3 What are Properties of Hit and Lead Compounds?
“If you do not expect to, you will not discover the unexpected.”
– Heraclitus
3.1 Sources of a Lead compound
We have previously defined important properties for a lead compound. A lead compound is a compound that:
- Interacts with the target to achieve the desired biological activity.
- Is amenable to synthetic modifications.
- Can reach the target once administered.
Although a significant amount of time and resources are usually invested into identifying a lead compound, they usually serve as starting points for large scale chemical optimization to evolve into safe and effective drug candidates. Lead compounds can arise from different sources as described below.
3.1.1 Natural Extracts
Many lead compounds are derived from plants, animals, or microorganisms as seen in Chapter 2. Roughly 35% of all current medicines originated from natural sources. Natural products are known for having unique chemical structures and broad diversity in composition of matter. These compounds often contain stereogenic modalities and involve high complexity that translates into diverse biological activity. These natural extracts may contain mixtures of hundreds or thousands of compounds and there can be challenges in identifying and isolating the active ingredient from the mixture, as well as developing a viable route to access the compound by chemical synthesis. Natural compounds may also be starting points for further synthetic chemistry screening. For example, captopril is an antihypertensive agent that was identified following studies of the venom of the Brazilian snake, Bothrops jararaca, which identified 9 peptides that potentiated the effects of bradykinin (a naturally occurring peptide that facilitates vasodilation). Bristol Myers Squibb (BMS) used these peptides as templates for synthetic campaigns that eventually led to the drug, captopril (Figure 3.1).
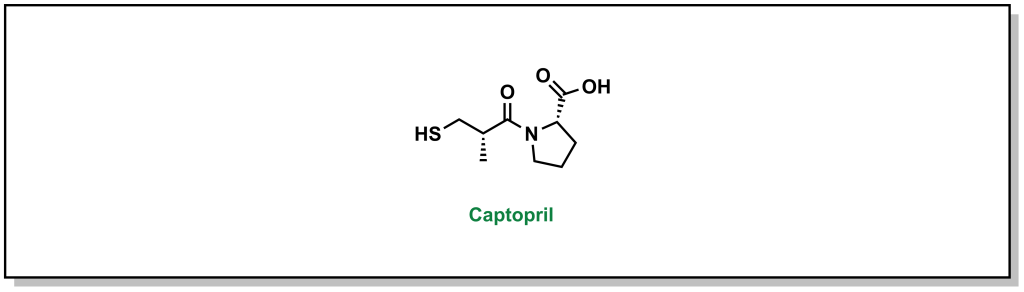
3.1.2 Prior Art
Often lead compounds can be created from modifications of existing drugs. The term ‘prior art’ is borrowed from patent law and refers to compounds that are already known or available in the public domain. This can be convenient because these compounds have safety profiles, synthetic feasibility, efficacy, and metabolic liabilities that are generally understood. As such, prior art can form large aspects of new drug discovery programs. One challenge with prior art is ensuring that there will be ‘freedom-to-operate’ for the chemist on any new composition of matter that would be created, and that the potential new drug could be protected under a new patent. Any derivatives not covered by one patent represent analogues that may be explored by other researchers. For example, consider the patent that covered sildenafil (Pfizer, 1998) and the alternative analogue verdenafil (GSK/Bayer, 2003). Both drugs are currently sold for the treatment of male erectile dysfunction as Viagra and Levitt, respectively (Figure 3.2).
