30 Complex Correlation
Learning Objectives
- Explain some reasons that researchers use complex correlational designs.
- Create and interpret a correlation matrix.
- Describe how researchers can use partial correlation and multiple regression to statistically control for third variables.
As we have already seen, researchers conduct correlational studies rather than experiments when they are interested in noncausal relationships or when they are interested in causal relationships but the independent variable cannot be manipulated for practical or ethical reasons. In this section, we look at some approaches to complex correlational research that involve measuring several variables and assessing the relationships among them.
Assessing Relationships Among Multiple Variables
Most complex correlational research involves measuring several variables—either binary or continuous—and then assessing the statistical relationships among them. For example, researchers Nathan Radcliffe and William Klein studied a sample of middle-aged adults to see how their level of optimism (measured by using a short questionnaire called the Life Orientation Test) relates to several other variables related to having a heart attack (Radcliffe & Klein, 2002)[1]. These included their health, their knowledge of heart attack risk factors, and their beliefs about their own risk of having a heart attack. They found that more optimistic participants were healthier (e.g., they exercised more and had lower blood pressure), knew about heart attack risk factors, and correctly believed their own risk to be lower than that of their peers.
In another example, Ernest Jouriles and his colleagues measured adolescents’ experiences of physical and psychological relationship aggression and their psychological distress. Because measures of physical aggression (such as the Conflict in Adolescent Dating Relationships Inventory and the Relationship Violence Interview) often tend to result in highly skewed distributions, the researchers transformed their measures of physical aggression into a dichotomous (i.e., binary) measure (0 = did not occur, 1 = did occur). They did the same with their measures of psychological aggression and then measured the correlations among these variables, finding that adolescents who experienced physical aggression were moderately likely to also have experienced psychological aggression and that experiencing psychological aggression was related to symptoms of psychological distress. (Jouriles, Garrido, Rosenfield, & McDonald, 2009)[2]
This approach is often used to assess the validity of new psychological measures. For example, when John Cacioppo and Richard Petty created their Need for Cognition Scale—a measure of the extent to which people like to think and value thinking—they used it to measure the need for cognition for a large sample of college students, along with three other variables: intelligence, socially desirable responding (the tendency to give what one thinks is the “appropriate” response), and dogmatism (Caccioppo & Petty, 1982)[3]. The results of this study are summarized in Table 6.1, which is a correlation matrix showing the correlation (Pearson’s r) between every possible pair of variables in the study. For example, the correlation between the need for cognition and intelligence was +.39, the correlation between intelligence and socially desirable responding was +.02, and so on. (Only half the matrix is filled in because the other half would contain exactly the same information. Also, because the correlation between a variable and itself is always +1.00, these values are replaced with dashes throughout the matrix.) In this case, the overall pattern of correlations was consistent with the researchers’ ideas about how scores on the need for cognition should be related to these other constructs.
Table 6.1 Correlation Matrix Showing Correlations Among the Need for Cognition and Three Other Variables Based on Research by Cacioppo and Petty (1982)
Need for cognition | Intelligence | Social desirability | Dogmatism | |
Need for cognition | — | |||
Intelligence | +.39 | — | ||
Social desirability | +.08 | +.02 | — | |
Dogmatism | −.27 | −.23 | +.03 | — |
Factor Analysis
When researchers study relationships among a large number of conceptually similar variables, they often use a complex statistical technique called factor analysis. In essence, factor analysis organizes the variables into a smaller number of clusters, such that they are strongly correlated within each cluster but weakly correlated between clusters. Each cluster is then interpreted as multiple measures of the same underlying construct. These underlying constructs are also called “factors.” For example, when people perform a wide variety of mental tasks, factor analysis typically organizes them into two main factors—one that researchers interpret as mathematical intelligence (arithmetic, quantitative estimation, spatial reasoning, and so on) and another that they interpret as verbal intelligence (grammar, reading comprehension, vocabulary, and so on). The Big Five personality factors have been identified through factor analyses of people’s scores on a large number of more specific traits. For example, measures of warmth, gregariousness, activity level, and positive emotions tend to be highly correlated with each other and are interpreted as representing the construct of extraversion. As a final example, researchers Peter Rentfrow and Samuel Gosling asked more than 1,700 university students to rate how much they liked 14 different popular genres of music (Rentfrow & Gosling, 2008)[4]. They then submitted these 14 variables to a factor analysis, which identified four distinct factors. The researchers called them Reflective and Complex (blues, jazz, classical, and folk), Intense and Rebellious (rock, alternative, and heavy metal), Upbeat and Conventional (country, soundtrack, religious, pop), and Energetic and Rhythmic (rap/hip-hop, soul/funk, and electronica); see Table 6.2.
