29 Correlational Research
Learning Objectives
- Define correlational research and give several examples.
- Explain why a researcher might choose to conduct correlational research rather than experimental research or another type of non-experimental research.
- Interpret the strength and direction of different correlation coefficients.
- Explain why correlation does not imply causation.
What Is Correlational Research?
Correlational research is a type of non-experimental research in which the researcher measures two variables (binary or continuous) and assesses the statistical relationship (i.e., the correlation) between them with little or no effort to control extraneous variables. There are many reasons that researchers interested in statistical relationships between variables would choose to conduct a correlational study rather than an experiment. The first is that they do not believe that the statistical relationship is a causal one or are not interested in causal relationships. Recall two goals of science are to describe and to predict and the correlational research strategy allows researchers to achieve both of these goals. Specifically, this strategy can be used to describe the strength and direction of the relationship between two variables and if there is a relationship between the variables then the researchers can use scores on one variable to predict scores on the other (using a statistical technique called regression, which is discussed further in the section on Complex Correlation in this chapter).
Another reason that researchers would choose to use a correlational study rather than an experiment is that the statistical relationship of interest is thought to be causal, but the researcher cannot manipulate the independent variable because it is impossible, impractical, or unethical. For example, while a researcher might be interested in the relationship between the frequency people use cannabis and their memory abilities they cannot ethically manipulate the frequency that people use cannabis. As such, they must rely on the correlational research strategy; they must simply measure the frequency that people use cannabis and measure their memory abilities using a standardized test of memory and then determine whether the frequency people use cannabis is statistically related to memory test performance.
Correlation is also used to establish the reliability and validity of measurements. For example, a researcher might evaluate the validity of a brief extraversion test by administering it to a large group of participants along with a longer extraversion test that has already been shown to be valid. This researcher might then check to see whether participants’ scores on the brief test are strongly correlated with their scores on the longer one. Neither test score is thought to cause the other, so there is no independent variable to manipulate. In fact, the terms independent variable and dependent variable do not apply to this kind of research.
Another strength of correlational research is that it is often higher in external validity than experimental research. Recall there is typically a trade-off between internal validity and external validity. As greater controls are added to experiments, internal validity is increased but often at the expense of external validity as artificial conditions are introduced that do not exist in reality. In contrast, correlational studies typically have low internal validity because nothing is manipulated or controlled but they often have high external validity. Since nothing is manipulated or controlled by the experimenter the results are more likely to reflect relationships that exist in the real world.
Finally, extending upon this trade-off between internal and external validity, correlational research can help to provide converging evidence for a theory. If a theory is supported by a true experiment that is high in internal validity as well as by a correlational study that is high in external validity then the researchers can have more confidence in the validity of their theory. As a concrete example, correlational studies establishing that there is a relationship between watching violent television and aggressive behavior have been complemented by experimental studies confirming that the relationship is a causal one (Bushman & Huesmann, 2001)[1].
Does Correlational Research Always Involve Quantitative Variables?
A common misconception among beginning researchers is that correlational research must involve two quantitative variables, such as scores on two extraversion tests or the number of daily hassles and number of symptoms people have experienced. However, the defining feature of correlational research is that the two variables are measured—neither one is manipulated—and this is true regardless of whether the variables are quantitative or categorical. Imagine, for example, that a researcher administers the Rosenberg Self-Esteem Scale to 50 American college students and 50 Japanese college students. Although this “feels” like a between-subjects experiment, it is a correlational study because the researcher did not manipulate the students’ nationalities. The same is true of the study by Cacioppo and Petty comparing college faculty and factory workers in terms of their need for cognition. It is a correlational study because the researchers did not manipulate the participants’ occupations.
Figure 6.2 shows data from a hypothetical study on the relationship between whether people make a daily list of things to do (a “to-do list”) and stress. Notice that it is unclear whether this is an experiment or a correlational study because it is unclear whether the independent variable was manipulated. If the researcher randomly assigned some participants to make daily to-do lists and others not to, then it is an experiment. If the researcher simply asked participants whether they made daily to-do lists, then it is a correlational study. The distinction is important because if the study was an experiment, then it could be concluded that making the daily to-do lists reduced participants’ stress. But if it was a correlational study, it could only be concluded that these variables are statistically related. Perhaps being stressed has a negative effect on people’s ability to plan ahead (the directionality problem). Or perhaps people who are more conscientious are more likely to make to-do lists and less likely to be stressed (the third-variable problem). The crucial point is that what defines a study as experimental or correlational is not the variables being studied, nor whether the variables are quantitative or categorical, nor the type of graph or statistics used to analyze the data. What defines a study is how the study is conducted.
