12.3 Data-Driven Analytics
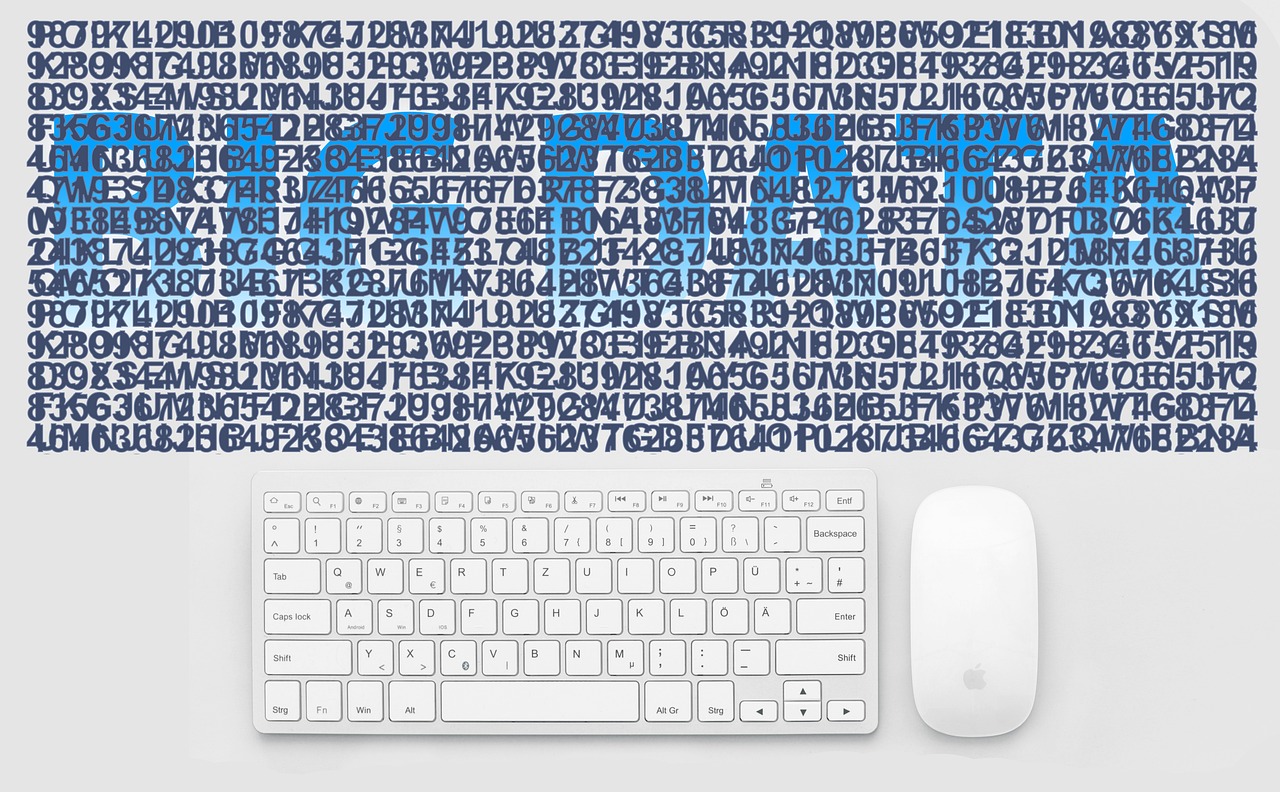
The move away from tactical thinking is shifting government procurement with data-driven decision-making. Public procurement teams use data to anticipate spending patterns, supplier performance, and market trends.