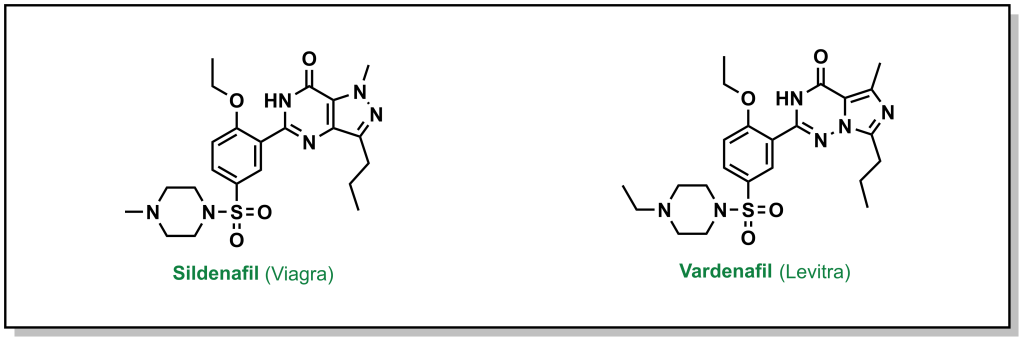
3.1.3 In Silico Screening
With the advent of more powerful computational prediction software in the past 10-20 years, in silico screening has become a mainstream approach for drug discovery. The most routine form of in silico screening involves molecular docking, where the ligand/target interactions of a prospective drug molecule are modelled at the predicted binding site with a three-dimensional conformation of the target. The potential interactions are computationally predicted, optimized, and the compounds are ranked according to parameters such as the free energy of binding (ΔGbind). Thousands of compound libraries can be screened in silico at much higher throughputs and lower resource burden than experimental screens. However, there are limitations that are defined by the structural inputs of the target and the ligand, as well as conformational mobility, especially of the protein target. Many ligands that are computationally predicted from random library screening to bind to the target may not demonstrate any binding, experimentally (in vitro). Notably, there are substantial improvements in predictions if a previous protein-drug structure has been defined (eg. via X-ray crystallography). There are rapidly expanding toolkits of different software packages available for in silico screening; however, any identified hits still need to be experimentally validated. In silico screening is expected to become even more prevalent as computational and AI-driven strategies become more robust, and every steady-state drug screening pipeline will likely involve computational screening at different levels.
3.1.4 Random and Targeted Library Screening
Random screening can involve exploring large chemical libraries without discretion for chemotype or molecule diversity. Since identification of positive hits can rely on serendipity, this is usually performed when there is limited knowledge on the target of interest. The activities of streptomycin and tetracycline were identified in this manner. By contrast, targeted screening can involve a range of chemical moieties and scaffolds that are usually related by at least one chemotype. For example, kinase-targeting libraries can employ molecular species with adenosine as a backbone, and SH2 domain-targeting libraries may focus on short peptides containing a phospho-tyrosine residue. The well understood binding modes of previous inhibitors with these bindings sites provides the chemist with a starting point for building a library of targeted molecules that is more likely to provide positive hit molecules, given the inclusion of an established binding fragment. The scaffolds are often modified with an assortment of chemical moieties and functional groups, which could have a variety of desired impacts such as achieving target selectivity. These types of libraries help improve the probability of obtaining a hit molecule, while also maintaining diversity in the composition-of-matter. One downfall of this approach, relative to random library screening, is the low likelihood of identifying novel binding sites for these protein classes.
3.1.5 Fragment-based Screening
One of the most widely employed strategies in hit-to-lead development is focused on fragment screening libraries. Fragments are low-molecular weight species that are usually characterized by the Rule of Three (the following rules involve parameters that are multiples of 3):
1) The molecular weight of a fragment molecule is < 300 Da
2) The number of hydrogen bond donors ≤ 3
3) The number of hydrogen bond acceptors ≤ 3
4) The number of rotatable bonds ≤ 3
5) The cLogP ~ 3; This parameter refers to the distribution of the compound in a mixture of hydrophobic (octanol) and hydrophilic (water) solvents, and can be experimentally determined in a shake-flask experiment and calculated by the equation: P = [cmpd]octanol / [cmpd]water (1-Octanol is typically used as it is a long saturated alkyl chain with a hydroxy group that mimics the lipid membrane and is sparingly soluble in water). The term cLogD is used to describe cLogP at a specific pH if the compound has an ionization site(s).