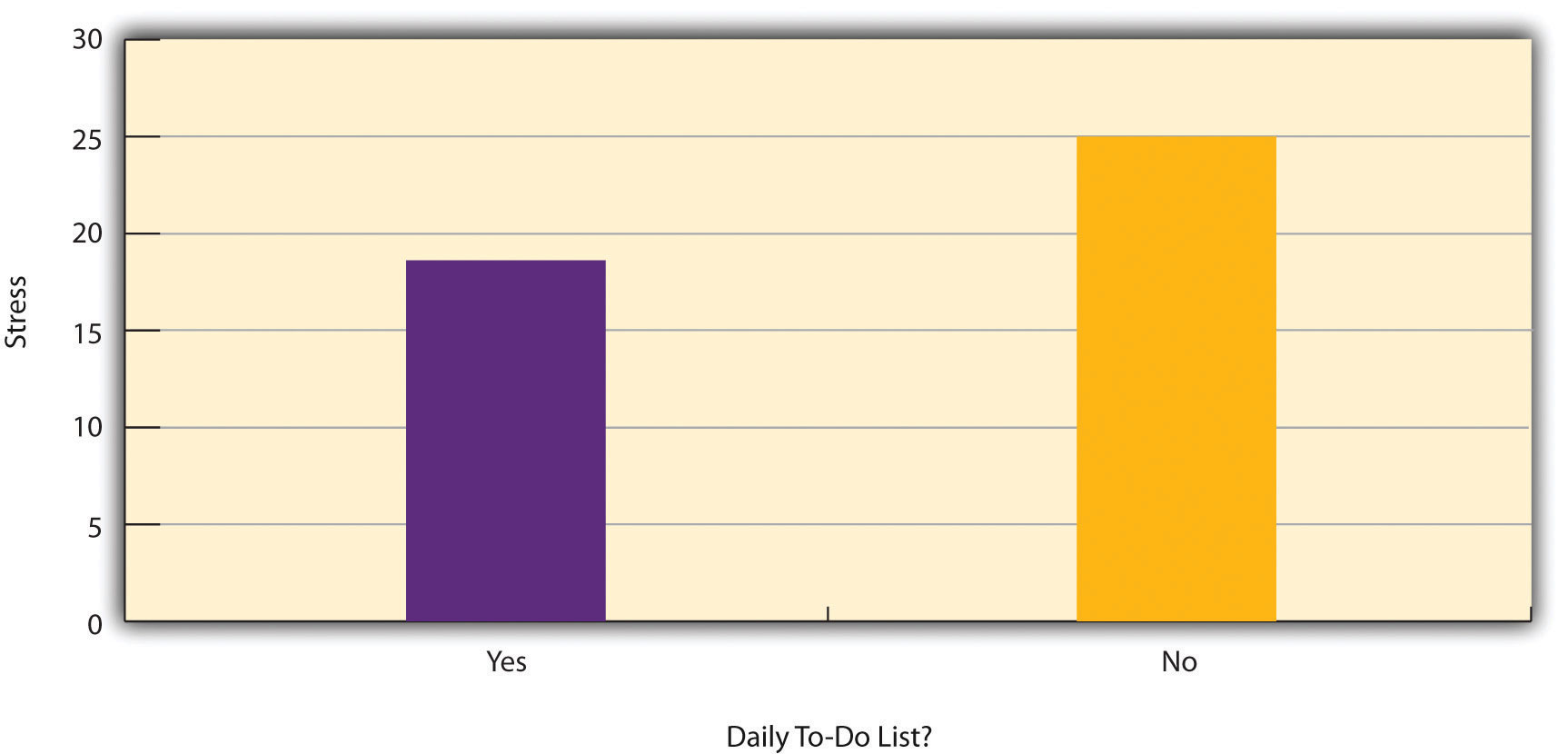
Data Collection in Correlational Research
Again, the defining feature of correlational research is that neither variable is manipulated. It does not matter how or where the variables are measured. A researcher could have participants come to a laboratory to complete a computerized backward digit span task and a computerized risky decision-making task and then assess the relationship between participants’ scores on the two tasks. Or a researcher could go to a shopping mall to ask people about their attitudes toward the environment and their shopping habits and then assess the relationship between these two variables. Both of these studies would be correlational because no independent variable is manipulated.