Table 6.2 Factor Loadings of the 14 Music Genres on Four Varimax-Rotated Principal Components. Based on Research by Rentfrow and Gosling (2003)
Music-preference dimension | ||||
Genre | Reflective and Complex | Intense and Rebellious | Upbeat and Conventional | Energetic and Rhythmic |
Blues | .85 | .01 | -.09 | .12 |
Jazz | .83 | .04 | .07 | .15 |
Classical | .66 | .14 | .02 | -.13 |
Folk | .64 | .09 | .15 | -.16 |
Rock | .17 | .85 | -.04 | -.07 |
Alternative | .02 | .80 | .13 | .04 |
Heavy metal | .07 | .75 | -.11 | .04 |
Country | -.06 | .05 | .72 | -.03 |
Sound tracks | .01 | .04 | .70 | .17 |
Religious | .23 | -.21 | .64 | -.01 |
Pop | -.20 | .06 | .59 | .45 |
Rap/hip-hop | -.19 | -.12 | .17 | .79 |
Soul/funk | .39 | -.11 | .11 | .69 |
Electronica/dance | -.02 | .15 | -.01 | .60 |
Note. N = 1,704. All factor loadings .40 or larger are in italics; the highest factor loadings for each dimension are listed in boldface type. |
Two additional points about factor analysis are worth making here. One is that factors are not categories. Factor analysis does not tell us that people are either extraverted or conscientious or that they like either “reflective and complex” music or “intense and rebellious” music. Instead, factors are constructs that operate independently of each other. So people who are high in extraversion might be high or low in conscientiousness, and people who like reflective and complex music might or might not also like intense and rebellious music. The second point is that factor analysis reveals only the underlying structure of the variables. It is up to researchers to interpret and label the factors and to explain the origin of that particular factor structure. For example, one reason that extraversion and the other Big Five operate as separate factors is that they appear to be controlled by different genes (Plomin, DeFries, McClean, & McGuffin, 2008)[5].
Exploring Causal Relationships
Another important use of complex correlational research is to explore possible causal relationships among variables. This might seem surprising given the oft-quoted saying that “correlation does not imply causation.” It is true that correlational research cannot unambiguously establish that one variable causes another. Complex correlational research, however, can often be used to rule out other plausible interpretations. The primary way of doing this is through the statistical control of potential third variables. Instead of controlling these variables through random assignment or by holding them constant as in an experiment, the researcher instead measures them and includes them in the statistical analysis called partial correlation. Using this technique, researchers can examine the relationship between two variables, while statistically controlling for one or more potential third variables.
For example, assume a researcher was interested in the relationship between watching violent television shows and aggressive behavior but she was concerned that socioeconomic status (SES) might represent a third variable that is driving this relationship. In this case, she could conduct a study in which she measures the amount of violent television that participants watch in their everyday life, the number of acts of aggression that they have engaged in, and their SES. She could first examine the correlation between violent television viewing and aggression. Let’s say she found a correlation of +.35, which would be considered a moderate sized positive correlation. Next, she could use partial correlation to reexamine this relationship after statistically controlling for SES. This technique would allow her to examine the relationship between the part of violent television viewing that is independent of SES and the part of aggressive behavior that is independent of SES. If she found that the partial correlation between violent television viewing and aggression while controlling for SES was +.34, that would suggest that the relationship between violent television viewing and aggression is largely independent of SES (i.e., SES is not a third variable driving this relationship). On the other hand, if she found that after statistically controlling for SES the correlation between violent television viewing and aggression dropped to +.03, then that would suggest that SES is indeed a third variable that is driving the relationship. If, however, she found that statistically controlling for SES reduced the magnitude of the correlation from +.35 to +.20, then this would suggest that SES accounts for some, but not all, of the relationship between television violence and aggression. It is important to note that while partial correlation provides an important tool for researchers to statistically control for third variables, researchers using this technique are still limited in their ability to arrive at causal conclusions because this technique does not take care of the directionality problem and there may be other third variables driving the relationship that the researcher did not consider and statistically control.