Screening libraries of fragment molecules can provide initial hit compounds that will be starting points for eventual lead discovery. However, since these are low molecular weight molecules, they are limited in their capacity to engage in multiple binding interactions and usually need to be modified substantially. Fragments can be altered into lead molecules through different chemical strategies, predominantly through four approaches that were summarized by Rees et al. in 2004 (Nature Reviews Drug Discovery).
3.1.5.1 Fragment Evolution
In this approach, a fragment is identified that binds to a specific site on the target, and structural information is employed to build out the fragment and reach other interactions within the binding site. For example, the tricyclic indole 12 (Figure 3.3) derivative was identified from an NMR screen as a modest hit against the anti-apoptotic protein, Mcl-1. Evolution of the hit through accessing hydrophobic interactions led to 10,000-fold improvement in potency over different iterations and introducing functionality to engage with adjacent regions of the active site.
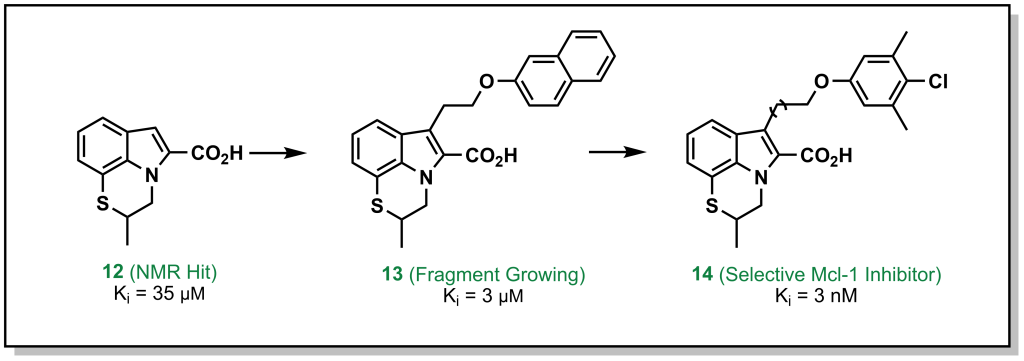
3.1.5.2 Fragment Linking
Fragment linking, involves two fragments that bind in close proximity to each other on the target, and can be linked synthetically to provides a higher affinity molecule. For example, the pyrrolidine and piperidine-based fragments 15 and 16 (Figure 3.4) were identified to bind to the protein EthR with high micromolar affinity. Linking both of these proximally binding fragments resulted in 10-fold improvement in affinity which was further improved (another 10-fold) via additional fragment evolution. In some cases, flexible alkyl (or other) linkers are included to idealize distances for binding of both fragments and later optimized in the SAR.
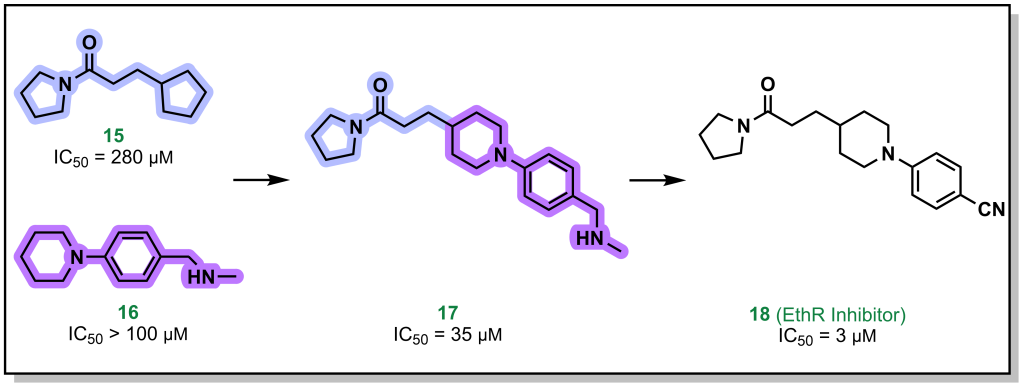
3.1.5.3 Fragment Self-Assembly
Fragment self-assembly is a variation of fragment linking where the fragments can be engineered to possess complementary reactive functional groups. In this way, if the fragments bind in close proximity to each other, they can self-anneal to form a new molecule that has increased potency than the individual fragments A and B (Figure 3.5). For example, the individual fragments have >1 mM binding affinity for CDK2 but following imine condensation form a molecule with an IC50 of 30 nM.
