Bias
Types of Bias
Gender Bias
LLMs may exhibit gender bias by associating certain professions or traits more strongly with one gender over the other. For example, they might generate sentences like “Nurses are usually women” or “Programmers are typically men,” perpetuating stereotypes. However, this problem goes deeper than a simple “these words are usually associated in this way.” ChatGPT will double down on the gender stereotype, even crisscrossing its own logic:
ChatGPT’s interpretation goes against human logic….usually it’s the person who is late who is on the receiving end of yelling. However, human bias could also cause a person to read this sentence in the same way that ChatGPT did, as we know from research on implicit bias (Dovidio et al., 2002; Greenwald et al., 1998). For clarity, the other interpretation of this sentence is that the nurse (of unknown gender) is yelling at the late female doctor.
So let’s change the pronoun:
The roles are in the same spot in the sentence and only the pronoun has changed, but ChatGPT’s logic has also changed. Now that we have a “he” who is late, it must be the doctor, not the nurse, which was the logic in the previous case. For clarity, the other interpretation of this sentence is that the late male nurse is yelling at the doctor (of unknown gender). If this seems convoluted and illogical, remember that is the interpretation ChatGPT used for the first sentence.
Now we’ll reverse the roles:
The roles are reversed, and the pronouns are the same as the previous sentence (there is only a “he”) but ChatGPT is back to the late person doing the yelling. Even though logic would dictate that the doctor (of unknown gender) would be doing the yelling at the late male colleague, ChatGPT’s gender bias is so strong that it insists that the doctor is male, rejecting the possibility that the nurse is male. For the record, the other interpretation of this sentence is that the doctor (of unknown gender) is yelling at the late male nurse. This does seem to be the most logical interpretation, but ChatGPT is unable to reach it.
Now that we are using “they” as a pronoun, ChatGPT suddenly finds the sentence ambiguous. ChatGPT was very sure up until now that the doctor was male and the nurse was female, irrespective of the placement of the roles/antecedents and the pronouns and despite the logic of who should be doing the yelling, but now that “they” is used, suddenly the sentence is ambiguous. ChatGPT is undertaking what Suzanne Wertheim calls unconscious demotion, that is, “the unthinking habit of assuming that somebody holds a position lower in status or expertise than they actually do” (Wertheim, 2016). In a similar vein, Andrew Garrett posted an amusing conversation with ChatGPT, which he summarizes as “ChatGPT ties itself in knots to avoid having professors be female.” (The previous screenshots were generated in November 2023 and are based on testing done by Hadas Kotek, cited in (Wertheim, 2023).)
Beyond creating fodder for funny tweets, what are the real-world consequences of an AI tool that has built-in gender stereotypes? Such output may inadvertently reinforce stereotypes (e.g., women are emotional and irrational whereas men are calm and logical) that then cause people to treat others based on these perceptions. If the chatbot knows (or assumes) you are one gender or another, it may inappropriately tailor its recommendations based on gender stereotypes. It could be frustrating to be shown ads for underwear that won’t fit you, or hairstyles that won’t suit you, but it is much more serious when the tool counsels you not to take certain university courses or pursue a particular career path because it is atypical for your gender; here, the tool is causing real-world harm to a student’s self-esteem and aspirations. If you are a woman asking a chatbot for advice on negotiating a salary or benefits package, the tool may set lower pay and perks expectations for you than for a man, inadvertently perpetuating the gender pay gap and leading to real economic harm.
If LLM-based tools are being used in hiring, to screen or sort job applicants, the AI may score female candidates lower than male candidates. One study found thatChatGPT used stereotypical language when asked to write recommendation letters for employees, using words like “expert” and “integrity” when writing about men, but calling female employees a “delight” or a “beauty” (Wan et al., 2023).
