9.3: Contract Performance Quality
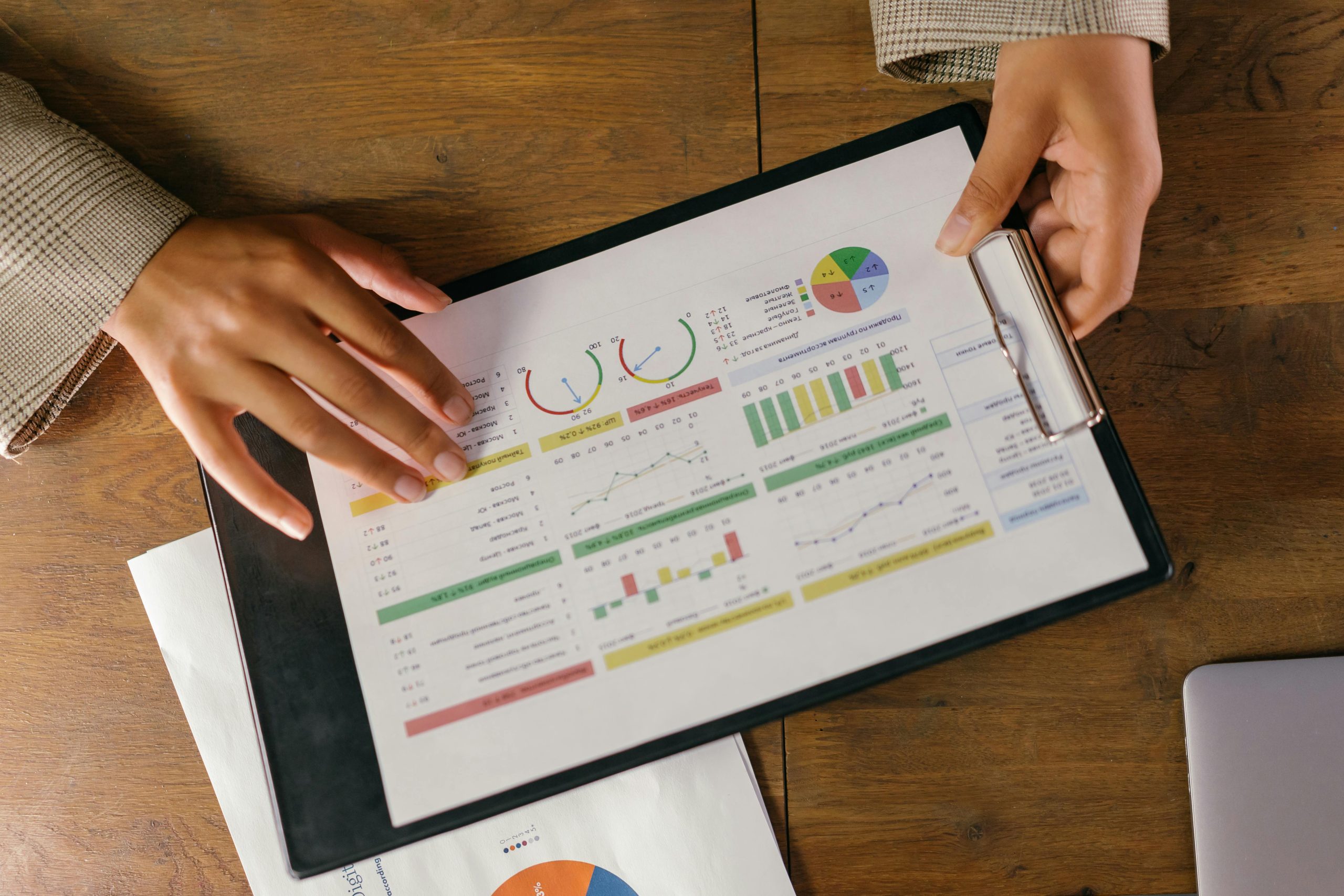
Quality in PM
It’s not enough to ensure a project gets done on time and under budget. You must make the right product to suit your stakeholders’ needs. Quality means ensuring you build what you said you would and do it as efficiently as possible. And that means trying not to make too many mistakes and always keeping your project working toward the goal of creating the right product.
