10.4 Use of Population Descriptors in Genomics
Population Descriptors
Appropriate use of population descriptors is a critical scientific issue that is important for advancing genomic research and improving healthcare across human populations. Given the ethical, legal and social implications of their historical and current use, thoughtful use by researchers and other interested parties is essential.
The inaccurate belief that human populations are biologically distinct has contributed to harm, such as justifying eugenics through the practice of scientific racism, and marginalizing groups. In turn, misapplication of concepts of population groups has contributed to health disparities, alienated marginalized groups from research participation, and led to harmful stereotypes that have reinforced inequities.
More work is needed to educate researchers, clinicians, policymakers, and the public on the distinctions between race, ethnicity, and genetic ancestry and advance the use of population descriptors in genomics and biomedical research.
The National Academies of Sciences, Engineering and Medicine (NASEM) assessed the methods, benefits and challenges in a review of the use of population descriptors in genomics research. The NASEM Report includes 13 recommendations to transform how population descriptors are used in human genetics and genomics research.
Types of population descriptors
Population descriptors are ways of describing or distinguishing people from each other based on perceived or actual differences. They capture the various ways in which people can differ from one another.
A wide variety of population descriptors describe groups of people in research, healthcare or society. Examples of population descriptors include race, ethnicity, skin colour, genealogical ancestry, genetic ancestry, Indigenous, primary language spoken, nationality, geographic origin, sex at birth, gender identity, disability status and age. Each population descriptor captures a different aspect of a group or individual. One population descriptor cannot fully describe or distinguish any individual or group. Depending on the situation, some population descriptors may be more relevant than others.
People commonly use population descriptors and their corresponding categories or numerical scales to describe themselves and others. For example, we use categories like female, male or intersex when referring to the biological sex assigned at birth (Phenex Toolkit, 2024-a). We use numerical values like months and years when referring to age. We also use categories like newborn, adolescent or older adult.
Researchers in genomics and healthcare also use population descriptors and corresponding categories to describe who is participating in a research study, what groups are being compared as part of the study and to whom their study findings may apply. These are collectively referred to as demographics. Researchers can obtain information about population descriptors in many ways, for instance, by asking participants how they identify, looking in an electronic medical record, using data from a prior research study that was shared, or searching public records. Researchers may also assign a population descriptor to an individual or group using a specific analytical approach, such as using statistics to look at the frequency of DNA variants across the genome.
The definitions, measurements, uses, and interpretations of population descriptors have varied over time across users and worldwide. Human rights movements or social and political action can bring about such changes. In addition, new scientific discoveries or knowledge, such as in the fields of genomics, archaeology or social science, can lead to changes. New scientific discoveries and well-established facts present an opportunity to improve our understanding of human genetic variation and our knowledge of what types of differences between or across groups may be important for health. For example, the first modern humans lived somewhere in Africa approximately 300,000 years ago (Hublin et al., 2017), and physical barriers to the migration of humans, such as oceans and mountains, led to geographical differences in the frequency of genetic variants we see within and between populations (Rosenberg, 2011).
While we’re often searching for differences, we must remember that human beings are far more similar than they are different. When identifying groups that differ genetically, researchers have found that most of the variation occurs within groups of people rather than between them. This means that nearly all differences are not specific to a group. Instead, they are sometimes found at different frequencies between groups.
Understanding genetic ancestry, race, and ethnicity
Concept in Action
Watch this video from the Canadian Nursing and Genomics Steering Committee on the intersection of genomics, social determinants of health, race and racism for an description and illustration of how these concepts impact nursing practice.
There is not one agreed-upon definition for these terms. The descriptions below highlight key differences across them.
Genetic ancestry
Genetic ancestry refers to the biological relationships between individuals resulting from inheriting common ancestors’ DNA. These common ancestors are tied to their geographical origins from many centuries ago when long-distance travel was extremely difficult. Parents do not pass down all their DNA to their children. Therefore, genealogical ancestry and genetic ancestry can be different. Genetic ancestry is based on a statistical measure of genetic similarity across individuals.