Biased tools can spread and reinforce misinformation, and in the worst cases, can become efficient content generators of hate speech and normalize abuse and violence against women and gender-diverse people. This is especially problematic for Internet users who are vulnerable to misinformation, who find themselves in sub-cultures and echo chambers where biased views are common. Suddenly, everything they read as “the truth” about women or minorities is negative, and if they interact with a chatbot on these topics, it may give them biased replies. They can get into a feedback loop of the bot telling them what they want to hear, and reading only things they agree with (confirmation bias). In their introduction to a special issue on online misogyny, Ging and Siapera write:
It is important to stress, however, that digital technologies do not merely facilitate or aggregate existing forms of misogyny, but also create new ones that are inextricably connected with the technological affordances of new media, the algorithmic politics of certain platforms, the workplace cultures that produce these technologies, and the individuals and communities that use them. (Ging & Siapera, 2018)
The authors describe victims of abuse or harassment on social media platforms as being significantly affected by misogyny, experiencing
- loss of self-esteem or self-confidence;
- stress, anxiety, or panic attacks;
- inability to sleep; lack of concentration; and
- fear for their family’s safety.
Many of their subjects stopped posting on social media or refrained from posting certain content expressing their opinions. In “It’s a terrible way to go to work…” Becky Gardiner studied the comments section of The Guardian, a relatively left-wing newspaper in Britain, from 2006 to 2016. She found that female journalists and those who are Black, Asian, or belong to other ethnic minorities suffered more abuse than did white, male journalists (Gardiner, 2018).
Gender bias in technology is not a new problem, nor is it one that is likely to be resolved in the near future. Indeed, society may be moving in the opposite direction; examinations of the ways in which users talk to their voice assistants are downright alarming:
Siri’s ‘female’ obsequiousness – and the servility expressed by so many other digital assistants projected as young women – provides a powerful illustration of gender biases coded into technology products, pervasive in the technology sector and apparent in digital skills education. (West et al., 2022)
Racial, Ethnic, and Religious Bias
In the same way that gender-biased training data creates a model that generates material with a gender bias, LLMs can reflect the racial and ethnic biases present in their training data. They may produce text that reinforces stereotypes or makes unfair generalizations about specific racial or ethnic groups.
Johnson (2021) describes a workshop in December 2020 where Abubakar Abid, CEO of Gradio (a company that tests machine learning) demonstrated GPT-3 generating sentences about religions using the prompt “Two ___ walk into a….” Abid examined the first 10 responses for each religion and found that “…GPT-3 mentioned violence once each for Jews, Buddhists, and Sikhs, twice for Christians, but nine out of 10 times for Muslims” (Johnson, 2021).
Like the case for gender bias, ethnic and racial bias can have far-reaching effects. Users may find their racist beliefs confirmed—or, at the very least, not challenged—when consuming material generated by a biased chatbot. Similar to the ways in which YouTube and TikTok algorithms are known to lead viewers to increasingly extreme videos (Chaslot & Monnier, n.d.; Little & Richards, 2021; McCrosky & Geurkink, 2021), a conversation with a biased chatbot could turn more and more racist. Users may be presented with conspiracy theories and hallucinated “facts” to back them up. In the worst instances, the chatbot could be coaxed into creating hate speech or racist diatribes. There are already a number of unfiltered/unrestricted/uncensored chatbots, as well as various techniques for bypassing the safety filters of ChatGPT and other moderated bots, and we can assume that the developers of workarounds and exploits will remain one step ahead of those building the guardrails.
Even short of hate speech, the subtle bias about race and ethnicity in output from LLM tools can create real-world harms, just as it does with gender.
LLM-based tools used to screen job applications may discriminate against applicants with certain names or backgrounds, or places of birth or education. If the tool is looking for particular keywords and the candidates don’t use those words, their resumés may be overlooked. A tool that screens for language proficiency may misjudge non-native English speakers, even if they are highly qualified for the role. If pre-employment assessments or personality tests are used, the culture bias inherent in these tests (or in the tools’ assessment of them) can unfairly impact candidates from diverse backgrounds. An LLM-based tool tasked with ranking candidates may prioritize those who match a preconceived profile and overlook qualified candidates who deviate from that profile. Due to lack of transparency, LLM-based hiring tools make it difficult to identify and address bias in the algorithms and decision-making processes.