Correlations Between Quantitative Variables
Correlations between quantitative variables are often presented using scatterplots. Figure 6.3 shows some hypothetical data on the relationship between the amount of stress people are under and the number of physical symptoms they have. Each point in the scatterplot represents one person’s score on both variables. For example, the circled point in Figure 6.3 represents a person whose stress score was 10 and who had three physical symptoms. Taking all the points into account, one can see that people under more stress tend to have more physical symptoms. This is a good example of a positive relationship, in which higher scores on one variable tend to be associated with higher scores on the other. In other words, they move in the same direction, either both up or both down. A negative relationship is one in which higher scores on one variable tend to be associated with lower scores on the other. In other words, they move in opposite directions. There is a negative relationship between stress and immune system functioning, for example, because higher stress is associated with lower immune system functioning.

The strength of a correlation between quantitative variables is typically measured using a statistic called Pearson’s Correlation Coefficient (or Pearson's r). As Figure 6.4 shows, Pearson’s r ranges from −1.00 (the strongest possible negative relationship) to +1.00 (the strongest possible positive relationship). A value of 0 means there is no relationship between the two variables. When Pearson’s r is 0, the points on a scatterplot form a shapeless “cloud.” As its value moves toward −1.00 or +1.00, the points come closer and closer to falling on a single straight line. Correlation coefficients near ±.10 are considered small, values near ± .30 are considered medium, and values near ±.50 are considered large. Notice that the sign of Pearson’s r is unrelated to its strength. Pearson’s r values of +.30 and −.30, for example, are equally strong; it is just that one represents a moderate positive relationship and the other a moderate negative relationship. With the exception of reliability coefficients, most correlations that we find in Psychology are small or moderate in size. The website http://rpsychologist.com/d3/correlation/, created by Kristoffer Magnusson, provides an excellent interactive visualization of correlations that permits you to adjust the strength and direction of a correlation while witnessing the corresponding changes to the scatterplot.

There are two common situations in which the value of Pearson’s r can be misleading. Pearson’s r is a good measure only for linear relationships, in which the points are best approximated by a straight line. It is not a good measure for nonlinear relationships, in which the points are better approximated by a curved line. Figure 6.5, for example, shows a hypothetical relationship between the amount of sleep people get per night and their level of depression. In this example, the line that best approximates the points is a curve—a kind of upside-down “U”—because people who get about eight hours of sleep tend to be the least depressed. Those who get too little sleep and those who get too much sleep tend to be more depressed. Even though Figure 6.5 shows a fairly strong relationship between depression and sleep, Pearson’s r would be close to zero because the points in the scatterplot are not well fit by a single straight line. This means that it is important to make a scatterplot and confirm that a relationship is approximately linear before using Pearson’s r. Nonlinear relationships are fairly common in psychology, but measuring their strength is beyond the scope of this book.

The other common situations in which the value of Pearson’s r can be misleading is when one or both of the variables have a limited range in the sample relative to the population. This problem is referred to as restriction of range. Assume, for example, that there is a strong negative correlation between people’s age and their enjoyment of hip hop music as shown by the scatterplot in Figure 6.6. Pearson’s r here is −.77. However, if we were to collect data only from 18- to 24-year-olds—represented by the shaded area of Figure 6.6—then the relationship would seem to be quite weak. In fact, Pearson’s r for this restricted range of ages is 0. It is a good idea, therefore, to design studies to avoid restriction of range. For example, if age is one of your primary variables, then you can plan to collect data from people of a wide range of ages. Because restriction of range is not always anticipated or easily avoidable, however, it is good practice to examine your data for possible restriction of range and to interpret Pearson’s r in light of it. (There are also statistical methods to correct Pearson’s r for restriction of range, but they are beyond the scope of this book).

Correlation Does Not Imply Causation
You have probably heard repeatedly that “Correlation does not imply causation.” An amusing example of this comes from a 2012 study that showed a positive correlation (Pearson’s r = 0.79) between the per capita chocolate consumption of a nation and the number of Nobel prizes awarded to citizens of that nation[2]. It seems clear, however, that this does not mean that eating chocolate causes people to win Nobel prizes, and it would not make sense to try to increase the number of Nobel prizes won by recommending that parents feed their children more chocolate.