Big Data
Big data and data analytics are new paradigms in public administration practices. When implemented accurately, it produces positive public administration results in efficacy, efficiency, and citizen satisfaction (Arnaboldi & Azzone, 2020). These advantages result from a considerable increase in decision-making accuracy, a rapid internal ‘‘information task’’ performance, and a significant decrease in the running costs of the decision-making process (Bright & Margetts, 2016). This is made possible by digitizing various aspects of human life and applying information technology (IT) developments, specifically for public administration. In big data methods, large amounts of data are processed based on reasoning by robust IT to gather the information that aids public administration in effectively performing its tasks (Klievink et al., 2017)
The term ‘‘big data’’ refers to collecting large amounts of data from several sources, including human input data, process data from sensors, and various monitoring systems (Desouza & Jacob, 2017). Data is being gathered at an unprecedented rate (Lee, 2020). Massive volumes of data have facilitated substantial innovation in the public and private sectors (Suominen & Hajikhani, 2021). The world is on the verge of creating a broad utility of big data and analytics, similar to companies like Google and Amazon, which are constantly innovating consumer services. Businesses are promoting this shift because of the shift in the size of the Industrial Revolution (Khurshid et al., 2019b). However, when it comes to employing big data and data analytics in decision-making, public policy lags due to a lack of acceptance and several problems that limit the utility of these technologies (Giest, 2017).
Data availability and innovative approaches are being increasingly understood in the public and corporate sectors (Wahyunengseh & Hastjarjo, 2021). Several public administrations have implemented big data strategies or policies. In terms of efficiency and effectiveness, big data and the strategies for using them are emerging phenomena in the management landscape that provide excellent outcomes (Kandt & Batty, 2021). A private sector example is the insurance provider Aviva (Maciejewski, 2017), which created a smartphone big data app that accurately records customers’ driving patterns. Previous statistics are employed to precisely determine a driver’s risk; hence, cautious drivers pay less for insurance compared to reckless drivers. Big data opportunities in public administration are comparable to those in private-sector businesses (Visvizi et al., 2021)
To utilize big data in the public sector, the concerned authority must have data science-related knowledge and abilities (Spence, 2021). This includes software-based modelling, statistics, data management, data exploration, algorithmic machine learning, data product formatting, and computer programming. Additionally, the public sector needs a strategy tailored to the general public goals, objectives, and policies. Big data have immense potential in public administration and for the public interest, irrespective of the required labour (Suominen & Hajikhani, 2021). New techniques of big data analysis can help governments better understand the behaviour of their constituents and enhance public services (Goyal et al., 2022).
Data-Driven Public Policy Implementation
Better Supervision—Detecting Irregularities
Big data technologies can assist in finding irregularities, a critical component of a public monitoring system. A public authority monitors a certain region, looks for behavioural anomalies, and takes supervisory action if irregularities are identified. The advantages of big data may be easily demonstrated in this domain. Big data are being used here in a variety of ways, including creating the broadest collection of data resources feasible to serve as the foundation for analysis and reasoning, creating digital models of irregularities, and applying the models to massive data sets, that is, having a computer filter the data, based on the models of abnormalities. This method results in the computer system choosing and presenting the facts that adhere to an applied irregularity model. The data analysis makes it possible to link any discovered abnormality to a specific entity. Before the official administrative procedure begins, big data approaches are applied in supervisory administration at the analytical level. At this point, big data enables an authority to identify the circumstances and organizations that may have broken the law. It allows them to prepare supervisory action based on the vast information available. However, the supervisory action is carried out through conventional administrative methods, such as on-site inspection. This reduces the risks associated with automated processes (Guenduez et al., 2020). Large data allow abnormalities to be successfully and quickly discovered and assist in predictive and behavioural analytics, which can indicate the possibility of irregularities even before they occur. In actuality, the application of suitable models may detect abnormalities with a probability of up to 95%. An example is using big data to identify tax evasion and other abnormalities. The British Connect system, used by the British HM Revenue and Customs Office, is an example of successful big data analysis for this purpose. The effectiveness and efficiency of the public body increased dramatically with the use of big data. This illustrates that similar results can be attained in public administration as a whole (Xu & Liu, 2022).