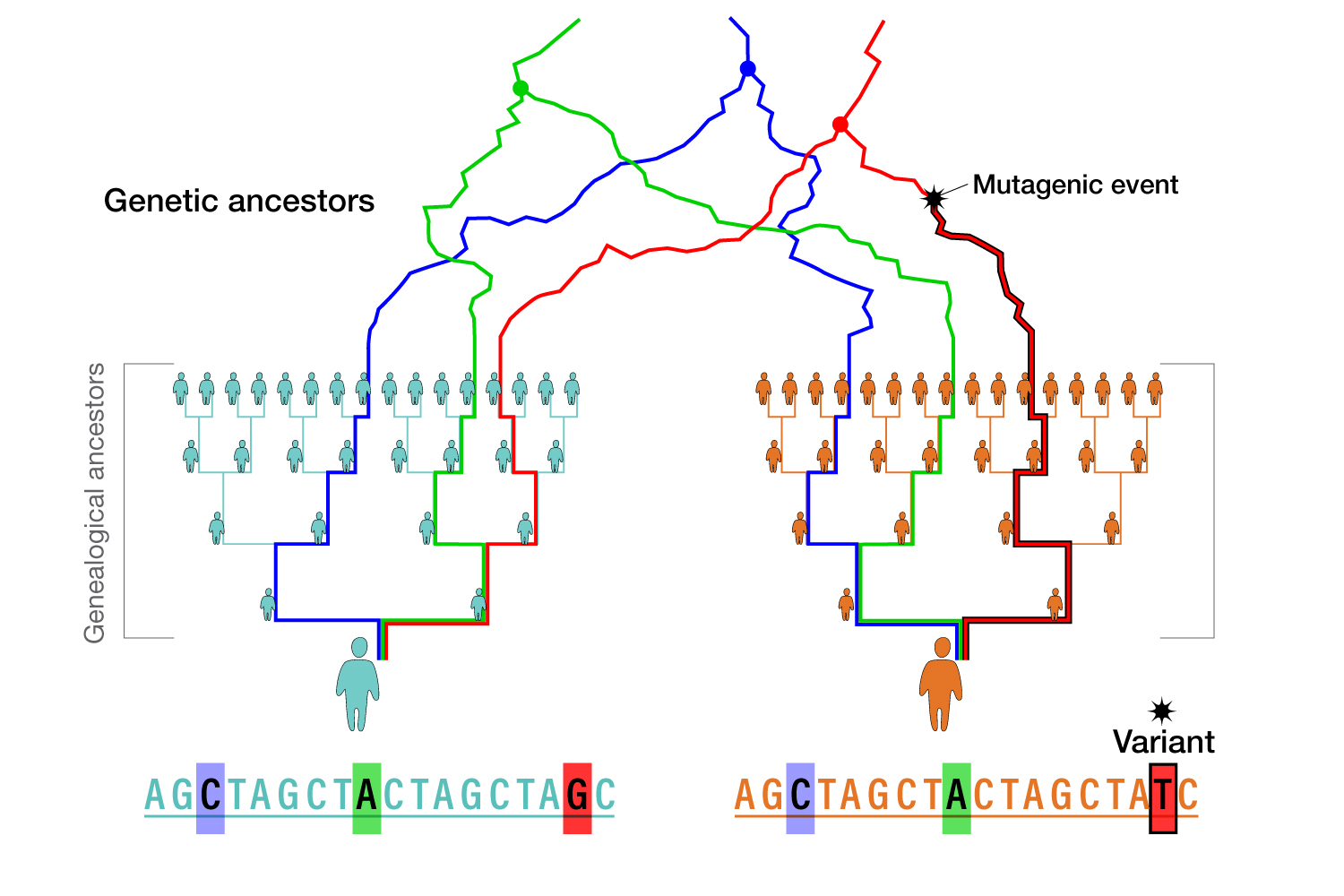
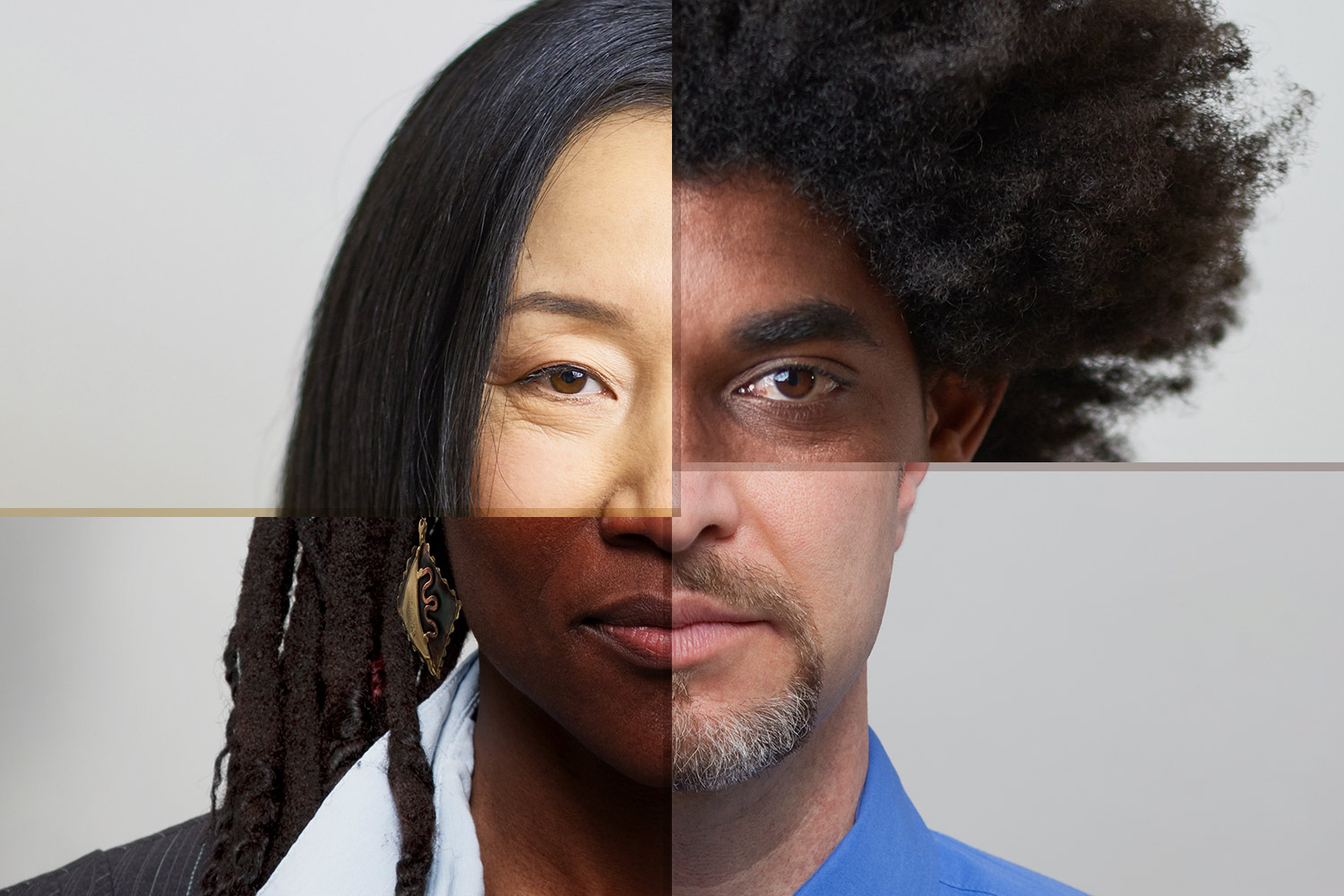
Race
People created the concept of race. Race typically divides human populations into groups based on perceived physical appearance (such as skin colour), social factors and cultural backgrounds (NHGRI, 2022). Race has been used to inappropriately group people into a hierarchical system to “establish and justify systems of power, privilege, disenfranchisement and oppression” (National Museum of African American History and Culture, n.d.-b, para. 1).
Ethnicity
Ethnicity refers to a group of people with shared language, religion, customs, beliefs, heritage and history, even though such attributes are not always confined to a single ethnic group. Ethnicity may also refer to groups that are considered indigenous to an area. Ethnicity is not a biological characteristic.