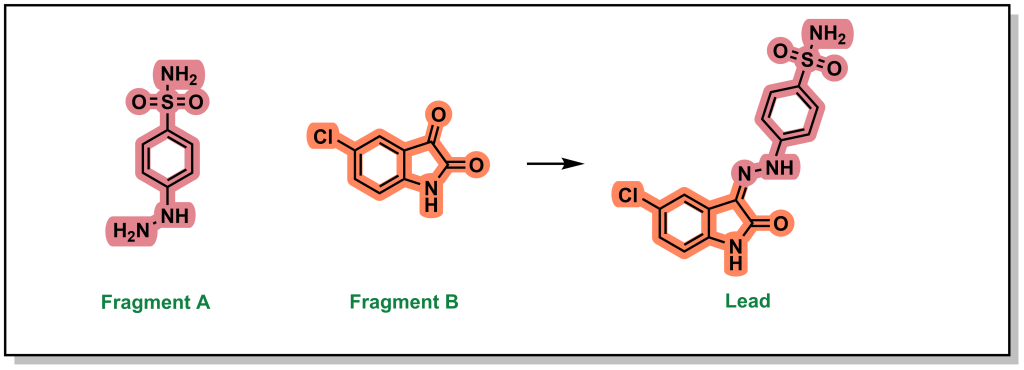
3.1.5.4 Fragment Optimization
The final approach (fragment optimization) is similar to conventional SAR optimization (i.e. a functional group determined to be relevant for target binding and is modified to increase binding or other desirable molecular properties). For example, drug candidate LY517717 for the blood clotting factor Xa, was built from naked benzimidamide fragments (with >200 µM potency), followed by more advanced in silico screening to design and synthesize larger molecules for bio-assay testing. The original fragment was ultimately replaced to improve oral bioavailability, highlighting the iterative steps involved in building and optimizing a fragment hit into a lead molecule.
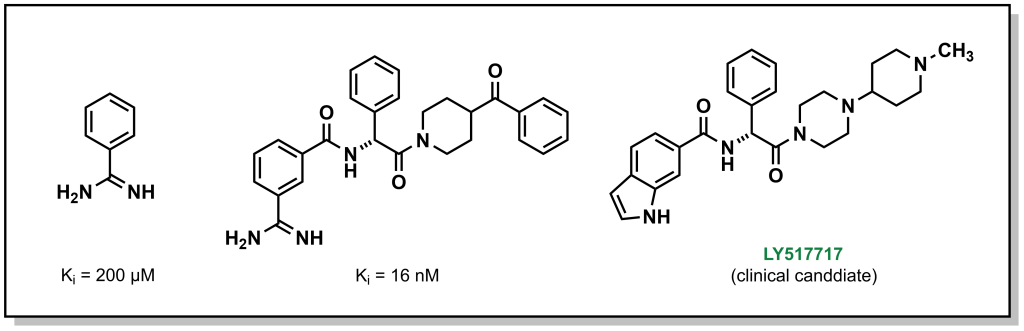
In all cases, these fragment-based approaches enable synthesizing de novo compounds with the goal of precision-based medicines by elaborating on chemical hits and fragments that have positive binding properties.
3.2 Measuring Target-Compound Binding
A key element of drug discovery programs involves stratifying compounds into potent hit and lead molecules which requires quantifying their capacity to engage with the target to objectively discriminate between compounds. This is most commonly performed through two parameters: i) determining the concentration where a molecule elicits half of its maximal inhibitory effect (IC50) or ii) a dissociation constant (Kd).