Regression
Once a relationship between two variables has been established, researchers can use that information to make predictions about the value of one variable given the value of another variable. For, instance, once we have established that there is a correlation between IQ and GPA we can use people’s IQ scores to predict their GPA. Thus, while correlation coefficients can be used to describe the strength and direction of relationships between variables, regression is a statistical technique that allows researchers to predict one variable given another. Regression can also be used to describe more complex relationships between more than two variables. Typically the variable that is used to make the prediction is referred to as the predictor variable and the variable that is being predicted is called the outcome variable or criterion variable. This regression equation has the following general form:
Y = b1X1
Y in this formula represents the person’s predicted score on the outcome variable, b1 represents the slope of the line depicting the relationship between two variables (or the regression weight), and X1 represents the person’s score on the predictor variable. You can see that to predict a person’s score on the outcome variable (Y), one simply needs to multiply their score on the predictor variable (X) by the regression weight (b1 )
While simple regression involves using one variable to predict another, multiple regression involves measuring several variables (X1, X2, X3,…Xi), and using them to predict some outcome variable (Y). Multiple regression can also be used to simply describe the relationship between a single outcome variable (Y) and a set of predictor variables (X1, X2, X3,…Xi). The result of a multiple regression analysis is an equation that expresses the outcome variable as an additive combination of the predictor variables. This regression equation has the following general form:
Y = b1X1+ b2X2+ b3X3+ … + biXi
The regression weights (b1, b2, and so on) indicate how large a contribution a predictor variable makes, on average, to the prediction of the outcome variable. Specifically, they indicate how much the outcome variable changes for each one-unit change in the predictor variable.
The advantage of multiple regression is that it can show whether a predictor variable makes a contribution to an outcome variable over and above the contributions made by other predictor variables (i.e., it can be used to show whether a predictor variable is related to an outcome variable after statistically controlling for other predictor variables). As a hypothetical example, imagine that a researcher wants to know how income and health relate to happiness. This is tricky because income and health are themselves related to each other. Thus if people with greater incomes tend to be happier, then perhaps this is only because they tend to be healthier. Likewise, if people who are healthier tend to be happier, perhaps this is only because they tend to make more money. But a multiple regression analysis including both income and health as predictor variables would show whether each one makes a contribution to the prediction of happiness when the other is taken into account (when it is statistically controlled). In other words, multiple regression would allow the researcher to examine whether that part of income that is unrelated to health predicts or relates to happiness as well as whether that part of health that is unrelated to income predicts or relates to happiness. Research like this, by the way, has shown both income and health make extremely small contributions to happiness except in the case of severe poverty or illness (Diener, 2000[6]).
The examples discussed in this section only scratch the surface of how researchers use complex correlational research to explore possible causal relationships among variables. It is important to keep in mind, however, that purely correlational approaches cannot unambiguously establish that one variable causes another. The best they can do is show patterns of relationships that are consistent with some causal interpretations and inconsistent with others.
- Radcliffe, N. M., & Klein, W. M. P. (2002). Dispositional, unrealistic, and comparative optimism: Differential relations with knowledge and processing of risk information and beliefs about personal risk. Personality and Social Psychology Bulletin, 28, 836–846. ↵
- Jouriles, E. N., Garrido, E., Rosenfield, D., & McDonald, R. (2009). Experiences of psychological and physical aggression in adolescent romantic relationships: Links to psychological distress. Child Abuse & Neglect, 33(7), 451–460. ↵
- Cacioppo, J. T., & Petty, R. E. (1982). The need for cognition. Journal of Personality and Social Psychology, 42, 116–131. ↵
- Rentfrow, P. J., & Gosling, S. D. (2008). The do re mi’s of everyday life: The structure and personality correlates of music preferences. Journal of Personality and Social Psychology, 84, 1236–1256. ↵
- Plomin, R., DeFries, J. C., McClearn, G. E., & McGuffin, P. (2008). Behavioral genetics (5th ed.). New York, NY: Worth. ↵
- Diener, E. (2000). Subjective well-being: The science of happiness, and a proposal for a national index. American Psychologist, 55, 34–43. ↵
A complex statistical technique in which researchers study relationships among a large number of conceptually similar variables.