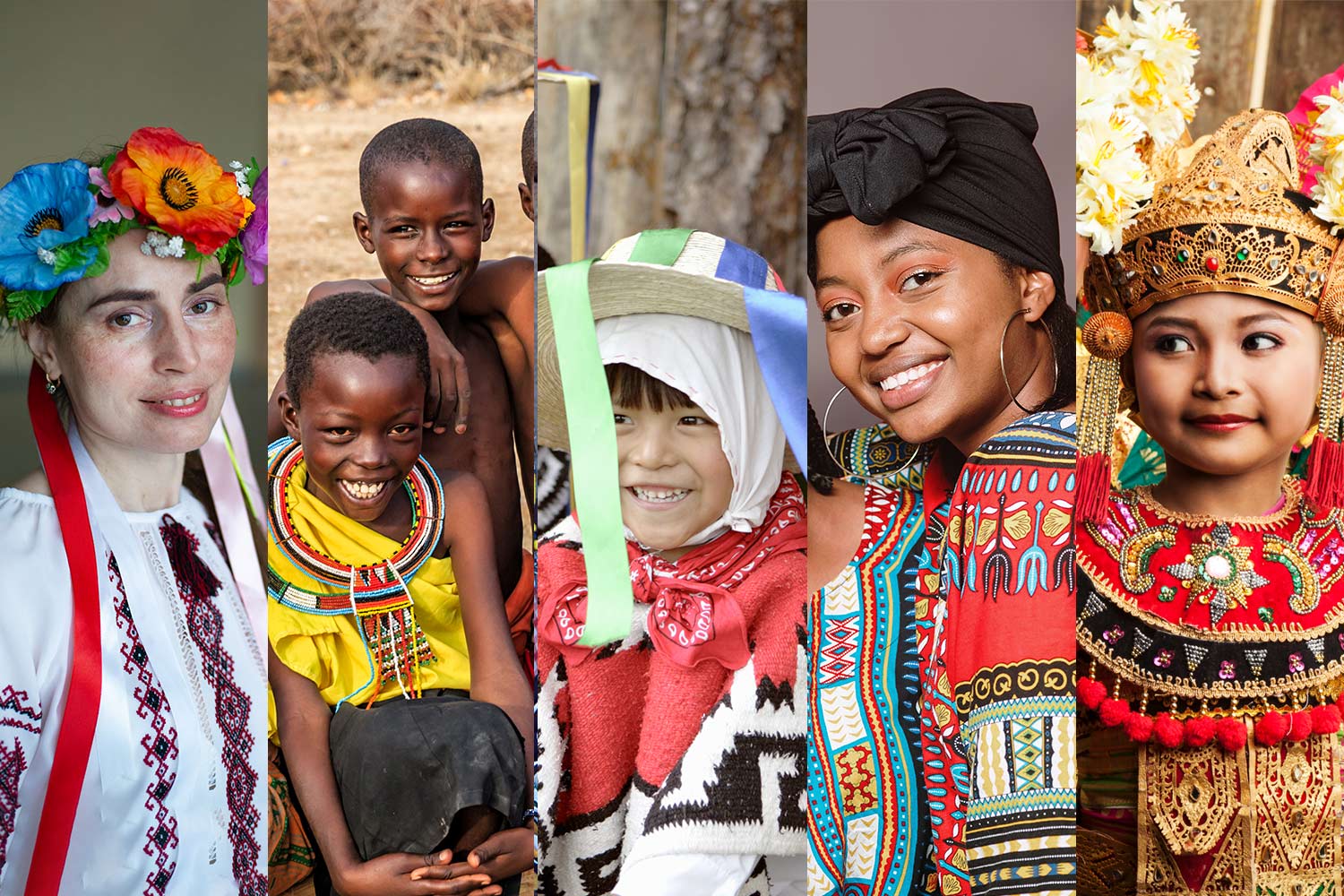
How well can researchers determine genetic ancestry?