IC50 values are one of the most commonly measured parameters in drug discovery. In an assay to determine IC50 , a parameter such as enzyme reaction rate is monitored as a function of the inhibitor/drug concentration (the enzyme and substrate are held at constant concentrations in the assay). The assay is performed multiple times with different concentrations of the inhibitor. In experimental trials where the inhibitor concentration is high, the inhibitor can compete with the substrate to reduce enzyme activity. At a certain inhibitor concentration, 50% of the enzyme activity will be inhibited, which provides the IC50 value (in less common assays, comparable values such as IC30 or IC80 are derived which reports 30% and 80% inhibition respectively). A compound with a lower IC50 value indicates a more potent compound, since it requires a lower concentration to inhibit the enzyme. An important aspect of determining IC50 values, is that if the assay is repeated with increased substrate or more enzyme, the IC50 value will increase (as this requires more drug to inhibit the enzyme). As such, changing conditions for the assay can mis-represent the apparent potency of a drug, and these are relative measurements that can only be compared between compounds if uniform conditions were used across the entire series.
Contrastingly, Kd characterizes the protein-ligand interaction with an absolute value that is intrinsic of the inhibitor and not dependent on enzyme/substrate concentration. In this case, the inhibitor is introduced to the enzyme at different concentrations, and the binding is measured directly (as opposed to another parameter such as reaction rate or product formation). Kd measures the binding equilibrium between the enzyme and inhibitor, and similar to IC50 values, the lower the Kd the more potent the inhibitor. Unlike IC50 values, Kd values for different compounds can be compared and ranked (even in different assay conditions) and a compound with a Kd in the nM regime is more potent than a compound in the µM regime. Hit compounds generally exhibit low to modest µM Kd values whereas lead compounds are typically optimized to nM or pM Kd values. Depending on the assay and tools available, Kd can be more challenging to interrogate and is less applicable in understanding a phenotypic effect, such as cellular cytotoxicity. When dealing with an inhibitor-target complex, the term inhibitory constant (Ki) maybe used in place of Kd. Ki generally requires even more intensive experimentation to determine, and involves measurement of compound inhibition across a series of inhibitor and substrate concentrations, in order to determine the intrinsic inhibitory activity for a particular enzyme target.
For inhibitors that compete with substrate for binding to the protein target, the Cheng-Prusoff equation can be used to convert IC50 values into Ki values, although the Michaelis constant (Km) should be a known variable.
Ki = IC50 / (1 + [S] / Km)
In general, it is often more feasible to determine IC50 values for all of the compounds in a library and ensure that the assay conditions remain constant. Notably, while lower Kd and IC50 values both indicate a more potent compound, due to the principles of thermodynamics, an experimentally determined IC50 cannot be lower than the Kd value. Therefore, it is not possible to keep reducing the concentration of substrate in an assay to artificially reduce the IC50 values observed.
3.3 Binding is Determined by Drug : Target Interactions
Ultimately, the capacity of a drug to engage with a target is determined by the thermodynamic binding energy. In a reversible reaction, the target enzyme (E) will bind an inhibitor (I) to give the complex E●I, where the equilibrium is governed by the constant Kd (or Ki in the presence of substrate). One of the main goals of medicinal chemistry and a significant portion of hit-to-lead investigations is to design molecules that will improve (decrease) the Kd. Understanding how to improve Kd, requires an understanding of the types of interactions that can occur between a target and its inhibitors. There are generally three types of binding interactions, i) electrostatic, ii) hydrogen bonding, and iii) “hydrophobic” interactions.