Controlling potential third variables to rule out other plausible interpretations.
Learning Objectives
- Describe a micro-, meso-, and macro-level approaches to research
- Provide examples of micro-, meso-, and macro-level studies
In Chapter 1, we reviewed the micro, meso, and macro framework that social workers use to understand the world. As you’ll recall, micro-level research studies individuals and one-on-one interactions, meso-level research studies groups, and macro-level research studies institutions and policies. Let’s take a closer look at some specific examples of social work research to better understand each of the three levels of inquiry described previously. Some topics are best suited to be examined at one specific level, while other topics can be studied at each of the three different levels. The particular level of inquiry might shape a social worker’s questions about the topic, or a social scientist might view the topic from different angles depending on the level of inquiry being employed.
First, let’s consider some examples of different topics that are best suited to a particular level of inquiry. Work by Stephen Marks offers an excellent example of research at the micro-level. In one study, Marks and Shelley MacDermid (1996) [1] draw from prior micro-level theories to empirically study how people balance their roles and identities. In this study, the researchers found that people who experience balance across their multiple roles and activities report lower levels of depression and higher levels of self-esteem and well-being than their less-balanced counterparts. In another study, Marks and colleagues examined the conditions under which husbands and wives feel the most balance across their many roles. They found that different factors are important for different genders. For women, having more paid work hours and more couple time were among the most important factors. For men, having leisure time with their nuclear families was important, and role balance decreased as work hours increased (Marks, Huston, Johnson, & MacDermid, 2001). [2] Both of these studies fall within the category of micro-level analysis.
At the meso-level, social scientists tend to study the experiences of groups and the interactions between groups. In a recent book based on their research with Somali immigrants, Kim Huisman and colleagues (Huisman, Hough, Langellier, & Toner, 2011) [3] examine the interactions between Somalis and Americans in Maine. These researchers found that stereotypes about refugees being unable or unwilling to assimilate and being overly dependent on local social systems are unsubstantiated. In a much different study of group-level interactions, Michael Messner (2009) [4] conducted research on children’s sports leagues. Messner studied interactions among parent volunteers, among youth participants, and between league organizers and parents. They found that gender boundaries and hierarchies are perpetuated by the adults who run such leagues. While these two studies are very different in their specific points of focus, they both have a meso-level focus.
Social workers who conduct macro-level research study interactions at the broadest level, such as interactions between and across nations, states, or cultural systems. One example of macro-level research can be seen in a recent article by David Frank and colleagues (Frank, Camp, & Boutcher, 2010). [5] These researchers examined worldwide changes over time in laws regulating sex. By comparing laws across a number of countries over a period of many years (1945–2005), Frank learned that laws regulating rape, adultery, sodomy, and child sexual abuse shifted in focus from protecting larger entities, such as families, to protecting individuals. In another macro-level study, Leah Ruppanner (2010) [6] studied how national levels of gender equality in 25 different countries affect couples’ divisions of housework. Ruppanner found that as women’s parliamentary representation increases, so does men’s participation in housework.
While it is true that some topics lend themselves to a particular level of inquiry, there are many topics that could be studied from any of the three levels. The choice depends on the specific interest of the researcher, the approach they would like to take and the sorts of questions they want to be able to answer about the topic.