Methods for estimating genetic ancestry are evolving. To determine an individual’s genetic ancestry, researchers compare DNA variants in that individual to the frequency of those DNA variants in groups of people from around the world who have provided samples of their DNA. These groups of people form what is referred to as reference populations. Genetic ancestry is estimated using statistical techniques and is typically based on some measure of genetic similarity. An individual with a collection of genetic variants that appear in the highest frequency within a reference population is estimated to have ancestors from that reference population. Individuals may have a collection of genetic variants that appear in more than one reference populations, which indicates they likely have ancestors from more than one group. Some have argued that instead of thinking about genetic ancestry as broad groups or categories, genetic ancestry should be considered a continuum.
Currently, genomic researchers do not have DNA samples from many groups of people around the world, which means genetic ancestries for some geographical locations cannot be estimated accurately. In addition, as mentioned above, scientists use reference datasets to calculate ancestries. Genetic ancestry estimations can differ from one analysis to another due to differences in the frequencies of genetic variants in the datasets used. Furthermore, when someone is estimated to have ancestors from more than one group, researchers sometimes lump individuals together into a single group to simplify analyses. Therefore, determining genetic ancestry is a statistical estimate based on available data and is inconsistent across studies. More recently, companies offering ancestry-related services directly to consumers have combined genetic ancestry information with family history information.
Read
Cerdeña, J. P., Grubbs, V. & Non, A.L. (2022). Genomic supremacy: the harm of conflating genetic ancestry and race. Human Genomics, 16(18), 1-5. https://doi.org/10.1186/s40246-022-00391-2
A closer look: Genetic ancestry and identification
Regardless of the outcome of a genetic ancestry test, people will choose how they want to be identified by others. DNA, social factors, personal or familial preferences, or lived experiences may inform these choices.
How do I identify?
Imagine your friend received an ancestry test for the holidays and was surprised by some results. After taking the test, your friend had a primary care appointment with a new doctor. When completing the required forms, they answered questions about their race and ethnicity differently than in the past based on the latest information provided in their ancestry test. While their physical body and health status did not change, their social identity did change. People vary in their response to ancestry testing. For some, the outcome may lead to an identity change. For others, they may maintain their original identity (Roth & Ivemark, 2018).
But how meaningful is this change for healthcare decision-making? An estimate of genetic ancestry (not race) can be informative for some conditions. For example, some heritable cancers are more common in certain groups than others. Should self-identified race or ethnicity change a doctor’s decision about medical treatment? The answer can depend on a variety of factors.
How am I identified?
As another example, the U.S. government has changed the reporting of race and ethnicity over time, with categories being renamed, merged, removed or expanded (United States Census Bureau, 2015). Was this change due to new information about how people differ genetically or biologically? No. The change was made to reflect better perceptions of growing diversity across groups in the country, better reflect how different people identify themselves and improve the quality of available demographic data. The way people self-identify can change in their lifetime or across generations, along with the questions and forms intended to capture this information.
When the U.S. government established racial categories around 1790, they were tied to colonialism and flawed science. They were used in population surveys for taxation, government representation, counting enslaved persons and maintaining power (Diamond, 2020). The names and number of categories changed over time due to shifts in scientific, political and social thinking about race and ethnicity (United States Census Bureau, 2015).
The major categories used in the U.S. 2020 Census (U.S. Census Bureau, 2020) included Hispanic, Latino or Spanish for ethnicity, and White, Black or African American, American Indian or Alaskan Native and Asian or Pacific Islander.
In addition to their use in the census, race and ethnicity have been used to measure racial and ethnic health disparities and track progress in reducing inequalities. Race and ethnicity are also commonly used as a proxy (Proxy, n.d.). These uses may be helpful for research and public health, especially when other data are unavailable.
Why should we be intentional about how population descriptors are used in genomics research and healthcare?
Advances in genomic medicine greatly amplify the urgency of ensuring the field exemplifies scientific and social accuracy in all our work. Simply stated, the design of some genomic research studies has exacerbated scientific flaws due to how data are being analyzed, interpreted, reported and aligned across data sets. In no small part, this is because of how we misuse population descriptors.