Everybody “knows” what quality is. However, how the word is used in everyday life is slightly different from how it is used in project management. Just like the triple constraint (scope, cost, and schedule), you manage the quality of a project by setting goals and taking measurements. That’s why you must understand the quality levels your stakeholders believe are acceptable and ensure that your project meets those targets, just like it needs to meet their budget and schedule goals.
Customer satisfaction is about ensuring taxpayers are happy with what they get. When the team gathers requirements for the specification, they try to write down all of the things that the customers want in the product so that they know how to make them happy. Some requirements can be left unstated. Those are the ones that are implied by the customer’s explicit needs. For example, some requirements are just common sense (e.g., a product people hold can’t be made from toxic chemicals that may kill them). It might not be stated, but it’s definitely a requirement.
“Fitness to use” is about making sure that the product you build has the best design possible to fit the customer’s needs. Which would you choose: a product that is beautifully designed, well constructed, solidly built, and all-around pleasant to look at but does not do what you need or a product that does what you want despite being ugly and hard to use? You’ll always choose the product that fits your needs, even if it’s seriously limited. That’s why it’s important that the product does what it is supposed to do and does it well. For example, you could pound in a nail with a screwdriver, but a hammer is a better fit for the job.
Conformance to requirements is the core of both customer satisfaction and fitness to use and is a measure of how well your product does what you intend. Above all, your product must do what you wrote down in your requirements document. Your requirements should consider what will satisfy your customer and the best design possible for the job. That means conforming to both stated and implied requirements.
Ultimately, your product’s quality is judged by whether you built what you said you would build.
Quality planning focuses on taking all of the information available to you at the beginning of the project and figuring out how you will measure quality and prevent defects. Your company should have a quality policy that states how quality is measured across the organization. You should ensure your project follows the company policy and any government rules or regulations on planning quality for your project.
You need to plan which activities you will use to measure the quality of the project’s product. And you’ll need to think about the cost of all the quality-related activities you want to do. Then, you’ll need to set some guidelines for what you will measure against. Finally, you’ll need to design the tests you will run when the product is ready to be tested.
Quality and Grade
The International Organization for Standardization (ISO) defines quality as “the degree to which a set of inherent characteristics fulfill requirements.” The requirements of a product or process can be categorized or given a grade that will provide a basis for comparison. The quality is determined by how well something meets its grade requirements.
For most people, quality also implies good value—getting your money’s worth. For example, even low-grade products should still work as expected, be safe to use, and last a reasonable amount of time. Consider the following examples.
Example: Quality of Gasoline Grades
Petroleum refiners provide gasoline in several grades based on the octane rating because higher octane ratings are suitable for higher compression engines. Gasoline must not be contaminated with dirt or water, and the actual performance of the fuel must be close to its octane rating. A shipment of low-grade gasoline graded as 87 octane, free of water or other contaminants, would be of high quality, while a shipment of high-grade 93 octane gas contaminated with dirt would be of low quality.
Statistics
Determining how well products meet grade requirements is done by taking measurements and then interpreting those measurements. Statistics—the mathematical interpretation of numerical data—are useful when interpreting large numbers of measurements and are used to determine how well the product meets a specification when the same product is made repeatedly. Measurements made on product samples must be within control limits—the upper and lower extremes of allowable variation—and it is up to management to design a process that will consistently produce products between those limits.
Instructional designers often use statistics to determine the quality of their course designs. Student assessments are one way by which instructional designers can tell whether learning occurs within the control limits.
Example: Setting Control Limits
A petroleum refinery produces large quantities of fuel in several grades. Samples of the fuels are extracted and measured at regular intervals. If a fuel is supposed to have an 87-octane performance, samples of the fuel should produce test results close to that value. Many of the samples will have scores that are different from 87. The differences are due to random factors that are difficult or expensive to control. Most samples should be close to the 87 rating, and none should be too far off. The manufacturer has grades of 85 and 89, so they decided that none of the samples of the 87-octane fuel should be less than 86 or higher than 88.
Suppose a process is designed to produce a product of a certain size or other measured characteristic. In that case, it is impossible to control all the small factors that can cause the product to differ slightly from the desired measurement. Some of these factors will produce products with larger than desired measurements, and some will have the opposite effect. If several random factors affect the process, they tend to offset each other, and the most common results are near the middle of the range; this phenomenon is called the central limit theorem.