Let’s look at an example. Gang activity has been a topic of interest to social workers for many years and has been studied from each of the levels of inquiry described here. At the micro-level, social workers might study the inner workings of a specific gang, communication styles, and what everyday life is like for gang members. Though not written by a social worker, one example of a micro-level analysis of gang activity can be found in Sanyika Shakur’s 1993 autobiography, Monster. [7] In his book, Shakur describes his former day-to-day life as a member of the Crips in South-Central Los Angeles. Shakur’s recounting of his experiences highlights micro-level interactions between himself, fellow Crips members, and other gangs.
At the meso-level, social workers are likely to examine interactions between gangs or perhaps how different branches of the same gang vary from one area to the next. At the macro-level, we could compare the impact of gang activity across communities or examine the national economic impact of gang activity. Excellent examples of gang research at all three levels of analysis can be found in the Journal of Gang Research published by the National Gang Crime Research Center (NGCRC). [8] Sudhir Venkatesh’s (2008) study, Gang Leader for a Day, [9] is an example of research on gangs that utilizes all three levels of analysis. Venkatesh conducted participant observation with a gang in Chicago. He learned about the everyday lives of gang members (micro) and how the gang he studied interacted with and fit within the landscape of other gang “franchises” (meso). In addition, Venkatesh described the impact of the gang on the broader community and economy (macro).
Key Takeaways
- Social work research can occur at any of the following three analytical levels: micro, meso, or macro.
- Some topics lend themselves to one particular analytical level, while others could be studied from any, or all, of the three levels of analysis.
The last chapter oriented you to the theories relevant to your topic area; the macro, meso, or micro levels of analysis; and the assumptions or paradigms of research. This chapter will use these elements to help you conceptualize and design your research project. You will decide the purpose of your research, whether you will use quantitative or qualitative methods, and how you will establish causality. You’ll also learn how and why researchers use both qualitative and quantitative methods in the same study.
Chapter Outline
- 7.1 Types of research
- 7.2 Causal relationships
- 7.3 Unit of analysis and unit of observation
- 7.4 Mixed methods
Content Advisory
This chapter discusses or mentions the following topics: child neglect and abuse, sexual harassment, the criminal justice system, homelessness, sexual and domestic violence, depression, and substance abuse.
Learning Objectives
- Describe the inductive approach to research, and provide examples of inductive research
- Describe the deductive approach to research, and provide examples of deductive research
- Describe the ways that inductive and deductive approaches may be complementary
Theory structures and informs social work research. Conversely, social work research structures and informs theory. Students become aware of the reciprocal relationship between theory and research when they consider the relationships between the two in inductive and deductive approaches. In both cases, theory is crucial but the relationship between theory and research differs for each approach.
Inductive and deductive approaches to research are quite different, but they can also be complementary. Let’s start by looking at each one and how they differ from one another. Then we’ll move on to thinking about how they complement one another.
Inductive approaches and some examples
When a researcher utilizes an inductive approach, they begin by collecting data that is relevant to their topic of interest. Once a substantial amount of data have been collected, the researcher will take a break from data collection to step back and get a bird’s eye view of their data. At this stage, the researcher looks for patterns in the data, working to develop a theory that could explain those patterns. Thus, when researchers take an inductive approach, they start with a set of observations and then they move from those particular experiences to a more general set of propositions about those experiences. In other words, they move from data to theory, or from the specific to the general. Figure 6.1 outlines the steps involved with an inductive approach to research.
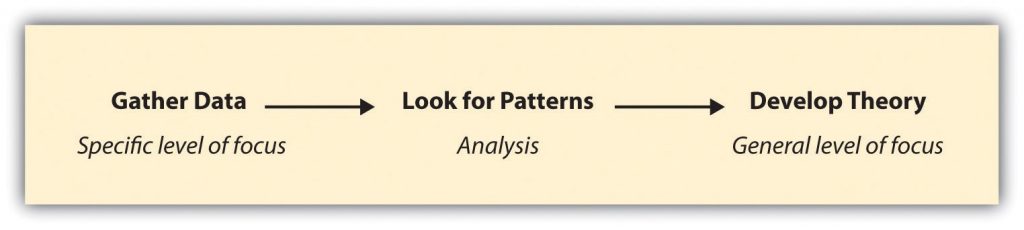
There are many good examples of inductive research, but we’ll look at just a few here. One fascinating study in which the researchers took an inductive approach is Katherine Allen, Christine Kaestle, and Abbie Goldberg’s (2011) study [10] of how boys and young men learn about menstruation. To understand this process, Allen and her colleagues analyzed the written narratives of 23 young men in which the men described how they learned about menstruation, what they thought of it when they first learned about it, and what they think of it now. By looking for patterns across all 23 men’s narratives, the researchers were able to develop a general theory of how boys and young men learn about this aspect of girls’ and women’s biology. They conclude that sisters play an important role in boys’ early understanding of menstruation, that menstruation makes boys feel somewhat separated from girls, and that as they enter young adulthood and form romantic relationships, young men develop more mature attitudes about menstruation. Note how this study began with the data—men’s narratives of learning about menstruation—and tried to develop a theory.
