Section 2: Data Literacy Competencies
Dr. Sinéad McElhone; Sherri Hannell; and Noah James
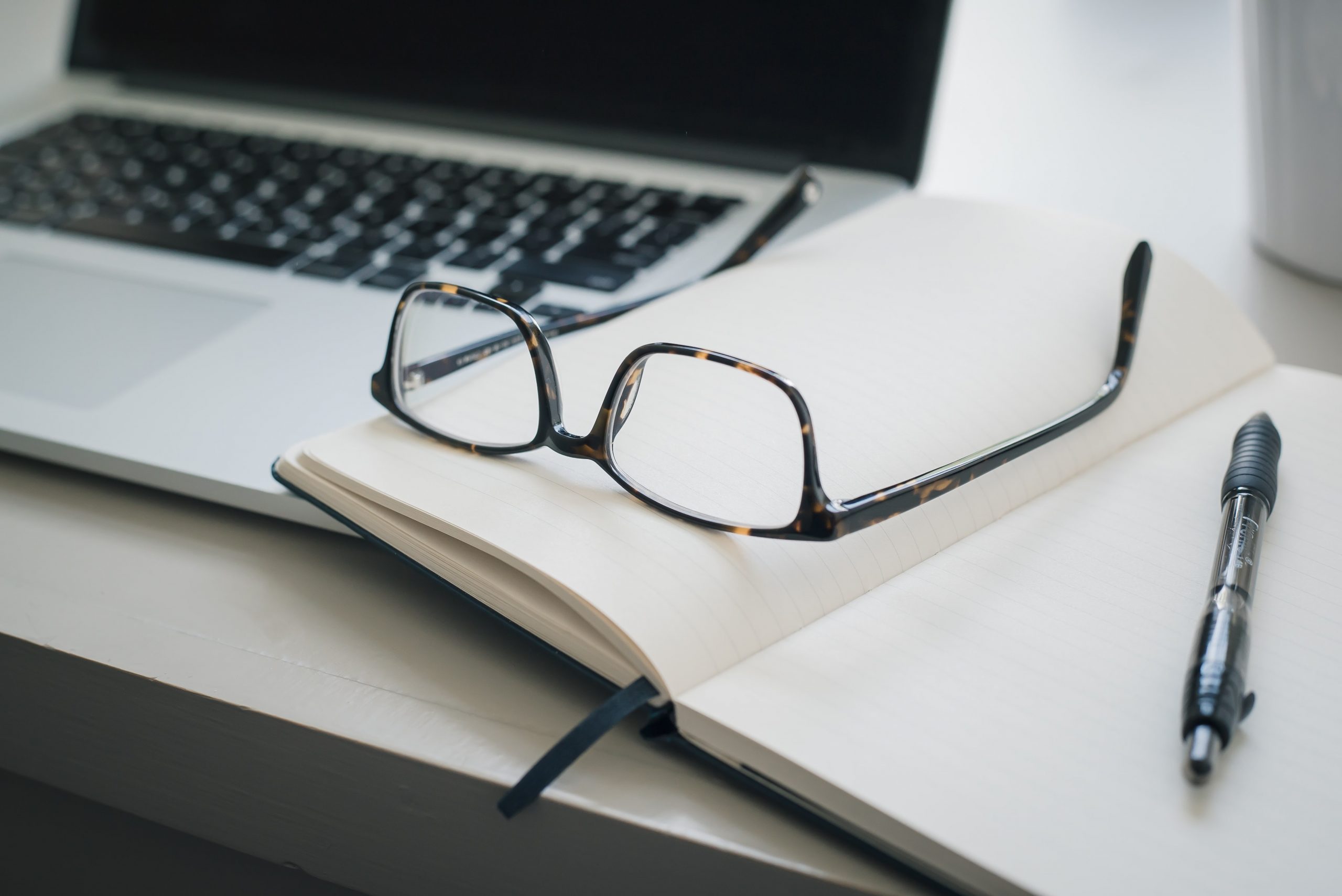
Section Overview
This section aims to provide an overview of data literacy competencies, which are the knowledge, skills, activities, and competencies required to successfully manage and work with data assets.
Section Objectives
By the end of this section, you will be able to:
- Provide an overview of what is meant by data literacy competency; and
- Describe several core data literacy competency skills and activities.
Test Your Knowledge
Complete the following activity to assess how much you already know about the content that will be covered in this section.
Introduction
We live in a data-driven world. Data is changing nearly every aspect of our lives, from business decisions to how we shop. Netflix is a great example of how the use of data (big data) gathered by the organization has helped it to predict trends in viewing among their audiences and has generated the company billions of dollars.