Suppose the range of possible measurement values is divided equally into subdivisions called bins. In that case, the measurements can be sorted, and the number of measurements that fall into each bin can be counted. The result is a frequency distribution that shows how many measurements fall into each bin. If the effects causing the differences are random and tend to offset each other, the frequency distribution is called a normal distribution, which resembles the shape of a bell with edges that flare out. The edges of a theoretical normal distribution curve get very close to zero but do not reach zero.
Example: Normal Distribution
A refinery’s quality control manager measures many samples of 87 octane gasoline, sorts the measurements by their octane rating into bins that are 0.1 octane wide, and then counts the number of measurements in each bin. Then, they create a frequency distribution chart of the data, as shown in Exhibit 9.4.
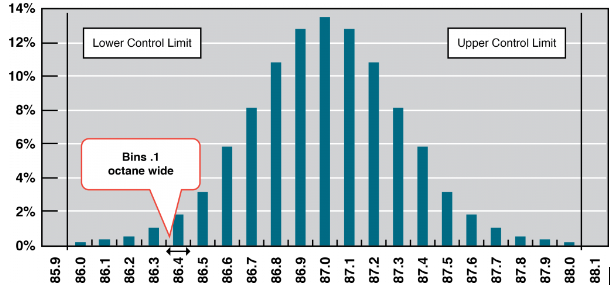
It is common to take samples—randomly selected subsets from the total population—and measure and compare their qualities since measuring the entire population would be cumbersome, if not impossible. Suppose the sample measurements are distributed equally above and below the centre of the distribution as they are in Exhibit 9.4. In that case, the average of those measurements is also the centre value that is called the mean and is represented in formulas by the lowercase Greek letter μ (pronounced mu). The difference in the measurements from the central value is called the sample standard deviation or the standard deviation.
The first step in calculating the standard deviation is subtracting each measurement from the central value (mean) and then squaring that difference. (Recall from your mathematics courses that squaring a number means multiplying it by itself, and the result is always positive.) The next step is to sum these squared values and divide by the number of values minus one. The last step is to take the square root. The result can be thought of as an average difference. (If you had used the usual method of taking an average, the positive and negative numbers would have summed to zero.) Mathematicians represent the standard deviation with the lowercase Greek letter ∑ (pronounced sigma). If all the elements of a group are measured instead of just a sample, it is called the population’s standard deviation. In the second step, the sum of the squared values is divided by the total number of values.
Exhibit 9.4 shows that the most common measurements of octane rating are close to 87 and that the other measurements are distributed equally above and below 87. The shape of the distribution chart supports the central limit theorem’s assumption that the factors affecting the octane rating are random and tend to offset each other, which is indicated by the symmetric shape. This distribution is a classic example of a normal distribution. The quality control manager noticed that none of the measurements were above 88 or below 86, so they were within control limits. She concluded that the process was working satisfactorily.
Example: Standard Deviation of Gasoline Samples
The refinery’s quality control manager uses the standard deviation function in their spreadsheet program to find the standard deviation of the sample measurements and finds that for their data, the standard deviation is 0.3 octane. They mark the range on the frequency distribution chart to show the values that fall within one sigma (standard deviation) on either side of the mean (Exhibit 9.5).
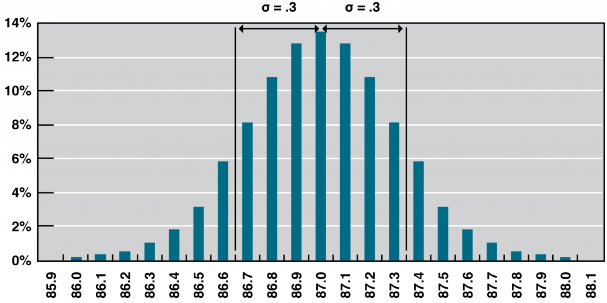
For normal distributions, about 68.3% of the measurements fall within one standard deviation on either side of the mean. This is a useful rule of thumb for analyzing some types of data. Suppose the variation between measurements is caused by random factors that result in a normal distribution, and someone tells you the mean and the standard deviation. In that case, you know that a little over two-thirds of the measurements are within a standard deviation on either side of the mean. Because of the shape of the curve, the number of measurements within two standard deviations is 95.4%, and the number of measurements within three standard deviations is 99.7%.