In another inductive study, Kristin Ferguson and colleagues (Ferguson, Kim, & McCoy, 2011) [11] analyzed empirical data to better understand how best to meet the needs of young people who are experiencing homelessness. The authors analyzed data from focus groups with 20 young people at a homeless shelter. From these data they developed a set of recommendations for those interested in applied interventions that serve youth that are experiencing homelessness. The researchers also developed hypotheses for people who might wish to conduct further investigation of the topic. Though Ferguson and her colleagues did not test the hypotheses that they developed from their analysis, their study ends where most deductive investigations begin: with a theory and a hypothesis derived from that theory.
Deductive approaches and some examples
Researchers taking a deductive approach will start with a compelling social theory and then test its implications with data. In other words, they utilize the same steps as inductive research, but they will reverse the order, moving from general to more specific levels. Deductive research approach is most associated with scientific investigation. The researcher studies what others have done, reads existing theories of whatever phenomenon they are studying, and then tests hypotheses that emerge from those theories. Figure 6.2 outlines the steps involved with a deductive approach to research.
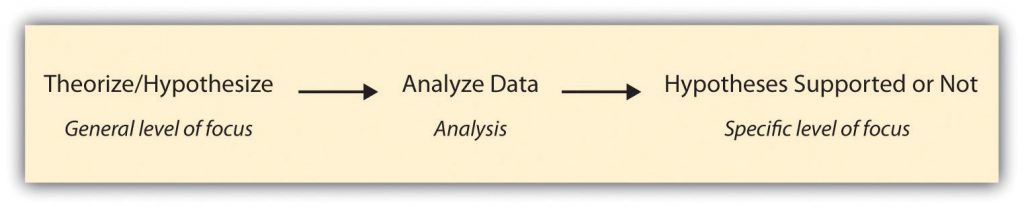
Although not all social science researchers utilize a deductive approach, there are some excellent, recent examples of deductive research. We’ll take a look at a couple of those next.
In a study of US law enforcement responses to hate crimes, Ryan King and colleagues (King, Messner, & Baller, 2009) [12] hypothesized that law enforcement’s response would be less vigorous in areas of the country that had a stronger history of racial violence. The authors developed their hypothesis from their reading of prior research and theories on the topic. They tested the hypothesis by analyzing data on states’ lynching histories and hate crime responses. Overall, the authors found support for their hypothesis. One might associate this research with critical theory.
In another recent deductive study, Melissa Milkie and Catharine Warner (2011) [13] studied the effects of different classroom environments on first graders’ mental health. Based on prior research and theory, Milkie and Warner hypothesized that negative classroom features, such as a lack of basic supplies and even heat, would be associated with emotional and behavioral problems in children. One might associate this research with systems theory. The researchers found support for their hypothesis, demonstrating that policymakers should be more attentive to the mental health outcomes of children’s school experiences, just as they track academic outcomes (American Sociological Association, 2011). [14]
Complementary approaches
While inductive and deductive approaches to research seem quite different, they can be rather complementary. In some cases, researchers will plan for their study to include multiple components, one inductive and the other deductive. In other cases, a researcher might begin their study planning to utilize only one approach but then discover along the way that the other approach is needed to help illuminate findings. Here is an example of each such case.
The original author of the textbook from which this textbook is adapted, Dr. Amy Blackstone, relates a story about her collaborative research on sexual harassment.