Race and ethnicity are not valid or reliable proxies for genetic ancestry. In addition, genetic ancestry is a poor proxy for the geographic area where someone is from, where they currently live or things that may be part of their surrounding environment. Relying on race, ethnicity or genetic ancestry as a proxy for something not measured in research often hides underlying biological, environmental or social factors that may contribute to health and disease. In healthcare, race and ethnicity have been improperly treated as biological or innate characteristics.
In society, there are tangible and measurable impacts of one’s racial or ethnic identity on health, wellness and status in the United States, whether self-identified or assigned by someone else. Thus, race and ethnicity may help examine social or political issues, document racial/ethnic health disparities, explore the impact of racial bias in health service delivery (Smedley et al., 2003) and monitor diversity, equity and inclusion efforts within the biomedical workforce. Directly measuring and analyzing social determinants of health (SDOH), such as racism, violence, access to nutritious food or safe water, or exposure to trees and nature, would improve the rigour and usefulness of research. A growing collection of SDOH measures is available in a toolkit for researchers (PhenX Toolkit, 2024-b).
In all types of research, when using population descriptors, researchers should be clear and transparent about which population descriptor(s) they are using, how they are measured and why they were chosen. Researchers should have a reasonable hypothesis for why specific descriptors may or may not be important to their research questions. Research should use labels and categories that accurately reflect what is being measured. Researchers should carefully consider whether race, ethnicity or genetic ancestry directly causes the health differences across individuals or groups. If proxies are used in research because data of interest are unavailable or cannot be collected, then the challenges and limitations of doing so should be acknowledged.
A closer look: Measuring “race” in heart disease research
Imagine three different studies that look at the severity of heart disease among people living in three different regions of the United States. “Race” is one of several variables analyzed in each study:
- The first study measures race by asking participants to select a category that best describes their race by checking a box on a form.
- The second study measures race by asking each participant for a saliva sample and using genetic analysis to group study participants into different races.
- The third study uses the birth certificates of participants and their parents to assign a race to each participant.
All three studies use similar labels — Black, White, Native American, Hispanic, Asian and Other — when reporting their findings of heart disease across groups. After completing their analysis, all three studies conclude that “race” is a key factor in the severity of heart disease.
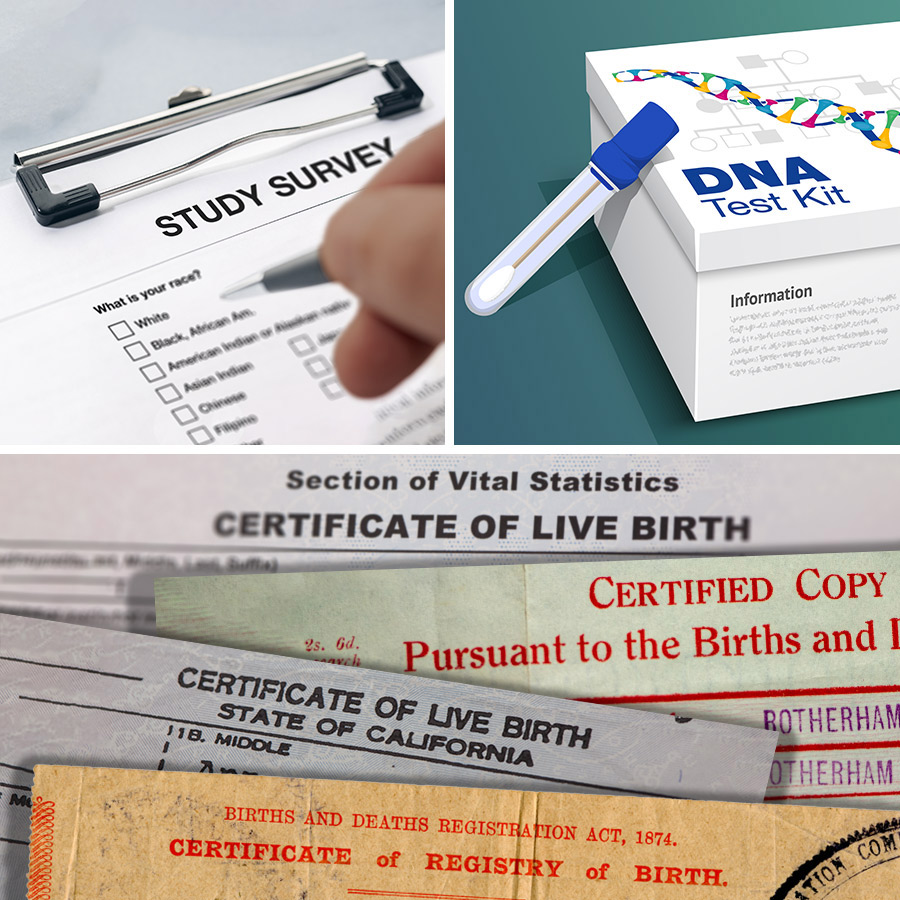
In this scenario, the same population descriptor and group labels are used in each study, but their measurements are different and range from self-reporting to DNA analysis to using vital records. In the second study, race and genetic ancestry appear to be merged as if they are similar or equal. We don’t know from this scenario why each study is including race as a variable. The reasons may be varied.