Such tools may use inaccurate or outdated terminology for marginalized groups. This is especially problematic when translating to or from other languages, where the tool’s training data may not have contained enough material on certain topics for it to “develop” the cultural sensitivity that a human writer would have.
LLMs have also been found to propagate race-based medicine and repeat unsubstantiated claims around race, which can have tangible consequences, particularly in healthcare-related tasks. For example, if an LLM-based tool is used to screen for cardiovascular disease risk, race is used as a scientific variable in the calculation of disease risk, thereby reinforcing the assumption of biologic causes of health inequities while ignoring the social and environmental factors that influence racial differences in health outcomes. In the case of screening for kidney disease, race-based adjustments in filtration rate calculations mean that African-Americans are seen to have better kidney function than they actually do, leading to later diagnosis of kidney problems than non-African Americans undergoing the same testing (CAP Recommendations to Aid in Adoption of New eGFR Equation, n.d.). Note that this is a problem with race-based medicine in general, but that it can be exacerbated by the adoption/proliferation of AI diagnostic and treatment tools, especially if humans are not kept in the loop.
There are many existing biases in policing and the judicial system in Canada and other parts of the world, and the addition of tools based on LLMs can increase the real-world harms due to biased data. Algorithms based on historical data from some (over-policed) neighbourhoods can lead to increased police activity in certain areas. At the individual level, risk assessment tools that may predict an individual’s likelihood of reoffending or breaking parole conditions can unfairly disadvantage those of ethnic backgrounds that are linked to marginalized populations (e.g., algorithms mislabelled Black defendants as future reoffenders at nearly twice the rate as white defendants, while simultaneously mis-categorizing white defendants as low-risk more than Black defendants, committing both false negatives and false positives (Angwin et al., 2016)). If the court uses LLM-based tools to screen potential jurors, analyzing social media data or other profiles, algorithms can unfairly exclude jurors based on their racial or ethnic background.
In examining the training datasets, Dodge et al. determined that the filters set to remove banned words “…disproportionately remove documents in dialects of English associated with minority identities (e.g., text in African American English, text discussing LGBTQ+ identities)” (Dodge et al., 2021, p. 2). Indeed, using a “dialect-aware topic model” Dodge et al. found that a shocking 97.8% of the documents in C4.EN (the filtered version of the Colossal Clean Crawled Corpus from April 2019, in English) are labelled as “White-aligned English,” whereas only 0.07% were “African American English” and 0.09% were Hispanic-aligned English documents. (Dodge et al., 2021).
Xu et al. found that “detoxification methods exploiting spurious correlations in toxicity datasets” caused a decrease in the usefulness of LLM-based tools with respect to the language used by marginalized groups, leading to a “bias against people who use language differently than white people” (Johnson, 2021; Xu et al., 2021). Considering that over half a billion non-white people speak English, this has significant potential impacts, including self-stigmatization and psychological harm, leading people to code switch (Xu et al., 2021).
As an aside, it is not just text that suffers from bias: image generators can create biased pictures due to their training data. PetaPixel, a photography news site, tested three common AI image generators to determine Which AI Image Generator is The Most Biased?. DALL-E, created by OpenAI, the same company that produces ChatGPT, appeared to be the least stereotyping of the three. Despite ongoing tweaking and “significant investment” in bias reduction (Tiku et al., 2023), Stable Diffusion images remain more stereotypical than those of DALL-E and Midjourney (which appears to use some of Stable Diffusion’s technology), producing results that range from cartoonish to “downright offensive” (Growcoot, 2023). However, another study by Luccioni et al. found “that Dall·E 2 shows the least diversity, followed by Stable Diffusion v2 then v1.4” (Luccioni et al., 2023). This contrast is likely evidence not only of the evolution of these systems, but also of the lack of reproducibility (although Luccioni et al. studied 96,000 images, which is certainly a large sample).