Better Regulation—Promoting Awareness and Feedback
Big data techniques enable public bodies to track and manage areas in real time or with a negligible delay. As a result, the authority has access to a wide range of information for decision-making, which helps it accomplish its regulatory goals (Maciejewski, 2017). The ‘‘physics’’ of exploiting big data revolves around amassing pertinent data as rapidly as feasible. The information must be presented in a helpful way for regulatory purposes. The regulatory authority can use this method to comprehend the functioning of the people and systems and decide on necessary regulatory actions. In addition, it is possible to include automatic notifications for particular situations in big data systems designed for regulatory purposes. When a reaction is required, these notifications alert a decision-maker regarding previously established occurrences. Additionally, if an administrative policy is not operating properly, it is feasible to monitor its impact and make the required modifications. This implies that large data usage can spot abnormalities as well. Regulated situations, however, require monitoring systemic abnormalities rather than individual ones. It could draw attention to compliant circumstances; however, other elements are crucial to the regulator (Shah et al., 2021). Public entities employing big data obtain more favourable results and advantages. Big data aid in establishing and implementing stronger regulatory policies by bolstering the information intake for evidence-based decision-making and offering quick feedback on policy effects (Casanovas et al., 2017)
Public Service Delivery—Offering Particular Products or Services
Big data techniques have major advantages for government agencies that supply public services, improving the quality of their delivery (Vydra & Klievink, 2019). This results from feedback and inputs about the ‘‘clients,’’ their requirements, and their behaviour. Modern ideas of a ‘‘smart’’ city result from the widespread use of big data for urban public functions (Miljand, 2020). When a wide range of public services are provided in a way that closely satisfies consumer demands and is done so logically and effectively due to the widespread use of information technologies, particularly ‘‘big data’’ and ‘‘internet of things,’’ the city is considered ‘‘smart’’ (Kandt & Batty, 2021). This is true for all types of public services, including fire protection, social housing, social allowances, and public highways, as well as for public electricity and water networks, grids, and other infrastructure. Big data in these public tasks encourage (a) customer behaviour analysis to better understand demands and provide public services in line with needs, (b) checking applicants’ eligibility for public benefits, such as allowances, and spotting fraud, and (c) better functioning of service provider’s operations.
Policy Feedback
Big data techniques can be applied to sentiment analysis to learn public opinion about the policies. Feedback regarding policies or individual decisions is a distinct field of big data application. It enables quick action from government agencies. In addition, big data may be used to forecast public sentiment about potential government actions. Text analytics acquire and analyze internet data for sentiment analysis, including information from tweets, really simple syndication (RSS) feeds, social media, and mobile applications. The monitoring and social media analysis tool Vizie was created by the Commonwealth Scientific and Industrial Research Organization (CSIRO) (Maciejewski, 2017). To stop the spread of misinformation, for example, this program may use fresh social media material and instantly alert the authorities about the changes that may require their attention.
Challenges of Implementing a Data-Driven Policy
Government agencies need to be aware of data security and privacy. Some governments have open data rules that may result in massive data disasters if terrorists or parties with entrenched interests utilize the information (Sarker et al., 2018). Hence, government organizations must closely monitor this security concern. It must protect the privacy of citizen data since it is utilized to make decisions, catch criminals, lessen corruption, and promote social welfare (Ge & Wang, 2019). Before implementing big data technologies, a secure system needs to be developed. Government organizations use big data analytics to make decisions quickly. It must be flawless and delivered on time for immediate use. Certain government organizations preserve data flow and accessibility by using standardized formats and metadata (van der Voort et al., 2019). Many countries today have open data policies, which make data sets accessible to the general public. Collaboration between multiple agencies is aided; however, privacy policies must be followed. A steady stream of accurate, available, discoverable, and useable data is needed for smart governance (Sarker et al., 2018). Table 12.3 provides an overview of the challenges of data-driven public policy.