For example, if someone said the average (mean) height for adult men in the United States is 178 cm (70 inches), and the standard deviation is about 8 cm (3 inches), you would know that 68% of the men in the United States are between 170 cm (67 inches) and 186 cm (73 inches) in height. You would also know that about 95% of adult men in the United States are between 162 cm (64 inches) and 194 cm (76 inches) tall and that almost all of them (99.7%) are between 154 cm (61 inches) and 202 cm (79 inches) tall. These figures are referred to as the 68-95-99.7 rule.
Quality Planning
High quality is achieved by planning for it rather than by reacting to problems after they are identified. Standards are chosen, and processes are put in place to achieve those standards.
Measurement Terminology
During the execution phase of the project, services and products are sampled and measured to determine if the quality is within control limits for the requirements and to analyze causes for variations. A separate quality control group often does this evaluation, and knowledge of a few process measurement terms is necessary to understand their reports. Several of these terms are similar, and knowing the distinction between them is valuable.
The quality plan specifies the control limits of the product or process; the size of the range between those limits is the tolerance. Tolerances are often written as the mean value, plus or minus the tolerance. The plus and minus signs are written together, ±.
Example: Tolerance in Gasoline Production
The petroleum refinery chose to set its control limits for 87-octane gasoline at 86 and 88-octane. The tolerance is 87 ± 1. Tools are selected that can measure the samples closely enough to determine if the measurements are within control limits and if they are showing a trend. Each measurement tool has its own tolerances.
The choice of tolerance directly affects the cost of quality (COQ). In general, producing and measuring products with small tolerances costs more. The costs associated with making products with small tolerances for variation can be very high and not proportional to the gains. For example, suppose the cost of evaluating each screen as it is created in an online tutorial is greater than delivering the product and fixing any issues after the fact. In that case, the COQ may be too high, and the instructional designer will tolerate more defects in the design.
Defining and Meeting Client Expectations
Clients provide specifications for the project that must be met for the project to be successful. Recall that meeting project specifications is one definition of project success. Clients often have expectations that are more difficult to capture in a written specification. For example, one client will want to be invited to every meeting of the project and will then select the ones that seem most relevant. Another client will want to be invited only to project meetings that need client input. Inviting this client to every meeting will cause unnecessary frustration. Listening to the client and developing an understanding of the expectations that are not easily captured in specifications is important to meeting those expectations.
Project surveys can capture how the client perceives the project performance and provide the project team with useful data for meeting client expectations. Suppose the results of the surveys indicate that the client is not pleased with some aspect of the project. In that case, the project team can explore the reasons for this perception with the client and develop recovery plans. The survey can also help define what is going well and what needs improvement.
Planning for quality is part of the initial planning process. The early scope, budget, and schedule estimates are used to identify processes, services, or products where the expected grade and quality should be specified. Risk analysis is used to determine which risks to the project could affect quality.
Planning and Control Techniques
Several different tools and techniques are available for planning and controlling the quality of a project. The extent to which these tools are used is determined by the project complexity and the client’s quality management program. The following represents the quality planning tools available to the project manager.
Cost-benefit analysis is looking at how much your quality activities will cost versus how much you will gain from doing them. The costs are easy to measure; the effort and resources required to do them are like any other task on your schedule. Since quality activities don’t actually produce a product, it is sometimes harder for people to measure the benefit. The main benefits are less reworking, higher productivity and efficiency, and more satisfaction from both the team and the customer.
Benchmarking means using the results of quality planning on other projects to set goals for your own. You might find that the last project in your company had 20% fewer defects than the one before it. You should want to learn from a project like that and put into practice any of the ideas they used to make such a great improvement. Benchmarks can give you some reference points for judging your project before starting the work.
Design of Experiments is the list of all the tests you will run on your product. It might list all the test procedures you’ll do, the approaches you’ll take, and even the tests themselves. (In the software world, this is called test planning.)
Cost of Quality is what you get when you add up the cost of all the prevention and inspection activities you will do on your project. It doesn’t just include the testing. It includes any time spent writing standards, reviewing documents, meeting to analyze the root causes of defects, and reworking to fix the defects once the team finds them: in other words, absolutely everything you do to ensure quality on the project. Cost of quality can be a good number to check to determine whether your project is doing well or having trouble. Say your company tracks the cost of quality on all of its projects; then you could tell if you are spending more or less than has been spent on other projects to get your project up to quality standards.