Suppose studies are unclear or inconsistent in the labels, definitions, measurements or justifications used for population descriptors in research. Our ability to advance science and improve health outcomes is compromised. For example, when research approaches are not specified, it is hard to repeat a study to confirm its accuracy or to see if the same outcome occurs in a different population or part of the world. Furthermore, broad categories for genetic ancestry can obscure DNA variation relevant to understanding certain health conditions (Rotimi & Jorde, 2010).
Poor use of population descriptors can also cause harm to communities. Findings from such studies are more likely to be misinterpreted and misused. For example, readers may believe there is something biological about race when a study uses DNA analysis to analyze “race” differences. Over the last seven decades, various population descriptors have been used in genomic research studies and have varied (Ganguly, 2021).
Using population descriptors in genomic and biomedical research is a critical scientific issue with varied ethical, legal and social implications (ELSI). NHGRI will continue focusing on this issue to promote the ethical, responsible and scientifically rigorous advancement of genomic science, genomic medicine and ELSI research. NHGRI is also focusing on this issue to:
- Recognize that people have been and continue to be harmed by the misuse of race in genomic research and the misinterpretation of research findings.
- Avoid repeating mistakes of the past, which have caused immediate and long-lasting harm to minoritized and disenfranchised groups here in the U.S. and worldwide.
- Earn the public’s trust by ensuring that researchers thoughtfully consider whether, when and how to use population descriptors and ensure they are used ethically.
- Build and maintain trust in science among those we hope will participate in genomic research.
- Ensure a more complete understanding of the diversity across people who participate in research.
- Ensure that all populations benefit from advances in genomic and biomedical research.
- Improve health equity and eliminate disparities in genomic medicine.
Looking forward
Understanding the true role of genomics in health and wellness will require careful attention to the full spectrum of potential contributing factors, including genomic, biological or clinical traits; components of the natural, built or social environment in which people live; and more significant systemic or structural issues. Clarity and specificity around population descriptors used in genomic research can improve the scientific integrity of research while also showing respect for the people represented in genomic research.
Attributions & References
Except where otherwise noted, this page is adapted from Use of Population Descriptors in Genomics courtesy of National Human Genome Research Institute (NHGRI), Public Domain with attribution. / References converted and attributed using APA.