Major Challenges | Description | Sources |
---|---|---|
Security and privacy challenges | The government is dedicated to maintaining citizens’ security and privacy. Government agencies have clear guidelines and stringent rules regarding this purpose. However, the public must trust government institutions as safe depositions. | Giest (2017), Klievink et al. (2017), Sarker et al. (2018) |
Data sharing challenges | Data has three fundamental qualities—discoverability, usability and accessibility. Readily available knowledge is a key component for achieving a sustainable economy. The government must follow privacy laws while collecting/acquiring, using and maintaining data. Big data analytics are used by government organizations to make quick decisions. These must be flawless and delivered on time to be of immediate use. | Taylor and Schroeder (2015), Klievink et al. (2017), Kiggins (2018), Mavriki and Karyda (2020) |
Technology-related challenges | Big data technology is currently used in every industry. The development of technology has made it feasible to manage, store, and analyze a large amount of data. Big data deployment in the public sector for data collection, analysis, and storage requires enhanced hardware and software systems. Related difficulties and challenges must be appropriately controlled for government agencies to benefit from the technology. | Lavertu (2016), Klievink et al. (2017), Poel et al. (2018). Suominen and Hajikhani (2021) |
Skill-related challenges | Big data management requires a team of skilled employees. There is a dearth of data scientists in this field since it demands a broad understanding of several other fields. To avoid data disasters, government agencies must hire people who can manage the data appropriately. However, the scarcity of skilled data scientists inside government bodies may deter them from implementing this technology. | Taylor and Schroeder (2015), Hochtl et al. (2016). Klievink et al. (2017) |
Data modelling challenges | There is shortage of compatible data models for data representation and discipline-specific element expression. The representation and query of data provenance and contextual information, languages for managing data uncertainty, and languages for describing and querying data quality information require simultaneous improvement. | Panagiotopoulos et al. (2017), Studinka and Guenduez (2018), Khurshid et al. (2019) |
Data management challenges | It is important to provide high-quality, cost-effective, reliable preservation and access to data and protect its privacy, security, and intellectual property rights. In addition, it is important to maintain data search and discovery across sources and connect the data sets from various domains to create open-linked data spaces (unstructured or semi-structured). Big data sets may be too large to be efficiently handled by a single computer, making data and task parallelization necessary. As a result, different data formats or labels must be used for the same items, and distinct data input procedures and vocabularies must be reconciled. | Hotchl et al. (2016). Klievink et al. (2017), Gregory and Halff (2020), Suominen and Hajikhani (2021) |
Data services and tools challenges | A majority of scientific disciplines lack suitable data analytics tools to enable research across all stages. As a result, scientists fail to be as prolific as they need to be. This highlights the urgent need to develop ICT tools capable of cleaning, analyzing, and visualizing massive volumes of data and data tools and policies to ensure cross-fertilization and collaboration among disciplines and scientific fields. | Taylor and Schroeder (2015), Giest (2017), Gregory and Halff (2020) |
Practical Procurement: Scenarios and Solutions
Ishrat Jan, the head of the Procurement Analytics Division at a provincial public procurement agency, faced a significant challenge. The agency was responsible for overseeing the procurement of goods and services for various government departments, ensuring transparency, efficiency, and compliance with regulations. Recently, Ishrat had been tasked with addressing a growing concern: the detection and prevention of procurement irregularities. This issue was critical as it directly impacted public trust and the efficient use of taxpayer money. Ishrat knew that leveraging data analytics could be the key to solving this problem, but the path forward was not entirely clear.
The agency was a large organization with over 500 employees, responsible for procuring a wide range of products and services, from office supplies to large-scale infrastructure projects. The agency served millions of citizens, ensuring public services were delivered effectively and efficiently. The procurement process involved multiple stages: needs assessment, supplier selection, contract management, and performance evaluation. Each stage generated vast amounts of data, which, if analyzed correctly, could provide valuable insights into potential irregularities and areas for improvement. The agency had recently invested in a big data platform, but its full potential had yet to be realized.
Ishrat considered several options to address the issue of procurement irregularities. The first option was to implement a comprehensive data analytics system that could monitor procurement activities in real time. This system would use big data techniques to identify anomalies and flag suspicious activities for further investigation. By creating digital models of irregularities and applying them to the procurement data, the system could help detect potential issues early and reduce the risk of fraud and inefficiency.