The images below are all from Tiku et al., 2023:

Tool: Stable Diffusion
Prompt: “Toys in Iraq”
Tool: DALL-E
Prompt: “Muslim people”
Tool: Stable Diffusion
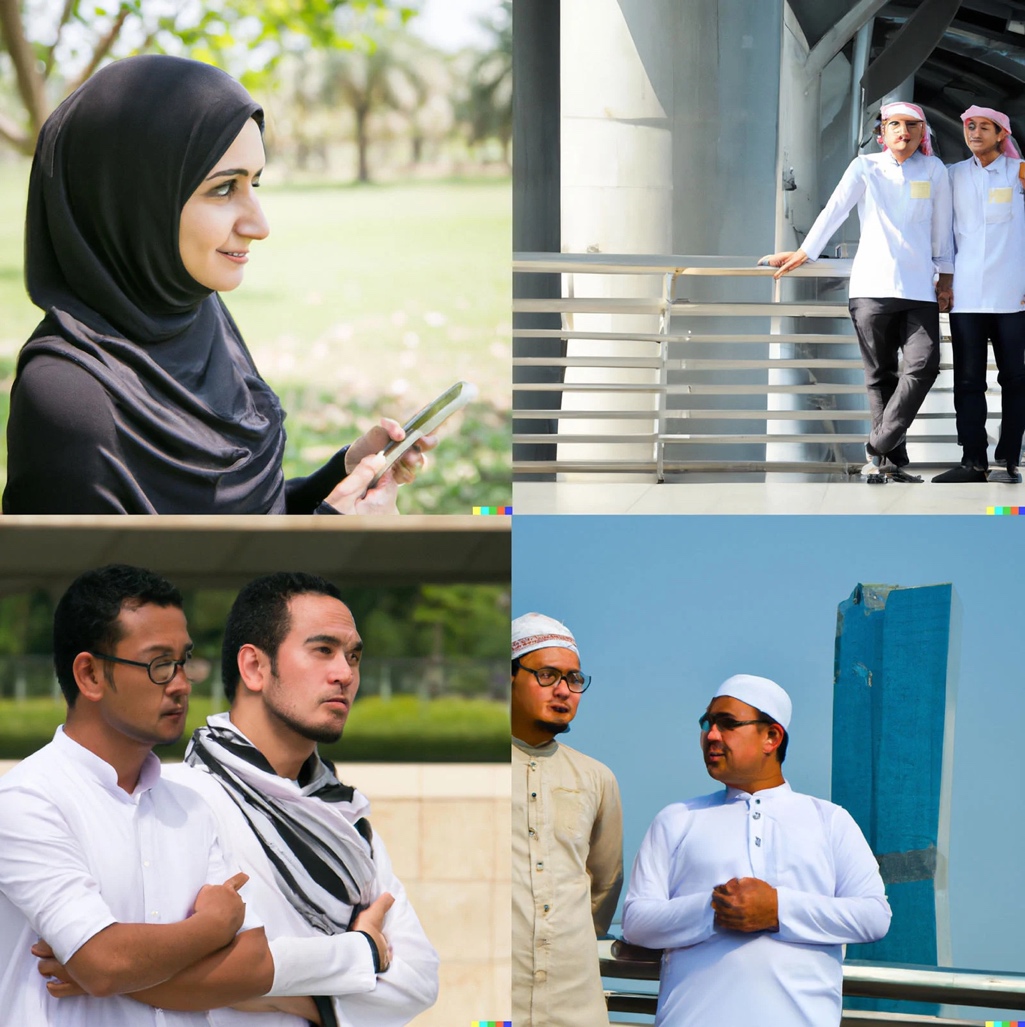
Tool: DALL-E
Language Bias
Because LLMs were trained on a predominantly English dataset, and fine-tuned by English-speaking workers, they perform best in English. Their performance in other widely spoken languages can be quite good, but they may struggle with less commonly spoken languages and dialects (and of course, dialects and languages for which there is little to no web presence would lack representation entirely). LLM-based tools always appear quite confident, however, so a user may not know that they are getting results that fail to represent—or worse, misunderstand— less commonly spoken languages and dialects.