Deeper Dive
- This link contains more information on this topic: https://www.forbes.com/sites/enriquedans/2020/01/15/netflix-big-data-and-playing-a-long-game-is-proving-a-winningstrategy/?sh=593fc57b766e
Data literacy is an essential part of a data-driven culture. Data literacy competencies are the knowledge, skills, and activities you need to effectively work with data. These competencies include the knowledge and skills to read, analyze, interpret, visualize, and communicate data, as well as the ability to understand the use of data in decision-making, and to ensure informed decisions are made.
Establishing consistent practices for the management of data assets increases their value by ensuring that the information obtained is high quality, easily accessible, and effectively managed and governed.
The following activities, skills, and knowledge represent the basis of data literacy competencies. A further explanation of core data literacy competencies, as well as some key advanced data literacy skills and concepts, will be explored later in this section.
Data literacy competencies include:
- Data analysis
- Data awareness
- Data cleaning
- Data discovery
- Data ethics
- Data exploration
- Data gathering
- Data governance
- Data interpretation
- Data management and organization
- Data modelling
- Data stewardship
- Data tools
- Data visualization
- Evaluating data quality
- Evaluating decisions based on data
- Evidence-based decision-making
- Metadata creation and use
- Storytelling
Core Data Literacy Skills
Data Discovery
Data discovery refers to the knowledge and skills to search, identify, locate, and access data from a range of sources related to the needs of an organization (Statistics Canada, 2020).
A gatherer or organization begins by asking questions that can be answered using data to generate insights. The first step is to identify the opportunity or challenge that needs to be addressed or answered. In this step, an individual or member of an organization must identify the need for data and initiate a conversation around the specific data needed, when it is needed, who the audience for the data is for, and ultimately what story or insight will be delivered from the data. The individual or organization identifies the need, the question they are trying to answer, or the problem they are trying to solve.
Once the need has been identified, the correct data or datasets must be identified to fulfill the need. Data discovery is the process by which data is sought, identified, located, and accessed from a variety of sources, both internal or external to an organization, to best answer the question being asked.
Data Gathering
Data gathering refers to the knowledge and skills to gather data in simple and more complex forms to support the gatherer’s or organization’s needs (Statistics Canada, 2020). This could involve the planning, development, and execution of surveys or gathering data from other sources such as administrative data, satellite, or social media data.
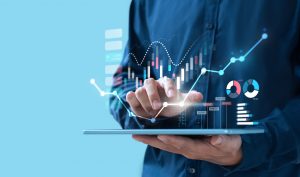
Data can be gathered, collected, or received from multiple sources and data providers, both internal and external to an organization. The gathering phase involves accumulation or transmission of data. Data can be gathered from surveys or other data sources or for organizations, from data providers or sources. Individuals gathering data may conduct data checks to ensure the integrity of data. For organizations, initial transmission and data quality checks are conducted, and alerts may be triggered if issues with the data are identified. These controls will reduce the risk of bad data being integrated into an organizations larger data ecosystem. If issues of data quality are identified and/or alerts are triggered, data quality issues need to be addressed or documented prior to the data being used.
All potential users of the data will also have access to metadata that helps describe the data in order to enable the discovery and understanding of the data available. Metadata is data about data, including the definitions and descriptions about the data, and makes finding and working with data easier. An example of metadata would be the date data was gathered. Users of the data can be notified about data availability and the data can be made available for use by authorized users.
Data Quality and Cleansing
Data quality refers to the knowledge and skills to assess data sources to ensure they meet the needs of the gatherer or organization (Statistics Canada, 2020). This includes both identifying errors and taking action to address the issues with the data.
Data cleansing refers to the knowledge and skills to determine if data is ‘clean’ and if not, using the best methods and tools to take necessary actions to resolve any problems (Statistics Canada, 2020).
As part of data collection, data quality checks are conducted to identify any issues with the data. Such controls reduce the risk of bad data being integrated or used. Data quality represents the degree to which data is accurate, valid, timely, usable, and consistent to make it fit for use. Data quality is measured along by its accuracy, validity, timeliness, usability, and consistency. Assessing, monitoring, and managing data quality issues upfront in the data collection process helps ensure that the data being provisioned is fit for its intended use.
Evaluating the quality of data and cleansing data are symbiotic. If the quality of data is determined to be poor, action is required to resolve issues and ensure its suitability for analysis. Data quality issues should be documented, allowing data consumers to understand and better use data, keeping in mind any known quality issues.
Click here to watch a video about data quality on the Statistics Canada website. In this video, you will be introduced to the fundamentals of data quality, which can be summed up in six different ways or dimensions to think about quality. You will also learn how each dimension can be used to evaluate the quality of data. Finally, you will learn about basic quality concepts, data quality expressed as six dimensions, and the interactions between these dimensions, which will help you gain a basic understanding of data quality.
Data Management and Organization
Data management and organization refer to the knowledge and skills required to navigate internal and external systems to locate, access, organize, protect, and store data related to the organization’s needs (Statistics Canada, 2020).