The second option was to enhance the existing regulatory framework by incorporating feedback mechanisms and automatic notifications. This approach would involve setting up a system that could track the impact of procurement policies and provide real-time feedback to decision-makers. If any irregularities or inefficiencies were detected, the system would alert the relevant authorities, allowing them to take corrective action promptly. This option would help identify issues and continuously improve the procurement process based on data-driven insights.
The third option was to focus on improving public service delivery by using data analytics to better understand the needs and behaviours of citizens. By analyzing data on service usage and feedback, the agency could tailor its procurement strategies to better meet the demands of the public. This approach would involve collaborating with other government agencies and stakeholders to ensure that the procurement process was aligned with the broader goals of public service delivery. It would also help identify areas where resources could be allocated more effectively, leading to better outcomes for citizens.
Ishrat knew that each option had its own set of advantages and challenges. Implementing a real-time monitoring system would require significant investment in technology and training, but it could provide immediate benefits in detecting and preventing irregularities. Enhancing the regulatory framework would improve transparency and accountability, but it might be challenging to integrate with existing systems and processes. Focusing on public service delivery would align procurement with citizen needs, but it would require extensive collaboration and coordination with other agencies.
The business problem Ishrat faced was critical for the agency and the broader public sector. Addressing procurement irregularities was essential for maintaining public trust and ensuring the efficient use of resources. Solving this dilemma promptly was crucial, as delays could lead to further inefficiencies and potential scandals. Ishrat needed to carefully consider the options and develop a strategic plan that leveraged data analytics to drive meaningful change in public procurement.
Discussion Questions:
- How can big data techniques be used to identify and prevent procurement irregularities in public procurement?
- What are the potential benefits and challenges of implementing a real-time monitoring system for procurement activities?
- How can data analytics improve public service delivery and align procurement strategies with citizen needs?
Source: Scenario and questions created with the assistance of Microsoft Copilot.
Lean Management Practices
The application of lean practices has been growing in the public sector. Public sector organizations, pressured and required to improve their services to society, also need to adopt new and flexible management models.
The lean approach is based on five principles:
- Specify Value: Identifying what adds value to your business is hard. Customers define value in terms of specific products and services they are willing to pay for.
- Identify the Value Stream: The Value Stream is the series of individual processes that connect to create the valuable goods or services an organization produces for its customers. The process of mapping the Value Stream is designed to view the big, macro picture so executives can make strategic decisions as part of an extended organizational transformation effort.
- Make Value Flow Continuous: The best way to have a continuous flow of material and information is to eliminate the waste in the supply chain process. Having eliminated waste makes the remaining value-creating steps flow.
- Let Customers Pull Value: Organizations work in two ways: Push and Pull. Traditional organizations used to work on Push Systems whereby whatever they produced was made available for consumers to use. This no longer works in today’s market; rather, a customer’s pull cascades all the way to the lowest level supplier, enabling just-in-time production.
- Pursue Perfection: Adding value and eliminating non-value-added steps is a never-ending process. With consumers’ changing needs and demands, companies need to look into the processes repeatedly to ensure perfection.
Value Stream
The emergence of waste in supply chain activities directly impacts the availability of goods to the final consumers. One of the best ways to manage waste is to apply a lean approach with the value stream mapping technique.
As cited by Adrianto & Kholil (2015) in the article by Amrina & Fitrahaj (2020), Lean is an approach to identifying and eliminating waste or non-value-added activities through continuous improvement. Eight things cause waste (Helleno et al., 2017):
- Overproduction (excessive production)
- Unnecessary inventory (inventory that is not needed)
- Defect (defective product)
- Unnecessary motion (movements that do not add value)
- Excessive transportation (excessive material or product movement)
- Inappropriate processing (inappropriate process)
- Waiting (waiting time)
- Unutilized talent (an ability that is not utilized) (Amrina & Fitrahaj, 2020).