Control Charts can be used to define acceptable limits. If some of the functions of a project are repetitive, statistical process controls can be used to identify trends and keep the processes within control limits. Part of the planning for controlling the quality of repetitive processes is to determine what the control limits are and how the process will be sampled.
Cause-and-effect diagrams can help in discovering problems. When control charts indicate an assignable cause for a variation, it is not always easy to identify the cause of a problem. Discussions intended to discover the cause can be facilitated using a cause-and-effect or fishbone diagram where participants are encouraged to identify possible causes of a defect.
Real Cases in Public Procurement: Learning from Experience
Oshawa (City) v. Brennan Paving Company Limited
Issue: Failure to comply with the conditions of the contract.
Background: Brennan Paving was contracted by the City of Oshawa to construct a street. Payment for materials was to be based on the weight of materials, and the engineer’s certificate was a condition that had to precede payment. An engineer was contracted for the construction project. One of their duties was to certify payment certificates. Brennan Paving complied with the provisions of the contract. However, the engineer refused to certify the project using the materials by weight methodology and arrived at the amounts to be paid by his own calculation methods.
Outcome: The Supreme Court ruled that the engineer’s refusal to verify the work as required by the contract meant he had relinquished his role. Consequently, the City of Oshawa was found to be in breach of contract. The court ruled in favour of Brennan Paving, releasing them from the requirement for the final verification and certificate.
The City of Oshawa appealed this decision. Its appeal was dismissed, but the verdict was altered slightly to deduct the value of excess asphalt supplied.
Discussion Questions
- If the contract manager had been involved earlier, would things have ended up in court?
- To what extent are parties bound by certificates that the contract does not clearly express?
- Why is it so important to have clearly defined expectations?
Sources: Based on information from CanLII. (1954). Oshawa (City) v. Brennan Paving Co., 1954 CanLII 20 (SCC), SCR 76. Retrieved from CanLII.
Checkpoint 9.3
Image Descriptions:
Exhibit 9.4: The image is a histogram representing the frequency distribution of octane values. The x-axis shows octane values ranging from 85.9 to 88.1, in increments of 0.1, while the y-axis displays the percentage frequency, ranging from 0% to 14%. Each bar represents a 0.1 octane range, and the height of each bar indicates the percentage of occurrences for that range. The distribution shows a peak frequency of about 13% between octane values 86.9 and 87.0. Two control limits are marked: the Lower Control Limit on the left and the Upper Control Limit on the right, indicating the acceptable range of octane values. Bars that lie beyond these limits suggest outliers or values potentially out of specification. A label is pointing to an interval on the x-axis highlighting the width of the octane bins as 0.1.
[back]
Exhibit 9.5: The image is a histogram displaying the distribution of octane values. The x-axis ranges from 85.9 to 88.1 in increments of 0.1 and represents octane values. The y-axis, labeled in percentages, ranges from 0% to 14%, indicating the relative frequency of each octane range. The histogram is symmetrical around the peak value at 87.0, which reaches the highest frequency of just below 14%. Each bar represents a 0.1 octane range, with the tallest bars centered around 87.0, showing a significant clustering of data around this value. The standard deviation, noted as σ = 0.3, is marked on either side of 87.0 with lines extending to 86.7 and 87.3. This suggests the normal distribution pattern is likely, with the proportions decreasing symmetrically as the values move away from the center.
[back]
Attribution
“9.3 Contract Performance Quality” is adapted from “Chapter 10: Project Quality and Control” in Essentials of Project Management, copyright © 2021 by Adam Farag, licensed under a Creative Commons Attribution-NonCommercial-ShareAlike 4.0 International License, except where otherwise noted.
Exhibits 9.4 and 9.5 are taken from Figures 10.1 and 10.2 from “Chapter 10: Project Quality and Control” in Essentials of Project Management, copyright © 2021 by Adam Farag, licensed under a Creative Commons Attribution-NonCommercial-ShareAlike 4.0 International License, except where otherwise noted.
The multiple choice questions in the Checkpoint boxes were created using the output from the Arizona State University Question Generator tool and are shared under the Creative Commons – CC0 1.0 Universal License.
A project management concept that says every project operates within the boundaries of scope, time, and cost.
What you get when you add up the cost of all the prevention and inspection activities you are going to do on your project.
Using the results of quality planning on other projects to set goals for your own.