These are key enablers of data sharing and use, ensuring sources of data, and consistent use of data. The management and organization, or architecture of data, is a set of rules, policies, standards, and models that establish how data is organized, stored, managed, and integrated within an organization. It includes the development and maintenance of conceptual and logical data models and their entities and relationships. Efficient data organization or architecture management ensures that new data requirements and specifications are integrated and work with the existing organizational data architecture. Enterprise data organization and management supports data standardization and integration.
Data privacy and security management includes the planning, implementation, and control activities to ensure that data services provided comply with all regulatory and legislative requirements that an organization is subject to. Data privacy and security management helps ensure the privacy and confidentiality of data and prevents unauthorized and inappropriate data access, use, and storage. Apart from complying with current laws and regulations, data privacy and security management play a key role in enabling both gatherers of data and organizations to build the trust and confidence of their audience which may be partners, customers, and the public.
Data Exploration
Data exploration refers to the knowledge and skills required to use a range of methods and tools to explore patterns and relationships in the data (Statistics Canada, 2020). The methods include summary statistics, frequency tables, outlier detection, and visualization to explore patterns and relationships in the data.
Once the need has been identified and a methodology developed, data consumers or users of the data create a plan for their work and explore the data assets available for use. This stage includes the completion of the data requirements document. The requirements document captures the details of the work being undertaken, the story to be told, and the plan for fulfilling the deliverable. Data sources, concepts, and indicators should be captured in the plan, as well as the measurement strategy and methodology. The requirements document is used in the quality assurance process to understand how the work was done and assess its validity.
The data steward role involves all activities to govern, safeguard, and protect data and the knowledge and skills required to effectively manage data assets. Data stewards may be consulted at this stage about the appropriate use of data assets.
Data Interpretation
Data interpretation refers to the knowledge and skills required to read and understand tables, charts, and graphs and identify points of interest (Statistics Canada, 2020). Interpretation of data also involves synthesizing information from related sources.
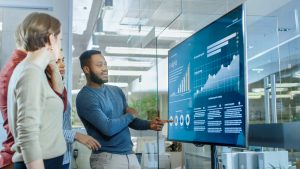
This is the process of reviewing data through some predefined processes, which will help assign some meaning to the data and arrive at a relevant conclusion. It involves taking the result of data analysis, making inferences on data relationships, and using them to make conclusions. Data interpretation and the process of conducting analysis to order, categorize, and summarize data is key to conducting analysis and using data to tell a story.
Data interpretation is the means to help make sense of the data that has been collected, analyzed, and presented. Data, when collected in raw form, may be difficult for the layman to understand, which is why analysts need to break down the information gathered so that others can make sense of it.
Decision-Making and Storytelling
Evidence-based decision-making refers to the knowledge and skills required to use data to help in the decision-making and policy-making process (Statistics Canada, 2020). This includes thinking critically when working with data, formulating appropriate business questions, identifying appropriate datasets, deciding on measurement priorities, prioritizing information garnered from data, converting data into actionable information, and weighing the merit and impact of possible solutions and decisions.
Storytelling refers to the knowledge and skills required to describe key points of interest in statistical information (i.e., data that has been analyzed) (Statistics Canada, 2020). This includes identifying the desired outcome of the presentation, identifying the audience’s needs and level of familiarity with the subject, establishing the context, and selecting effective visualizations.
In the evidence-based decision-making and storytelling phase, data used to draw conclusions and insights from the analyses has been completed and a summary of actionable ideas or answers is provided. Any meaningful features and trends are captured and aligned to the framework identified to solve the problem or answer the question that the organization has brought forward.
Information design is the practice of storytelling or presenting information in a way that fosters efficient and effective understanding of it. After the data has been interpreted, a review of the options for presenting information is undertaken and visualizations and graphics are designed that will enhance the understanding of the underlying data and tell a story to the intended audience.
Deeper Dive
- This Statistics Canada webpage contains more information on this topic: https://www.statcan.gc.ca/en/wtc/data-literacy
Summary
In summary, data literacy is the ability to understand and communicate data as information to inform decision-making. There are a wide variety of individuals with specific skill sets involved in this process, from the data engineer who builds pipelines of data from source systems to display on dashboards, to analysts who use a variety of different software to produce accurate and valid statistics, to knowledge translators who, through knowledge translation (see Chapter 4: Knowledge Translation and Exchange to Support Decision-Making), can understand, interpret these data, and translate these insights to leaders in order to underpin evidence-based decision-making. Historically, the concept of data literacy has not been taught consistently across higher education, however, it is now necessary to have data-literate graduates to support workplaces. Data literacy also takes into consideration the ownership of data from an Indigenous perspective via OCAP®. Data literacy is acknowledged as a crucial skill for the 21st-century workplace.
Test Your Knowledge
Complete the following activity to assess how much you learned about the content that was covered in this section.
