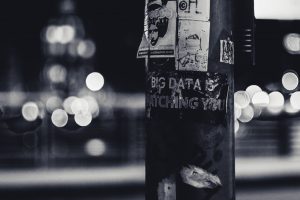
There is a contemporary interest in the use of “big data” and “analytics” to improve the quality and productivity of all sectors, including education. Big data refers to “datasets whose size is beyond the ability of typical database software tools to capture, store, manage, and analyze” (Manyika et al., 2012, p. 1). For example, everyday an estimated 3.8 billion people use the Internet and produce a staggering amount of information— an estimated 656 million tweets, 4 million hours of content uploaded to YouTube, 67, 305,600 Instagram posts, and 4.3 billion Facebook messages, and 5.2 billion Google searches (Schultz, 2017). The popular uses of the term “analytics” indicate this societal interest in data.
Colleges and universities traditionally collect a wide range of digital data or “big data” from students (Picciano, 2012), such as information on demographics, application data, transcripts, learning management systems, library accesses, surveys, and meals (de Freitas et al, 2015; Lester, Klein, Rangwala, Johri, 2017). More recently, higher education institutions express the focus on data in using the term “learning analytics,” which refers to “the measurement, collection, analysis and reporting of data about learners and their contexts, for the purposes of understanding and optimising learning and the environments in which it occurs” (Long & Siemens, 2011). Although learning analytics is still at relatively early stage of development in the educational sector, several institutions are investigating or already using learning analytics for predicting student retention (Arnold & Pistilli, 2012; de Freitas et al, 2015; Roberts, Howell, Seaman, & Gibson, 2016).
Learning analytics has been identified as a trend by the New Media Consortium 4 times in the past 6 years, and is related to the “Growing focus on measuring learning,” as a key mid-term trend driving educational technology adoption in higher education the next three to five years (NMC, 2017). This trend highlights the interest in assessment and the analytics and visualization tools that can be used to analyze data from online learning, mobile learning, and learning management systems to reveal how student behaviours in these learning environments can contribute to their learning progression.
In Canada, a recent national survey of 203 public postsecondary institutions reported that 90% of the universities and 80% of colleges outside Québec offered some form of distance education using the Internet and Learning Management Systems (LMS) as the main form of delivery technology (Bates et al. 2017). As online learning opportunities continue to grow, so do the opportunities to analyze and leverage the learning data that students automatically generate and that learning management systems automatically collect. Learning analytics is clearly a technology trend that will impact educators and learners as more and more higher education institutions begin to explore learning analytics for teaching and learning at scale.
From Research to Practice
In the last 7 years, the field of learning analytics has grown quickly, with researchers from various disciplines such as the Learning Sciences, Computer Science, Human-Computer Interaction, Psychology, and Behavioral Sciences contributing their perspectives on how to understand and optimize learning processes and environments (Ochoa, Hershkovitz, Wise, & Knight, 2017). The Society for Learning Analytics Research (SoLAR) holds international annual Learning Analytics and Knowledge (LAK) conferences and Learning Analytic Summer Institutes (LASI), there is an open-access, peer-reviewed journal devoted to learning analytics, Journal of Learning Analytics (JLA) that has published two issues of leading-edge research articles a year since 2014.
Learning analytics can be considered a form of educational data mining in which predictive modeling can reveal patterns of success or failure to enable interventions (Arroway, Morgan, O’Keefe, & Yanosky, 2016). Learning analytics promises to “expand the capacity and ability of organizations to make sense of complex environments” (Norris & Baer, 2013, p. 13) and improve pedagogy, course, design, student retention, and decision making (Ali, Hatala, Gasevic, & Jovanovic, 2012; Lester et al., 2017; Macfadyen & Dawson, 2012). Indeed, there are a few prominent examples of large scale, systematic implementations of learning analytics (Sclater, Peasgood, & Mullan, 2016), and learning analytic tools are increasingly available as part of learning management systems (Wilson et al., 2017).
However, somewhat less is known about how educators and learners in higher education can use learning analytics in their practices to benefit from advances being made in learning analytic research and development. Many learning analytics publications are replete with complex mathematical formulae or computer code, making them impenetrable to a general audience (Sclater, 2017). For instance, West, Heath, and Huijser (2016) studied the Australian context by surveying academic staff and found that “teaching academics in particular were mostly unaware of the initiatives taking place in their own institutions…most academics in the survey very rarely had discussions with anyone around learning analytics “ (np).
Wilson et al. (2017) caution that educators are the ones who will need to use and interpret learning analytics, and argue that analytics that relies the digital traces left by participants in online learning as proxies for learning tends towards a narrowly behaviorist evaluation of learning processes. They problematize the analytics and algorithms on which automated interventions are based, and encourages exploration and reflection on the limitations of learning analytics.
The aim of this practical guide is to introduce learning analytics to educators in higher education. To this end, we define learning analytics, provide some background to how learning analytics work, explore successful learning analytics implementations, and present findings from an exploratory study of perceptions of the users of learning analytics—educators, students, and advisors—of common metrics and visualizations from learning management system analytic tools at an Ontario university to provide a practical guide to learning analytics.