated with a digital object that permits it to be referenced reliably even if its location and metadata undergo change over time (CODATA Research Data Management Terminology, CC BY 4.0).
the Natural Sciences and Engineering Research Council of Canada (NSERC), the Social Sciences and Humanities Research Council of Canada (SSHRC), and the Canadian Institutes of Health Research (CIHR) (the agencies) are Canada’s three federal research funding agencies and the source of a large share of the government money available to fund research in Canada.
Tri-Council Policy Statement: Ethical Conduct for Research Involving Humans. The primary harmonized framework that accounts for Canadian-wide laws and broader ethical paradigms applicable to the rights of human participants in research
also known as version control, this means keeping track of the changes that are made to a file, no matter how small. This is usually done using an automated Version Control System, such as GitHub. Many file storage services, such as Dropbox, OneDrive, and Google Drive, keep historic versions of a file every time it is saved. These versions can be accessed by browsing the file's history.
a plain text file that includes detailed information about datasets or code files. These files help users understand what is required to use and interpret the files, which means they are unique to each individual project. Cornell University has a detailed guide to writing README files that includes downloadable templates (Research Data Management Service Group, n.d.).
the process of connecting different, often disparate systems or tools into a cohesive infrastructure.