The ability to understand and communicate data as information (Jackson & Carruthers, 2019).
A named collection of related data elements that is formally managed as a single unit. They may be a collection of facts represented as text, numbers, graphics, images, sound, or video, and are the raw material from which information can be derived and decisions can be made.
The knowledge and skills required to ask and answer a range of questions by analyzing data including developing an analytical plan, selecting and using appropriate statistical techniques and tools, and interpreting, evaluating, and comparing results with other findings (Statistics Canada, 2020).
The knowledge required to know what data is and what are different types of data (Statistics Canada, 2020). This includes understanding the use of data concepts and definitions.
The knowledge and skills to determine if data is 'clean' and if not, using the best methods and tools to take necessary actions to resolve any problems (Statistics Canada, 2020).
The knowledge and skills to search, identify, locate, and access data from a range of sources related to the needs of an organization (Statistics Canada, 2020).
The knowledge that allows a person to acquire, use, interpret and share data in an ethical manner including recognizing legal and ethical issues (e.g., biases, privacy) (Statistics Canada, 2020).
The knowledge and skills required to use a range of methods and tools to explore patterns and relationships in the data (Statistics Canada, 2020). The methods include summary statistics, frequency tables, outlier detection, and visualization to explore patterns and relationships in the data.
The knowledge and skills to gather data in simple and more complex forms to support the gatherer’s or organization's needs (Statistics Canada, 2020). This could involve the planning, development, and execution of surveys, or gathering data from other sources such as administrative data, satellite, or social media data.
A discipline which provides the necessary policies, processes, standards, roles, and responsibilities needed to ensure that data is managed as an asset (Fircan, 2021b).
The knowledge and skills required to read and understand tables, charts, and graphs and identify points of interest (Statistics Canada, 2020). Interpretation of data also involves synthesizing information from related sources.
The knowledge and skills required to navigate internal and external systems to locate, access, organize, protect, and store data related to the organization's needs (Statistics Canada, 2020).
The knowledge and skills required to apply advanced statistical and analytic techniques and tools (e.g., regression, machine learning, data mining) to perform data exploration and build accurate, valid and efficient modelling solutions that can be used to find relationships between data and make predictions about data (Statistics Canada, 2020).
The knowledge and skills required to effectively manage data assets (Statistics Canada, 2020). This includes the oversight of data to ensure fitness for use, the accessibility of the data, and compliance with polices, directives, and regulations.
The knowledge and skills required to use appropriate software, tools, and processes to gather, organize, analyze, visualize and manage data (Statistics Canada, 2020).
The knowledge and skills required to create meaningful tables, charts and graphics to visually present data (Statistics Canada, 2020). This also includes evaluating the effectiveness of the visual representation (i.e., using the right chart) while ensuring accuracy to avoid misrepresentation.
The knowledge and skills to assess data sources to ensure they meet the needs of the gatherer or organization (Statistics Canada, 2020). This includes both identifying errors and taking action to address the issues with the data.
The knowledge and skills required to evaluate a range of data sources and evidence in order to make decisions and take actions (Statistics Canada, 2020). This can include monitoring and evaluating the effectiveness of policies and programs.
The knowledge and skills required to use data to help in the decision-making and policy-making process (Statistics Canada, 2020). This includes thinking critically when working with data, formulating appropriate business questions, identifying appropriate datasets, deciding on measurement priorities, prioritizing information garnered from data, converting data into actionable information, and weighing the merit and impact of possible solutions and decisions.
The knowledge and skills required to extract and create meaningful documentation that will enable the correct usage and interpretation of the data (Statistics Canada, 2020). This includes the documentation of metadata which is the underlying definitions and descriptions about the data.
The knowledge and skills required to describe key points of interest in statistical information (i.e., data that has been analyzed) (Statistics Canada, 2020). This includes identifying the desired outcome of the presentation, identifying the audience's needs and level of familiarity with the subject, establishing the context, and selecting effective visualizations.
This is data about data, including the definitions and descriptions about the data, and makes finding and working with data easier.
A set of rules, models, policies, or standards that govern which data is collected, and how it is acquired, stored, arranged, secured, accessed, and used (Olavsrud, 2022).
The planning, implementation, and control activities to ensure that data services provided comply with all regulatory and legislative requirements that an organization is subject to.
The interrelated conditions in which something exists or occurs (Merriam-Webster, 2022).
A dynamic and iterative process that includes synthesis, dissemination, exchange, and ethically sound application of knowledge to improve the health of Canadians, provide more effective health services and products, and strengthen the health care system (Canadian Institutes of Health Research, 2012, p. 1).
The synthesis, exchange, and application of knowledge by relevant stakeholders to accelerate the benefits of global and local innovation in strengthening health systems and improving people’s health (World Health Organization, 2005, p. 2).