We began the study knowing that we would like to take both a deductive and an inductive approach in our work. We therefore administered a quantitative survey, the responses to which we could analyze in order to test hypotheses, and also conducted qualitative interviews with a number of the survey participants. The survey data were well suited to a deductive approach; we could analyze those data to test hypotheses that were generated based on theories of harassment. The interview data were well suited to an inductive approach; we looked for patterns across the interviews and then tried to make sense of those patterns by theorizing about them.
For one paper (Uggen & Blackstone, 2004), [15] we began with a prominent feminist theory of the sexual harassment of adult women and developed a set of hypotheses outlining how we expected the theory to apply in the case of younger women’s and men’s harassment experiences. We then tested our hypotheses by analyzing the survey data. In general, we found support for the theory that posited that the current gender system, in which heteronormative men wield the most power in the workplace, explained workplace sexual harassment—not just of adult women but of younger women and men as well. In a more recent paper (Blackstone, Houle, & Uggen, 2006), [16] we did not hypothesize about what we might find but instead inductively analyzed interview data, looking for patterns that might tell us something about how or whether workers’ perceptions of harassment change as they age and gain workplace experience. From this analysis, we determined that workers’ perceptions of harassment did indeed shift as they gained experience and that their later definitions of harassment were more stringent than those they held during adolescence. Overall, our desire to understand young workers’ harassment experiences fully—in terms of their objective workplace experiences, their perceptions of those experiences, and their stories of their experiences—led us to adopt both deductive and inductive approaches in the work. (Blackstone, n.d., p. 21)
Researchers may not set out to employ both approaches in their work, but sometimes their use of one approach leads them to the other. One such example is described eloquently in Russell Schutt’s Investigating the Social World (2006). [17] As Schutt describes, researchers Lawrence Sherman and Richard Berk (1984) [18] conducted an experiment to test two competing theories of the effects of punishment on deterring deviance (in this case, domestic violence). Specifically, Sherman and Berk hypothesized that deterrence theory would provide a better explanation of the effects of arresting accused batterers than labeling theory. Deterrence theory predicts that arresting an accused spouse batterer will reduce future incidents of violence. Conversely, labeling theory predicts that arresting accused spouse batterers will increase future incidents. Figure 6.3 summarizes the two competing theories and the predictions that Sherman and Berk set out to test.
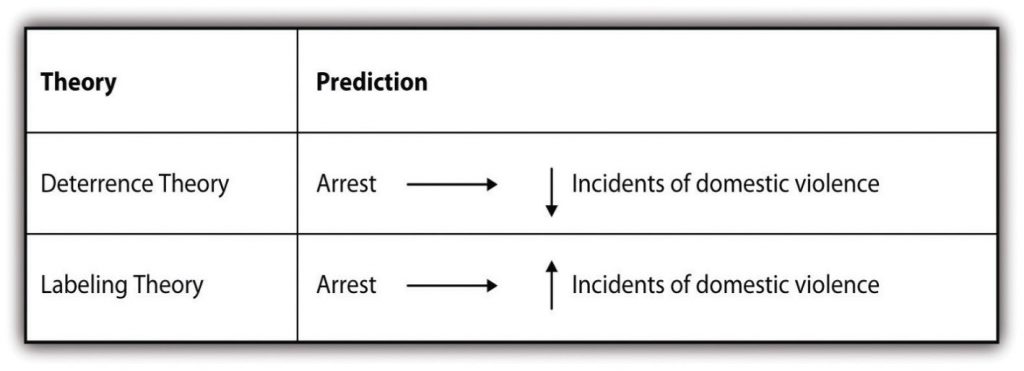
After conducting an experiment with the help of local police, Sherman and Berk found, that arrest did deter future incidents of violence, thus supporting their hypothesis that deterrence theory would better predict the effect of arrest. After conducting this research, they and other researchers went on to conduct similar experiments [19] in six additional cities (Berk, Campbell, Klap, & Western, 1992; Pate & Hamilton, 1992; Sherman & Smith, 1992). [20] The follow-up studies yielded mixed results. In some cases, arrest deterred future incidents of violence while in other cases, arrest did not. These results left the researchers with new data that they needed to explain, so they utilized an inductive approach to make sense of their latest empirical observations. The new studies revealed that arrest has a deterrent effect on individuals that are married and employed, while arrest may encourage future battering offenses in individuals that are unmarried and unemployed. Researchers thus turned to control theory to explain their observations, as it predicts that stakes in conformity are developed through social ties like marriage and employment.