There are two reasons that correlation does not imply causation. The first is called the directionality problem. Two variables, X and Y, can be statistically related because X causes Y or because Y causes X. Consider, for example, a study showing that whether or not people exercise is statistically related to how happy they are—such that people who exercise are happier on average than people who do not. This statistical relationship is consistent with the idea that exercising causes happiness, but it is also consistent with the idea that happiness causes exercise. Perhaps being happy gives people more energy or leads them to seek opportunities to socialize with others by going to the gym. The second reason that correlation does not imply causation is called the third-variable problem. Two variables, X and Y, can be statistically related not because X causes Y, or because Y causes X, but because some third variable, Z, causes both X and Y. For example, the fact that nations that have won more Nobel prizes tend to have higher chocolate consumption probably reflects geography in that European countries tend to have higher rates of per capita chocolate consumption and invest more in education and technology (once again, per capita) than many other countries in the world. Similarly, the statistical relationship between exercise and happiness could mean that some third variable, such as physical health, causes both of the others. Being physically healthy could cause people to exercise and cause them to be happier. Correlations that are a result of a third-variable are often referred to as spurious correlations.
Some excellent and amusing examples of spurious correlations can be found at http://www.tylervigen.com (Figure 6.7 provides one such example).

“Lots of Candy Could Lead to Violence”
Although researchers in psychology know that correlation does not imply causation, many journalists do not. One website about correlation and causation, http://jonathan.mueller.faculty.noctrl.edu/100/correlation_or_causation.htm, links to dozens of media reports about real biomedical and psychological research. Many of the headlines suggest that a causal relationship has been demonstrated when a careful reading of the articles shows that it has not because of the directionality and third-variable problems.
One such article is about a study showing that children who ate candy every day were more likely than other children to be arrested for a violent offense later in life. But could candy really “lead to” violence, as the headline suggests? What alternative explanations can you think of for this statistical relationship? How could the headline be rewritten so that it is not misleading?
As you have learned by reading this book, there are various ways that researchers address the directionality and third-variable problems. The most effective is to conduct an experiment. For example, instead of simply measuring how much people exercise, a researcher could bring people into a laboratory and randomly assign half of them to run on a treadmill for 15 minutes and the rest to sit on a couch for 15 minutes. Although this seems like a minor change to the research design, it is extremely important. Now if the exercisers end up in more positive moods than those who did not exercise, it cannot be because their moods affected how much they exercised (because it was the researcher who used random assignment to determine how much they exercised). Likewise, it cannot be because some third variable (e.g., physical health) affected both how much they exercised and what mood they were in. Thus experiments eliminate the directionality and third-variable problems and allow researchers to draw firm conclusions about causal relationships.
- Bushman, B. J., & Huesmann, L. R. (2001). Effects of televised violence on aggression. In D. Singer & J. Singer (Eds.), Handbook of children and the media (pp. 223–254). Thousand Oaks, CA: Sage. ↵
- Messerli, F. H. (2012). Chocolate consumption, cognitive function, and Nobel laureates. New England Journal of Medicine, 367, 1562-1564. ↵
Learning Objectives
- Identify the key components of empirical journal articles
- Define the basic elements of the results section in a journal article
- Describe statistical significance and confidence intervals
Reading scholarly articles can be a more challenging than reading a book, magazine, news article—or even some textbooks. Theoretical and practical articles are, generally speaking, easier to understand. Empirical articles, because they add new knowledge, must go through great detail to demonstrate that the information they offer is based on solid science. Empirical articles can be challenging to read, and this section is designed to make that process easier for you.
Nearly all articles will have an abstract, the short paragraph at the beginning of an article that summarizes the author’s research question, methods used to answer the question, and key findings. The abstract may also give you some idea about the theoretical perspective of the author. In effect, the abstract provides you with a framework to understand the rest of the article and the article's punch line: what the author(s) found, and whether the article is relevant to your area of inquiry. For this reason, I suggest skimming abstracts as part of the literature search process.
As you will recall from Chapter 2, theoretical articles have no set structure and will look similar to reading a chapter of a book. Empirical articles contain the following sections (although exact section names vary): introduction, methods, results, and discussion. The introduction contains the literature review for the article and is an excellent source of information as you build your own literature review. The methods section reviews how the author gathered their sample, how they measured their variables, and how the data were analyzed. The results section provides an in-depth discussion of the findings of the study. The discussion section reviews the main findings and addresses how those findings fit in with the existing literature. At the end, there will be a list of references (which you should read!) and there may be a few tables, figures, or appendices if applicable.
