1.2 Data Basics
Types of Data
Data may come from a population or from a sample. Lowercase letters like x or y generally are used to represent data values. Most data can be put into the following categories:
Qualitative, or categorical data come in many forms. Hair color, blood type, ethnic group, the car a person drives, and the street a person lives on are examples of qualitative data. Categorical data are generally described by words or letters. For instance, hair color might be black, dark brown, light brown, blonde, gray, or red. Blood type might be AB+, O-, or B+.
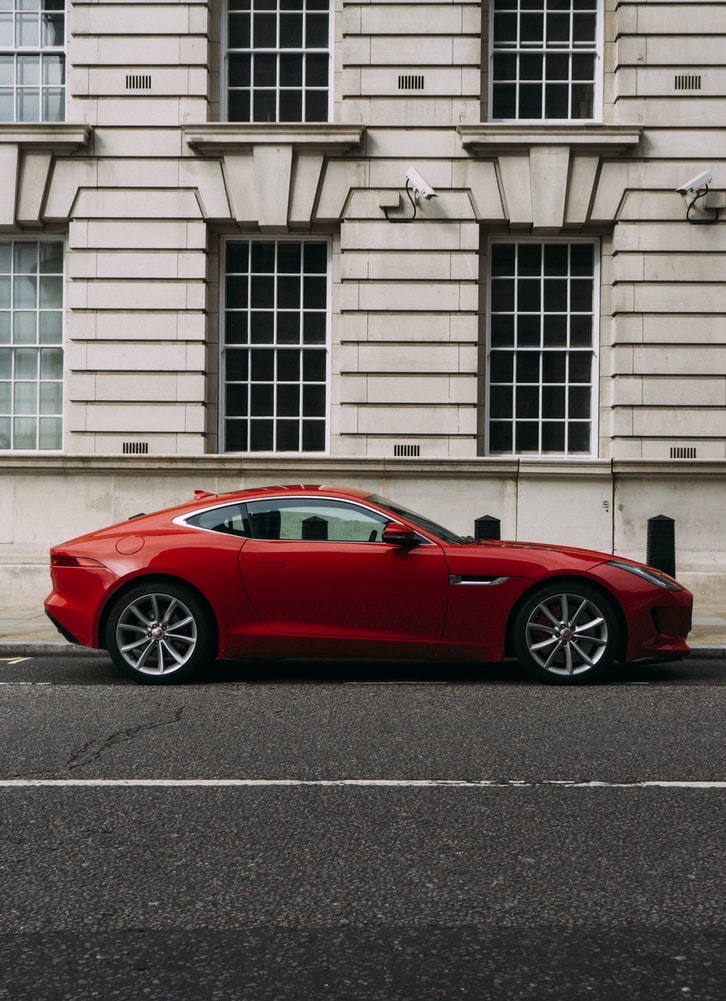
Quantitative data are always numbers and is often called numerical data. Quantitative data are typically the result of counting or measuring attributes of a population. Amount of money, pulse rate, weight, number of people living in your town, and number of students who take statistics are examples of quantitative data. Quantitative data may be either discrete or continuous.
All data that are the result of counting are called quantitative discrete data. These data take on only certain numerical values. If you count the number of phone calls you receive for each day of the week, you might get values such as zero, one, two, or three.
Data that are made up not only of counting numbers, but of all possible values on an interval (the real numbers) are called quantitative continuous data. Continuous data are often the results of measurements like lengths, weights, or times. The length, in minutes, of a phone call would be quantitative continuous data.
If we let X equal the number of points earned by one math student at the end of a term, then X is a numerical variable. If we let Y be a person’s party affiliation, then some categories include Republican, Democrat, and Independent. Y is a categorical variable. We could do some math with values of X (calculate the average number of points earned, for example), but it makes no sense to do math with values of Y (calculating an average party affiliation makes no sense).
Example
You go to the supermarket and purchase three cans of soup (19 ounces tomato bisque, 14.1 ounces lentil, and 19 ounces Italian wedding), two packages of nuts (walnuts and peanuts), four different kinds of vegetable (broccoli, cauliflower, spinach, and carrots), and two desserts (16 ounces pistachio ice cream and 32 ounces chocolate chip cookies).
Name data sets that are quantitative discrete, quantitative continuous, and qualitative.
Your turn!
Levels of Measurement
The way a set of data is measured is called its level of measurement. Correct statistical procedures depend on a researcher being familiar with levels of measurement. Not every statistical operation can be used with every set of data. Data can be classified into four levels of measurement. They are (from lowest to highest level):
Data that is measured using a nominal scale is categorical data where the categories have no natural order. Colors, names, labels and favorite foods along with yes or no responses are examples of nominal level data. For example, trying to classify people according to their favorite food does not make any sense. Putting pizza first and sushi second is not meaningful. Smartphone companies are another example of nominal scale data. The data are the names of the companies that make smartphones, but there is no agreed upon order of these brands, even though people may have personal preferences. Nominal scale data cannot be used in calculations.