In the lean concept, waste can be removed through 12 techniques, one of which is value stream mapping (Amrina & Zagloel, 2019). As cited by Firdaus (2018) in the article by Amrina and Fitrahaj (2020), value stream mapping (VSM) is a method used to visualize waste in a complete process. VSM maps the process flow, information flow and material flow. By mapping current conditions, VSM helps decision-makers identify activities that do not add value.
Mapping the value-added processes within the supply chain is useful for management because it aligns stakeholders from multiple departments on the needs of the customer and the demands of the supplier. It’s also an effective tool for illustrating a product’s overall supply chain to various audiences.
Building a Value Stream Map
The Value Stream Map is a visual way to identify steps involved in the supply chain process and their relationship. It makes decision-makers see value-added and non-value-added activities clearly by drawing the whole process from upstream to downstream on paper. “To create flow, you need a vision. Mapping helps you see and focus on flow with a vision of an ideal or improved state (Rother & Shook, 2018). Exhibit 12.1 shows an example of how the Value Stream Map looks.
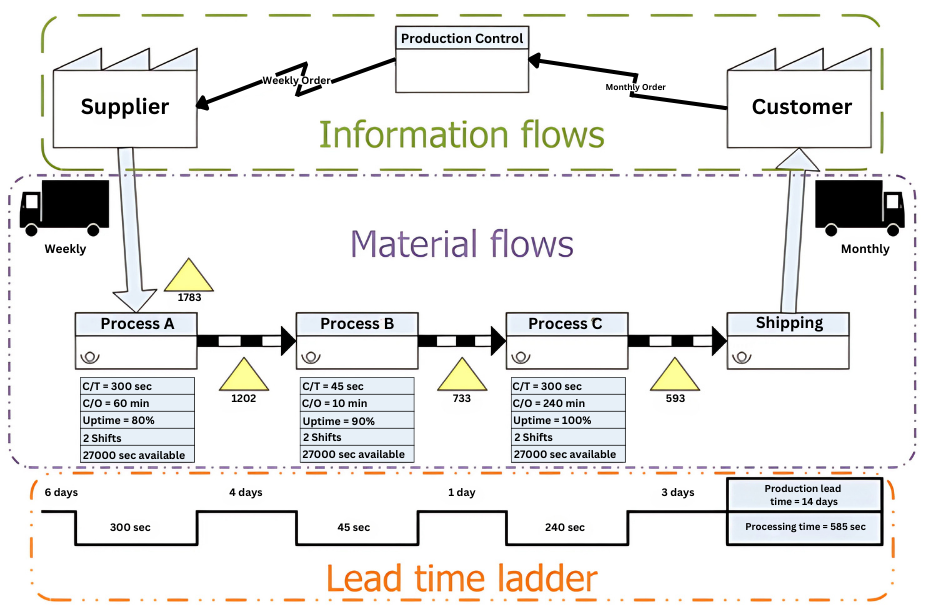
As shown in Exhibit 12.1, Value Stream Maps are divided into three sections — Information Flows, Material Flows and Lead Time Ladder. Let’s look into these sections:
- Information Flows: Information flow is located at the top half of the map and is drawn from right to left, starting with customers indicating the pull value principle. A narrow line is used to present the flow of information between customer and company and then company and supplier.
- Material Flow: Material Flow is located in the center of the map and is drawn from left to right. It provides valuable information to decision-makers and includes activities such as identifying different processes along with a few statistics such as cycle time, changeover time, uptime, batch sizes, working time and scrap rate. The icons used at this stage are very important as they represent the clear flow of material. This section highlights the waste a company creates in terms of holding over or under inventory. It is important to note that material movement is pushed by the producer and not pulled by the consumer.
- Lead Time Ladder: The bottom part is the simplest and is extremely important. It indicates the length each process takes in the value chain.
The shorter your production lead time, the shorter the time between paying for raw materials and getting paid for products made from those materials. A shorter production lead time will lead to an increase in the number of inventory turns, a measure with which you may be more familiar (Rother & Shook, 2018).