References
Australian Bureau of Statistics. (2011, July). 2071.0 – Reflecting a nation: Stories from the 2011 Census, July 2011. https://www.abs.gov.au/ausstats/abs@.nsf/Lookup/2071.0Feature+Article2July+2011
Australian Bureau of Statistics. (2021). Sample copies of the 2021 Census paper forms. Australia Census. https://www.abs.gov.au/census/census-media-hub/resources/education#sample-copies-of-the-2021-census-paper-forms
Dauda, B., Molina, S. J., Allen, D. S., Fuentes, A., Ghosh, N., Mauro, M., Neale, B. M., Panofsky, A., Sohail, M., Zhang, S. R., & Lewis, A. C. F. (2023). Ancestry: How researchers use it and what they mean by it. Frontiers in Genetics, 14. https://doi.org/10.3389/fgene.2023.1044555
Diamond, A. (2020, April 10). The Enumerated Story of the Census. Smithsonian Magazine. https://www.smithsonianmag.com/history/enumerated-story-census-180974648/
Ganguly, P. (2021, October 19). Language used by researchers to describe human populations has evolved over the last 70 years. National Human Genome Research Institute. https://www.genome.gov/news/news-release/language-used-by-researchers-to-describe-human-populations-has-evolved-over-the-last-70-years
Hublin, J., Ben-Ncer, A., Bailey, S. E., Freidline, S. E., Neubauer, S., Skinner, M. M., Bergmann, I., Cabec, A. L., Benazzi, S., Harvati, K., & Gunz, P. (2017). New fossils from Jebel Irhoud, Morocco and the pan-African origin of Homo sapiens. Nature, 546(7657), 289–292. https://doi.org/10.1038/nature22336
Jablonski, N. G., & Chaplin, G. (2010). Human skin pigmentation as an adaptation to UV radiation. Proceedings of the National Academy of Sciences, 107(supplement_2), 8962–8968. https://doi.org/10.1073/pnas.0914628107
National Human Genome Research Institute (NHGRI). (2022). Race. In Talking Glossary of Genetic and Genomic Terms. Genome.gov. https://www.genome.gov/genetics-glossary/Race
National Museum of African American History and Culture (n.d.-a). Historical foundations of race. Talking About Race. https://nmaahc.si.edu/learn/talking-about-race/topics/historical-foundations-race
National Museum of African American History and Culture. (n.d.-b) Race and racial identity. Talking About Race. https://nmaahc.si.edu/learn/talking-about-race/topics/race-and-racial-identity
Pew Research Centre. (2020). What Census calls us: A historical timeline. https://www.pewresearch.org/wp-content/uploads/2020/02/PH_15.06.11_MultiRacial-Timeline.pdf
PhenX Toolkit. (2024-a, October 30). Protocol – Biological Sex Assigned at Birth. https://www.phenxtoolkit.org/protocols/view/11601
PhenX Toolkit. (2024-b, October 30). Social determinants of health collections. PhenX. https://www.phenxtoolkit.org/collections/view/6
Proxy. (n.d.). In Oxford Reference.https://www.oxfordreference.com/display/10.1093/oi/authority.20110803100351624;jsessionid=8C39AEE0D2D34DCE364B4456E85125DE
Rosenberg, N. A. (2011). A Population-Genetic Perspective on the Similarities and Differences among Worldwide Human Populations. Human Biology, 83(6), 659–684. https://doi.org/10.3378/027.083.0601
Rotimi, C. N., & Jorde, L. B. (2010). Ancestry and disease in the age of genomic medicine. New England Journal of Medicine, 363(16), 1551–1558. https://doi.org/10.1056/nejmra0911564
Roth, W. D., & Ivemark, B. (2018). Genetic options: The impact of genetic ancestry testing on consumers’ racial and ethnic identities. American Journal of Sociology, 124(1), 150–184. https://doi.org/10.1086/697487
Smedley, B. D., Stith, A. Y., & Nelson, A. R. (Eds). (2003). Unequal treatment: Confronting racial and ethnic disparities in health care. The National Academies Press. https://doi.org/10.17226/12875
United States Census Bureau. (2015). Measuring race and ethnicity across the decades: 1790—2010. https://www.census.gov/data-tools/demo/race/MREAD_1790_2010.html
U.S. Census Bureau. (2020). U.S. Census Bureau 2020 Census Questionnaire. In U.S. Census Bureau. https://www2.census.gov/programs-surveys/decennial/2020/technical-documentation/questionnaires-and-instructions/questionnaires/2020-informational-questionnaire-english_DI-Q1.pdf
United States Office of Management and Budget. (1997). Revisions to the standards for the classification of federal data on race and ethnicity. The White House: President Barack Obama. https://obamawhitehouse.archives.gov/omb/fedreg_1997standards