3.3.1 Electrostatic Interactions
Electrostatic interactions involve the interaction between two charged, or partially charged, species. These types of interactions represent the strongest type of non-covalent interactions. However, they are the most relevant in a hydrophobic environment. Within the environment of a polar solvent such as water, the charges may be partially of fully masked, as there is an increased opportunity for the ions to be solvated. Resultantly, the interactions between the charged residue and solvent (water) would need to be disrupted to facilitate inhibitor binding, which results in additional energetic binding penalties. In addition to location/environment, the intermolecular distance between the ions is also important as the strength is determined by Coulomb’s law of electrostatics. Therefore, lead molecules need to be optimized in order to position functional groups at ideal distances to target the appropriate residues on a protein. There are three main types of electrostatic interactions that are important in medicinal chemistry.
3.3.1.1 Salt-Bridges
Salt bridges typically form between positively and negatively charged species and play a large role in stabilizing compound binding. Salt bridges are the strongest type of electrostatic interaction (–20 to –40 kJ/mol). As with all electrostatic interactions, their strength relies on the distance between the two species. However, since these interactions are quite strong, they are the ‘least distance-dependent’ electrostatic interaction. Salt bridges generally dominate the initial long-range interaction between drugs and receptors.
Salt bridges largely depend on the dielectric constant of the media. The dielectric constant is a physical property that reports on the solvent polarity and varies substantially based on the environment. For example, the dielectric constant is ~80 for bulk phase water, 28 near the surface of a protein, and 4 at the interior of a protein (which is comparable to the highly non-polar organic solvents such as chloroform or dichloromethane). Salt bridges generally arise from specific amino acids – arginine, lysine, histidine (positively charged) or aspartic and glutamic acid (negatively charged) and will depend on the pKa of all amino acid side chains. For example, in the kinase inhibitor shown below (Figure 3.7), introducing an N,N-dimethylamino group in place of the isopropyl tail introduces a positively-charged nitrogen that is capable of forming a salt-bridge with the carboxylate group of Asp 831 on EGFR, leading to an ~1000-fold improvement in potency.
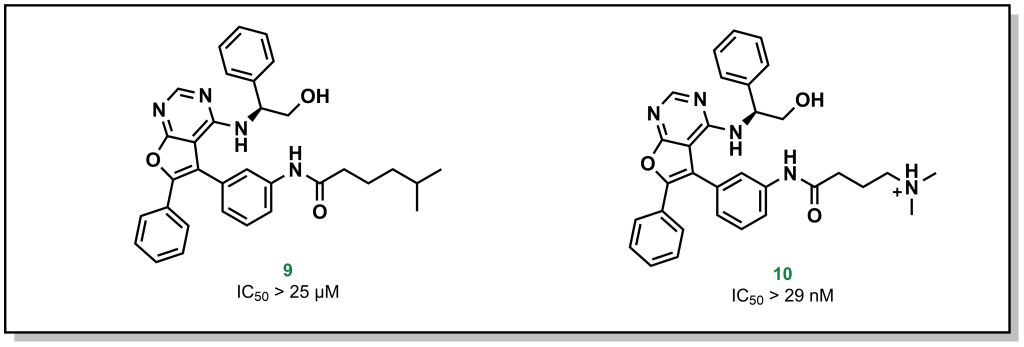
3.3.1.2 Ion-Dipole Interactions
Ion-Dipole interactions result when one of the binding partners does not possess a full electrostatic charge. A dipole occurs when the electron density of a covalent bond is asymmetrically distributed as a result of the electronegativity difference between the two atoms (unequal sharing of electrons). This creates a directionality for a partial charge that can interact with a stronger ionic charge. The energy associated with ion-dipole interactions is –12 to –20 kJ/mol and decays with distance according to 1/r2 distance. Zapleon (Sonata) is a sedative used to treat insomnia that engages with GABA-A receptor via an anionic carboxylate sidechain which interacts with carbonyl centre of the drug in an ion-dipole interaction (Figure 3.8).