While you should get into the habit of familiarizing yourself with each part of the articles you wish to cite, there are strategic ways to read journal articles that can make them a little easier to digest. Once you have read the abstract for an article and determined it is one you’d like to read in full, read through the introduction and discussion sections next. The introduction section will showcase other articles and findings that are significant in your topic area, so reading through it will be beneficial for your own information-gathering process for your literature review. Reading an article’s discussion section helps you understand what the author views as their study’s major findings and how the author perceives those findings to relate to other research.
As you progress through your research methods course, you will pick up additional research elements that are important to understand. You will learn how to identify qualitative and quantitative methods, as well as exploratory, explanatory, and descriptive research methods. You will also learn the criteria for establishing causality and the different types of causality. Subsequent chapters of this textbook will address other elements of journal articles, including choices about measurement, sampling, and design. As you learn about these additional items, you will find that the methods and results sections begin to make more sense and you will understand how the authors reached their conclusions.
As you read a research report, there are several questions you can ask yourself about each section, from abstract to conclusion. Those questions are summarized in Table 3.1. Keep in mind that the questions covered here are designed to help you, the reader, to think critically about the research you come across and to get a general understanding of the strengths, weaknesses, and key takeaways from a given study. I hope that by considering how you might respond to the following questions while reading research reports, you’ll gain confidence in describing the report to others and discussing its meaning and impact with them.
Report section | Questions worth asking |
Abstract | What are the key findings? How were those findings reached? What framework does the researcher employ? |
Acknowledgments | Who are this study’s major stakeholders? Who provided feedback? Who provided support in the form of funding or other resources? |
Problem statement (introduction) | How does the author frame their research focus? What other possible ways of framing the problem exist? Why might the author have chosen this particular way of framing the problem? |
Literature review (introduction) |
How selective does the researcher appear to have been in identifying relevant literature to discuss? Does the review of literature appear appropriately extensive? Does the researcher provide a critical review? |
Sample (methods) | Where was the data collected? Did the researcher collect their own data or use someone else's data? What population is the study trying to make claims about, and does the sample represent that population well? What are the sample’s major strengths and major weaknesses? |
Data collection (methods) | How were the data collected? What do you know about the relative strengths and weaknesses of the method employed? What other methods of data collection might have been employed, and why was this particular method employed? What do you know about the data collection strategy and instruments (e.g., questions asked, locations observed)? What don’t you know about the data collection strategy and instruments? |
Data analysis (methods) | How were the data analyzed? Is there enough information provided for you to feel confident that the proper analytic procedures were employed accurately? |
Results | What are the study’s major findings? Are findings linked back to previously described research questions, objectives, hypotheses, and literature? Are sufficient amounts of data (e.g., quotes and observations in qualitative work, statistics in quantitative work) provided in order to support conclusions drawn? Are tables readable? |
Discussion/conclusion | Does the author generalize to some population beyond her/his/their sample? How are these claims presented? Are claims made supported by data provided in the results section (e.g., supporting quotes, statistical significance)? Have limitations of the study been fully disclosed and adequately addressed? Are implications sufficiently explored? |
Understanding the results section
As mentioned previously in this chapter, reading the abstract that appears in most reports of scholarly research will provide you with an excellent, easily digestible review of a study’s major findings and of the framework the author is using to position their findings. Abstracts typically contain just a few hundred words, so reading them is a nice way to quickly familiarize yourself with a study. If the study seems relevant to your paper, it’s probably worth reading more. If it’s not, then you have only spent a minute or so reading the abstract. Another way to get a snapshot of the article is to scan the headings, tables, and figures throughout the report (Green & Simon, 2012). [1]
At this point, I have read hundreds of literature reviews written by students. One of the challenges I have noted is that students will report the summarized results from the abstract, rather than the detailed findings in the results section of the article. This is a problem when you are writing a literature review because you need to provide specific and clear facts that support your reading of the literature. The abstract may say something like: “we found that poverty is associated with mental health status.” For your literature review, you want the details, not the summary. In the results section of the article, you may find a sentence that states: “for households in poverty, children are three times more likely to have a mental health diagnosis.” This more detailed information provides a stronger basis on which to build a literature review.