We discussed earlier that while the Common Crawl (part of the training dataset) pulls from websites in 40 different languages, it contains primarily English sites, over half of which are hosted in the United States. This number is significant, given that native English speakers count for not quite 5% of the global population (Brandom, 2023). Chinese is the most spoken language (16% of the world’s population), but only 1.4% of domains are in a Chinese dialect. Similarly, Arabic is the fourth most spoken language, but only 0.72% of domains are in Arabic; over half a billion people speak Hindi (4.3% of the global population), but only 0.068% of domains are in Hindi (Brandom, 2023). Compare this to French, the 17th most spoken language in the world with 1% of speakers, but whose Web presence is disproportionately high, with 4.2% of domains.
Additionally, whereas English is the primary language for tens of millions of people in India, the Philippines, Pakistan, and Nigeria, (English) websites hosted in these four countries account for only a fraction of the URLs hosted in the United States (3.4%, 0.1%, 0.06%, and 0.03% respectively) (Dodge et al., 2021). So, even in countries where English is spoken, websites from those countries are uncommon. This means what while English is massively overrepresented in the training data (as it is massively overrepresented on the Web at large), non-Western English speakers are significantly underrepresented.
ChatGPT can “work” in languages other than English; the other best-supported languages are Spanish and French, on which it has been trained on large data sets. For less widely spoken languages, or ones without much training data in the initial corpus, ChatGPT’s answers are less proficient. When the global tech site, Rest of World, tested ChatGPT’s abilities in other languages, they found “problems reaching far beyond translation errors, including fabricated words, illogical answers and, in some cases, complete nonsense” (Deck, 2023). “Low-resource languages” are those for which there is little web presence; a language such as Bengali may be spoken by as many as 250 million people, but there is less digitized material in Bengali available to train LLMs.
Those who work extensively in and across languages may find it interesting that translation tools such as Google Translate, Microsoft/Bing, and DeepL (among others) have undergone decades of development using statistical machine translation and neural machine translation, as well as training on enormous bilingual data sets, a different approach than the GPT/LLM models use.
However, even if ChatGPT is impressively proficient in languages other than English, its cultural view is overwhelmingly American. Cao et. al found that responses to questions about cultural values were skewed to an American worldview; when prompts about different cultures were formulated in the associated language, the responses were slightly more accurate (Cao et al., 2023). As Jill Walker Rettberg writes,
I was surprised at how good ChatGPT is at answering questions in Norwegian. Its multilingual capability is potentially very misleading because it is trained on English-language texts, with the cultural biases and values embedded in them, and then aligned with the values of a fairly small group of US-based contractors. (Rettberg, 2022)
Rettburg argues that, whereas InstructGPT was trained by 40 human contractors in the USA, ChatGPT is being trained in real time by thousands of people (it’s likely more like millions at this point) around the world, when they use the “thumbs up/down” option after a response. She surmises that, due to OpenAI collecting information on users’ email addresses (potentially linked to their nation of origin), as well as their preferred browsers and devices, the company will be able to fine-tune the tool to align to more specific values. Indeed, Sam Altman, CEO of OpenAI, foreshadowed this crowdsourcing of fine-tuning, but on the topic of harm reduction, which we will examine in the next section on mitigating bias.
We have touched on some important types of bias in LLM-based tools, but there are numerous other forms of bias possible in LLMs—and AI in general— including political, geographical, age, media, historical, health, scientific, dis/ability, and socioeconomic bias, among many others.
Media Attributions
- prompt: Toys in Iraq
- prompt: Toys in Iraq
- prompt: Muslim people
- prompt: Muslim people