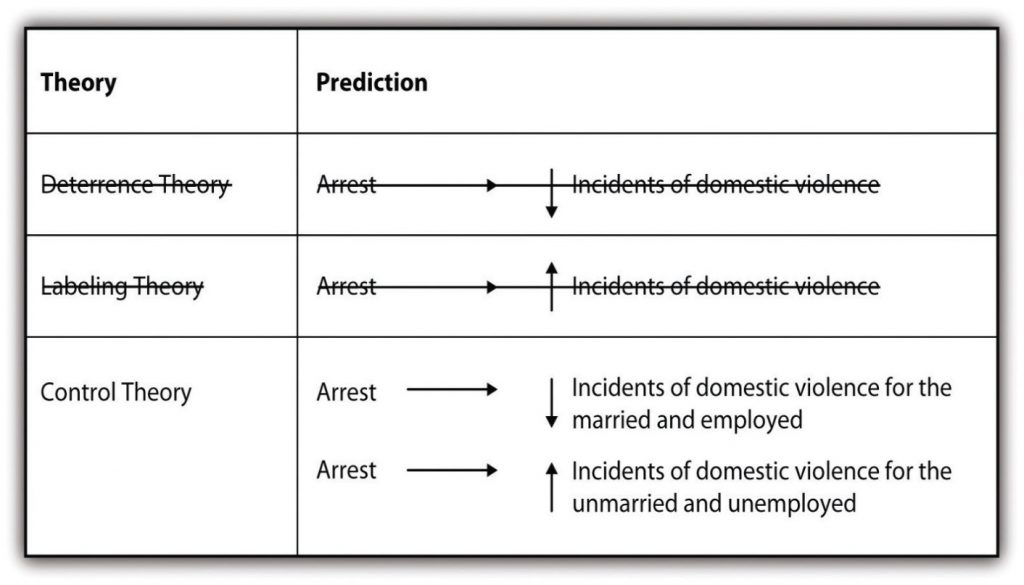
Sherman and Berk's research and the associated follow-up studies demonstrate that researchers can start with a deductive approach and move to inductive approach when confronted with new data that must be explained.
Key Takeaways
- The inductive approach begins with a set of empirical observations, seeking patterns in those observations, and then theorizing about those patterns.
- The deductive approach begins with a theory, developing hypotheses from that theory, and then collecting and analyzing data to test those hypotheses.
- Inductive and deductive approaches to research can be employed together for a more complete understanding of the topic that a researcher is studying.
- Though researchers don’t always set out to use both inductive and deductive strategies in their work, they sometimes find that new questions arise in the course of an investigation that can best be answered by employing both approaches.
Glossary
Deductive approach- when a researcher studies what others have done, reads existing theories of whatever phenomenon they are studying, and then tests hypotheses that emerge from those theories
Inductive approach- when a researcher starts with a set of observations and then moves from particular experiences to a more general set of propositions about those experiences
In this chapter, we’ll explore the connections between paradigms, social theories, and social scientific research methods. We’ll also consider how our analytic, paradigmatic, and theoretical perspective might shape or be shaped by our methodological choices. In short, we’ll discuss the relationship between theory and research methods.
Chapter Outline
- 6.1 Micro, meso, and macro approaches
- 6.2 Paradigms, theories, and how they shape a researcher’s approach
- 6.3 Inductive and deductive reasoning
Content Advisory
This chapter discusses or mentions the following topics: laws regulating rape, sodomy, and child sexual abuse; gang communication styles; racism, policing, and lynching; domestic violence and sexual harassment; and substance abuse.
Involves measuring several variables (X1, X2, X3,…Xi), and using them to predict some outcome variable (Y).