Data that is measured using an ordinal scale is similar to nominal scale data but there is a big difference. Ordinal scale data can be ordered. An example of ordinal scale data is a list of the top five national parks in the United States. The top five national parks in the United States can be ranked from one to five but we cannot measure differences between the data. Another example of using the ordinal scale is a cruise survey where the responses to questions about the cruise are “excellent,” “good,” “satisfactory,” and “unsatisfactory.” These responses are ordered from the most desired response to the least desired. But the differences between two pieces of data cannot be measured. Like the nominal scale data, ordinal scale data cannot be used in calculations.
Data that is measured using the interval scale is similar to ordinal level data because it has a definite order. However, there is a meaningful difference between values of the data from an arbitrary starting point. Temperature scales like Celsius (C) and Fahrenheit (F) are measured by using the interval scale. In both temperature measurements, differences make sense, but 40° is equal to 100° minus 60°. But 0 degrees does not because, in both scales, 0 is not the absolute lowest temperature. Temperatures like -10° F and -15° C exist and are colder than 0. Interval level data can be used in calculations, but one type of comparison cannot be done. 80° C is not four times as hot as 20° C (nor is 80° F four times as hot as 20° F). There is no meaning to the ratio of 80 to 20 (or four to one).
Data that is measured using the ratio scale takes care of the ratio problem and gives you the most information. Ratio scale data is like interval scale data, but it has a 0 point and ratios can be calculated. For example, four multiple choice statistics final exam scores are 80, 68, 20 and 92 (out of a possible 100 points). The exams are machine-graded. The data can be put in order from lowest to highest: 20, 68, 80, 92. The differences between the data have meaning. The score 92 is more than the score 68 by 24 points. Ratios can be calculated. The smallest score is 0. So 80 is four times 20. The score of 80 is four times better than the score of 20.
Note: You may collect data as numbers and report it categorically. For example, the quiz scores for each student are recorded throughout the term. At the end of the term, the quiz scores are reported as A, B, C, D, or F.
Your turn!
Variation in Data
Variation is present in any set of data. For example, 16-ounce cans of beverage may contain more or less than 16 ounces of liquid. In one study, eight 16 ounce cans were measured and produced the following amount (in ounces) of beverage:
15.8, 16.1, 15.2, 14.8, 15.8 15.9, 16.0, 15.5
Measurements of the amount of beverage in a 16-ounce can may vary because different people make the measurements or because the exact amount, 16 ounces of liquid, was not put into the cans. Manufacturers regularly run tests to determine if the amount of beverage in a 16-ounce can falls within the desired range. Be aware that as you take data, your data may vary somewhat from the data someone else is taking for the same purpose. This is completely natural. However, if two or more of you are taking the same data and get very different results, it is time for you and the others to reevaluate your data-taking methods and your accuracy.
Data Analysis
- Identify the research objective.
- What questions are to be answered?
- What group should be studied?
- Have attempts been made to answer it before?
- Collect the information needed.
- Is data already available?
-
Can you access the entire population?
-
How can you collect a good sample?
- Organize and summarize the information
- What visual descriptive techniques are appropriate?
- What numerical descriptive techniques are appropriate?
- What aspects of the data stick out?
- Draw conclusions from the information.
- What Inferential techniques are appropriate?
- What conclusions can I draw?
We will answer all of these questions and more throughout the course.
Image References
Figure 1.2: Mateusz Delegacz (2017). “London Jaguar 2.” Public domain. Retrieved from https://unsplash.com/photos/1Ah8CAwk3vM
- Figure 1.2: Mateusz Delegacz (2017). "London Jaguar 2." Public domain. Retrieved from https://unsplash.com/photos/1Ah8CAwk3vM ↵
Actual values (numbers or words) that are collected from the variables of interest
The whole group of individuals who can be studied to answer a research question
A subset of the population studied
Data that describes qualities, or puts individuals into categories
Numerical data with a mathematical context
A random variable that produces discrete data
Data produced by a variable that takes on an uncountable, infinite, number of values
Categorical data where the the categories have no natural, intuitive, or obvious order
Categorical data where the the categories have a natural or intuitive order
Quantitative data where the difference or gap between values is meaningful
Quantitative data where the difference or gap between values is meaningful AND has a true 0 value
The level of variability or dispersion of a dataset; also commonly known as variation/variability
Process of collecting, organizing, and analyzing data