Using the summarized results in an abstract is an understandable mistake to make. The results section often contains terminology, diagrams, and symbols that may be hard to understand without having completed advanced coursework on statistical or qualitative analysis. To that end, the purpose of this section is to improve reading comprehension by providing an introduction to the basic components of a results section.
Journal articles often contain tables, and scanning them is a good way to begin reading an article. A table provides a quick, condensed summary of the report’s key findings. The use of tables is not limited to one form or type of data, though they are used most commonly in quantitative research. Tables are a concise way to report large amounts of data. Some tables present descriptive information about a researcher’s sample, which is often the first table in a results section. These tables will likely contain frequencies (N) and percentages (%). For example, if gender happened to be an important variable for the researcher’s analysis, a descriptive table would show how many and what percent of all study participants are women, men, or other genders. Frequencies or counts will probably be listed as N, while the percent symbol (%) might be used to indicate percentages.
In a table presenting a causal relationship, two sets of variables are represented. The independent variable, or cause, and the dependent variable, the effect. We’ll go into more detail on variables in Chapter 6. The independent variable attributes are typically presented in the table’s columns, while dependent variable attributes are presented in rows. This allows the reader to scan across a table’s rows to see how values on the dependent variable attributes change as the independent variable attribute values change. Tables displaying results of quantitative analysis will also likely include some information about the strength and statistical significance of the relationships presented in the table. These details tell the reader how likely it is that the relationships presented will have occurred simply by chance.
Let’s look at a specific example. Table 3.2 shows data from a study of older adults that was conducted by Dr. Blackstone, an original author of this textbook. It presents the causal relationship between gender and the experience of harassing behaviors in the workplace. In this example, gender is the independent variable (the cause) and the harassing behaviors listed are the dependent variables (the effects). [2] Therefore, we place gender in the table’s columns and harassing behaviors in the table’s rows.
Reading across the table’s top row, we see that 2.9% of women in the sample reported experiencing subtle or obvious threats to their safety at work, while 4.7% of men in the sample reported the same. We can read across each of the rows of the table in this way. Reading across the bottom row, we see that 9.4% of women in the sample reported experiencing staring or invasion of their personal space at work while just 2.3% of men in the sample reported having the same experience. We’ll discuss p value later in this section.
Behavior Experienced at work | Women | Men | p value |
Subtle or obvious threats to your safety | 2.9% | 4.7% | 0.623 |
Being hit, pushed, or grabbed | 2.2% | 4.7% | 0.480 |
Comments or behaviors that demean your gender | 6.5% | 2.3% | 0.184 |
Comments or behaviors that demean your age | 13.8% | 9.3% | 0.407 |
Staring or invasion of your personal space | 9.4% | 2.3% | 0.039 |
Note: Sample size was 138 for women and 43 for men. |
These statistics represent what the researchers found in their sample, and they are using their sample to make conclusions about the true population of all employees in the real world. Because the methods we use in social science are never perfect, there is some amount of error in that value. The researchers in this study estimated the true value we would get if we asked every employee in the world the same questions on our survey. Researchers will often provide a confidence interval, or a range of values in which the true value is likely to be, to provide a more accurate description of their data. For example, at the time I’m writing this, my wife and I are expecting our first child next month. The doctor told us our due date was August 15th. But the doctor also told us that August 15th was only their best estimate. They were actually 95% sure our baby might be born any time between August 1st and September 1st. Confidence intervals are often listed with a percentage, like 90% or 95%, and a range of values, such as between August 1st and September 1st. You can read that as: we are 95% sure your baby will be born between August 1st and September 1st. So, while we get a due date of August 15th, the uncertainty about the exact date is reflected in the confidence interval provided by our doctor.
Of course, we cannot assume that these patterns didn’t simply occur by chance. How confident can we be that the findings presented in the table did not occur by chance? This is where tests of statistical significance come in handy. Statistical significance tells us the likelihood that the relationships we observe could be caused by something other than chance. While your statistics class will give you more specific details on tests of statistical significance and reading quantitative tables, the important thing to be aware of as a non-expert reader of tables is that some of the relationships presented will be statistically significant and others may not be. Tables should provide information about the statistical significance of the relationships presented. When reading a researcher’s conclusions, pay attention to which relationships are statistically significant and which are not.