Once the current information, material flow, and lead time calculation are complete, companies tend to reach a current state map, which all the stakeholders and company representatives analyze to highlight waste and its sources. These wastes are eliminated by implementing a future-state map, which is the final stage of the value stream mapping process.
Checkpoint 12.3
Image Description
Exhibit 12.1: The image illustrates a flowchart representing a supply chain process. The chart is divided into three main sections: “Information flows” at the top, “Material flows” in the middle, followed by a “Lead time ladder” at the bottom.
In the “Information flows” section, there is an arrow pointing from Production Control” to “Supplier” labeled “Weekly Order” and another arrow from “Customer” to “Production Control” labeled “Monthly Order.”
The “Material flows” section shows a sequential flow from left to right. It starts with a truck icon labeled “Weekly” pointing to “Process A,” followed by arrows leading to “Process B,” “Process C,” and ending with “Shipping,” which is connected to another truck labeled “Monthly.” Each process box contains performance metrics: processing time (C/T), change over time (C/O), uptime percentage, number of shifts, and available seconds.
The bottom section, “Lead time ladder,” details the time allocation for each segment of the production process: “Process A” takes 6 days, “Process B” takes 4 days, “Process C” takes 1 day, and “Shipping” takes 3 days. The total “Production lead time” sums up to 14 days, with “Processing time” being 585 seconds.
Top Section (Information Flows): A triangular flow is shown with arrows indicating information moving from Production Control to the Supplier and Customer. Suppliers get weekly orders, while the Customer receives monthly orders.
Middle Section (Material Flows): The flow of materials is shown through four phases: Process A, Process B, Process C, and Shipping. Each phase has a detailed listing of cycle time, changeover time, uptime percentage, and other operational details. Materials start at Process A with 1783 units, transitioning through stages with counts like 1202 units between A and B, ending at 593 units ready for shipping.
Bottom Section (Lead Time Ladder): Illustrates the lead time for each process. Each step lists days and seconds, starting with 6 days for Process A, decreasing through the stages down to 3 days for Shipping. The total production lead time is 14 days and the total processing time is 585 seconds.
[back]
Attributions
“12.3 Data-Driven Analysis” is remixed and adapted from the following:
“Big Data-Driven Public Policy Decisions: Transformation Toward Smart Governance,” copyright © 2023 by Md Altab Hossin, Jie Du and Isaac Owusu Asante in Sage Open, licensed under a Creative Commons – Attribution 4.0 International License.
“Chapter 9: Emerging Value Chain Concepts,” in Global Value Chain, copyright © 2022 by Dr. Kiranjot Kaur and Iuliia Kau and used under a Creative Commons Attribution-NonCommercial-ShareAlike 4.0 International License, except where otherwise noted.
Exhibit 12.1 is taken from Components of a Value Stream Map, copyright © 2013 by Daniel Penfield from Wikimedia Commons, licensed under a Creative Commons Attribution-ShareAlike 3.0 License.
“Practical Procurement: Scenarios and Solutions” box was created with the assistance of Microsoft Copilot and is shared under the Creative Commons – CC0 1.0 Universal License.
The multiple choice questions in the Checkpoint boxes were created using the output from the Arizona State University Question Generator tool and are shared under the Creative Commons – CC0 1.0 Universal License.
Image descriptions and alt text for the exhibits were created using the Arizona State University Image Accessibility Creator and are shared under the Creative Commons – CC0 1.0 Universal License.
Identifying areas that add benefit to a business.
The set of actions that must take place to add value for a customer from the initial request through realization of value by the customer.
The elimination of waste that makes the remaining value-creating steps flow.
Value for customers is maximized when it is created in response to real demand and a continuous and uninterrupted flow.
A method used to visualize waste in a complete process. VSM maps the flow of process, information, and material.
A visual way to identify steps involved in the supply chain process and their relationship.
The inventory turnover is a measure of the number of times inventory is sold or used in a time period.
Document showing how things should work in order to gain the best competitive advantage.