In Table 3.2, you may have noticed that a p value is noted in the very last column of the table. A p value is a statistical measure of the probability that there is no relationship between the variables under study. Another way of putting this is that the p value provides guidance on whether or not we should reject the null hypothesis. The null hypothesis is simply the assumption that no relationship exists between the variables in question. In Table 3.2, we see that for the first behavior listed, the p value is 0.623. This means that there is a 62.3% chance that the null hypothesis is correct in this case. In other words, it seems likely that any relationship between observed gender and experiencing threats to safety at work in this sample is simply due to chance.
In the final row of the table, however, we see that the p value is 0.039. In other words, there is a 3.9% chance that the null hypothesis is correct. Thus, we can be somewhat more confident than in the preceding example that there may be some relationship between a person’s gender and their experiencing the behavior noted in this row. Statistical significance is reported in reference to a value, usually 0.05 in the social science. This means that the probability that the relationship between gender and experiencing staring or invasion of personal space at work is due to random chance is less than 5 in 100. Social science often uses 0.05, but other values are used. Studies using 0.1 are using a more forgiving standard of significance, and therefore, have a higher likelihood of error (10%). Studies using 0.01 are using a more stringent standard of significance, and therefore, have a lower likelihood of error (1%).
Notice that I’m hedging my bets here by using words like somewhat and may be. When testing hypotheses, social scientists generally phrase their findings in terms of rejecting the null hypothesis rather than making bold statements about the relationships observed in their tables. You can learn more about creating tables, reading tables, and tests of statistical significance in a class focused exclusively on statistical analysis. For now, I hope this brief introduction to reading tables will improve your confidence in reading and understanding the quantitative tables you encounter while reading reports of social science research.
A final caveat is worth noting here. The previous discussion of tables and reading the results section is applicable to quantitative articles. Quantitative articles will contain a lot of numbers and the results of statistical tests demonstrating association between those numbers. Qualitative articles, on the other hand, will consist mostly of quotations from participants. For most qualitative articles, the authors want to put their results in the words of their participants, as they are the experts. The results section may be organized by theme, with each paragraph or subsection illustrating through quotes how the authors interpret what people in their study said.
Key Takeaways
- Reading a research article requires reading beyond the abstract.
- In tables presenting causal relationships, the independent variable is typically presented in the table’s columns while the dependent variables are presented in the table’s rows.
- When reading a research report, there are several key questions you should ask yourself for each section of the report.
Glossary
Abstract- the short paragraph at the beginning of an article that summarizes its main point
Confidence interval- a range of values in which the true value is likely to be
Null hypothesis- the assumption that no relationship exists between the variables in question
p-value- a statistical measure of the probability that there is no relationship between the variables under study
Statistical significance- the likelihood that the relationships that are observed could be caused by something other than chance
Table- a quick, condensed summary of the report’s key findings
Image Attributions
CSAF releases 2009 reading list by Master Sgt. Steven Goetsch public domain
A relationship in which higher scores on one variable tend to be associated with higher scores on the other.
A relationship in which higher scores on one variable tend to be associated with lower scores on the other.
A statistic that measures the strength of a correlation between quantitative variables.
Research that combines both quantitative and qualitative approaches.
Learning Objectives
- Differentiate among exploratory, descriptive, and explanatory research studies
A recent news story on college students’ addictions to electronic gadgets (Lisk, 2011) [3] describes current research findings from Professor Susan Moeller and colleagues from the University of Maryland (http://withoutmedia.wordpress.com). The story raises a number of interesting questions. Just what sorts of gadgets are students addicted to? How do these addictions work? Why do they exist, and who is most likely to experience them?
Social science research is great for answering these types of questions, but to answer them thoroughly, we must take care in designing our research projects. In this chapter, we’ll discuss what aspects of a research project should be considered at the beginning, including specifying the goals of the research, the components that are common across most research projects, and a few other considerations.
When designing a research project, you should first consider what you hope to accomplish by conducting the research. What do you hope to be able to say about your topic? Do you hope to gain a deep understanding of the phenomenon you’re studying, or would you rather have a broad, but perhaps less deep, understanding? Do you want your research to be used by policymakers or others to shape social life, or is this project more about exploring your curiosities? Your answers to each of these questions will shape your research design.
Exploration, description, and explanation
In the beginning phases, you’ll need to decide whether your research will be exploratory, descriptive, or explanatory. Each has a different purpose, so how you design your research project will be determined in part by this decision.
Researchers conducting exploratory research are typically in the early stages of examining their topics. These sorts of projects are usually conducted when a researcher wants to test the feasibility of conducting a more extensive study and to figure out the “lay of the land” with respect to the particular topic. Perhaps very little prior research has been conducted on this subject. If this is the case, a researcher may wish to do some exploratory work to learn what method to use in collecting data, how best to approach research subjects, or even what sorts of questions are reasonable to ask. A researcher wanting to simply satisfy their curiosity about a topic could also conduct exploratory research. For example, an exploratory study may be a suitable step toward understanding the relatively new phenomenon of college students' addictions to their electronic gadgets.
It is important to note that exploratory designs do not make sense for topic areas with a lot of existing research. For example, it would not make much sense to conduct an exploratory study on common interventions for parents who neglect their children because the topic has already been extensive study in this area. Exploratory questions are best suited to topics that have not been studied. Students may justify an exploratory approach to their project by claiming that there is very little literature on their topic. Most of the time, the student simply needs more direction on where to search, however each semester a few topics are chosen for which there actually is a lack of literature. Perhaps there would be less available literature if a student set out to study child neglect interventions for parents who identify as transgender or parents who are refugees from the Syrian civil war. In that case, an exploratory design would make sense as there is less literature to guide your study.
Another purpose of research is to describe or define a particular phenomenon, termed descriptive research. For example, a social work researcher may want to understand what it means to be a first-generation college student or a resident in a psychiatric group home. In this case, descriptive research would be an appropriate strategy. A descriptive study of college students’ addictions to their electronic gadgets, for example, might aim to describe patterns in how many hours students use gadgets or which sorts of gadgets students tend to use most regularly.
Researchers at the Princeton Review conduct descriptive research each year when they set out to provide students and their parents with information about colleges and universities around the United States. They describe the social life at a school, the cost of admission, and student-to-faculty ratios among other defining aspects. Although students and parents may be able to obtain much of this information on their own, having access to the data gathered by a team of researchers is much more convenient and less time consuming.
Social workers often rely on descriptive research to tell them about their service area. Keeping track of the number of children receiving foster care services, their demographic makeup (e.g., race, gender), and length of time in care are excellent examples of descriptive research. On a macro-level, the Centers for Disease Control provides a remarkable amount of descriptive research on mental and physical health conditions. In fact, descriptive research has many useful applications, and you probably rely on findings from descriptive research without even being aware.
Finally, social work researchers often aim to explain why particular phenomena work in the way that they do. Research that answers “why” questions is referred to as explanatory research. In this case, the researcher is trying to identify the causes and effects of whatever phenomenon they are studying. An explanatory study of college students’ addictions to their electronic gadgets might aim to understand why students become addicted. Does the addiction have anything to do with their family histories, extracurricular hobbies and activities, or with whom they spend their time? An explanatory study could answer these kinds of questions.
There are numerous examples of explanatory social scientific investigations. For example, the recent work of Dominique Simons and Sandy Wurtele (2010) [4] sought to discover whether receiving corporal punishment from parents led children to turn to violence in solving their interpersonal conflicts with other children. In their study of 102 families with children between the ages of 3 and 7, the researchers found that experiencing frequent spanking did, in fact, result in children being more likely to accept aggressive problem-solving techniques. Another example of explanatory research can be seen in Robert Faris and Diane Felmlee’s (2011) [5] research study on the connections between popularity and bullying. From their study of 8th, 9th, and 10th graders in 19 North Carolina schools, they found that aggression increased as adolescents’ popularity increased. [6]
The choice between descriptive, exploratory, and explanatory research should be made with your research question in mind. What does your question ask? Are you trying to learn the basics about a new area, establish a clear “why” relationship, or define or describe an activity or concept? In the next section, we will explore how each type of research is associated with different methods, paradigms, and forms of logic.
Key Takeaways
- Exploratory research is usually conducted when a researcher has just begun an investigation and wishes to understand the topic generally.
- Descriptive research aims to describe or define the topic at hand.
- Explanatory research is aims to explain why particular phenomena work in the way that they do.
Glossary
Descriptive research- describes or defines a particular phenomenon
Explanatory research- explains why particular phenomena work in the way that they do, answers “why” questions
Exploratory research- conducted during the early stages of a project, usually when a researcher wants to test the feasibility of conducting a more extensive study