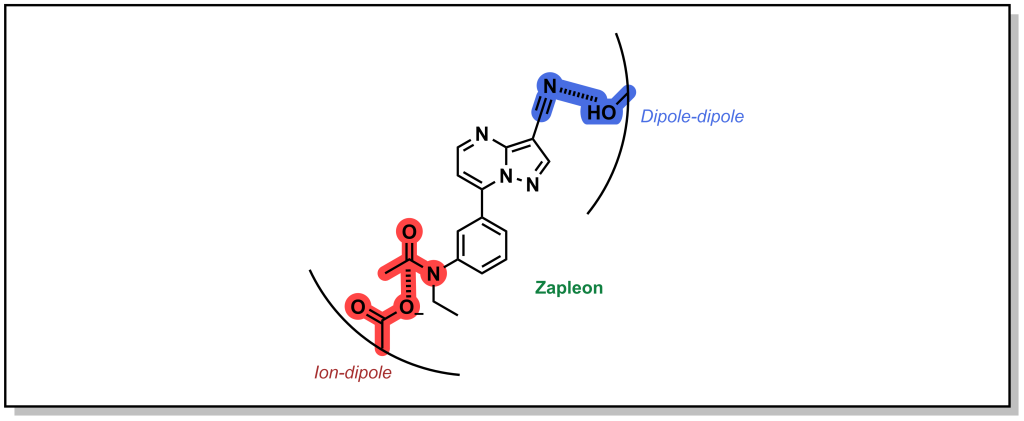
3.3.1.3 Dipole-Dipole Interactions
Dipole-dipole interactions are generally the weakest electrostatic interaction (–4 to –12 kJ/mol) but are the most common mode of interaction in drug-target binding and drive the affinity of many drugs for their target. Importantly, the magnitude of the dipoles is important, but the angle between the dipoles also affects the strength of the interaction. Certain angles are non-productive, and produce a zero value and depending on orientation. The energy of the interaction also decays with distance1(/r3). In the example above (Figure 3.8), the nitrile of Zalepon interacts with hydroxy-moiety of the side-chain in a dipole-dipole interaction.
3.3.2 Hydrogen Bonding
Hydrogen bonding involves sharing a hydrogen nucleus (proton) between an electron-rich heteroatom and an electronegative heteroatom. The electronic rich heteroatom (H-bond acceptor) must have a lone pair of electrons and is usually a nitrogen or oxygen atom. The H-bond donor is typically an electronegative heteroatom whose covalent bond to a hydrogen is highly polarized towards the electronegative atom (strong dipole). This also means, that generally speaking, carbon is not involved in H-bonding.
The free energy for H-bonding is usually within the rage of –6 to –30 kJ/mol and depends on the intermolecular distance and orientation of the H-bond. The shorter the H-bond, the more orbital overlap, and the stronger the interaction (typical H-bonds range from 1.5 to 2.2 Å). Similarly, the more linear the orientation, the greater the orbital overlap, with the maximum overlap occurring at 180º. Stronger H-bonds have a higher degree of symmetry which enables the H atom to be shared more equally.
Both oxygen and nitrogen are common hydrogen bond donors and acceptors, because they are electronegative atoms with lone pairs of electrons. Sulfur is a heteroatom, right under oxygen in the periodic table. However, sulfur is generally not an ideal hydrogen bond acceptor because its lone pair is in a 3s orbital which results in a large and diffuse electron cloud.
H-bonds can be critical to ensuring the proper inhibitor binding. Vancomycin is a non-ribosomal glycopeptide that acts by inhibiting cell wall synthesis in gram positive bacteria. It binds to a conserved sequence (acyl-D-Ala-D-Ala) at the end of the peptidoglycan chain and inhibits cross-linking. However, antibiotic resistance occurs when bacteria are capable of replacing the terminal D-Ala with D-Lac residue (lactate ester for an amide). This substitution disrupts a critical H-bond and leads to a 1000-fold reduction in affinity enabling bacterial survival (Figure 3.9).
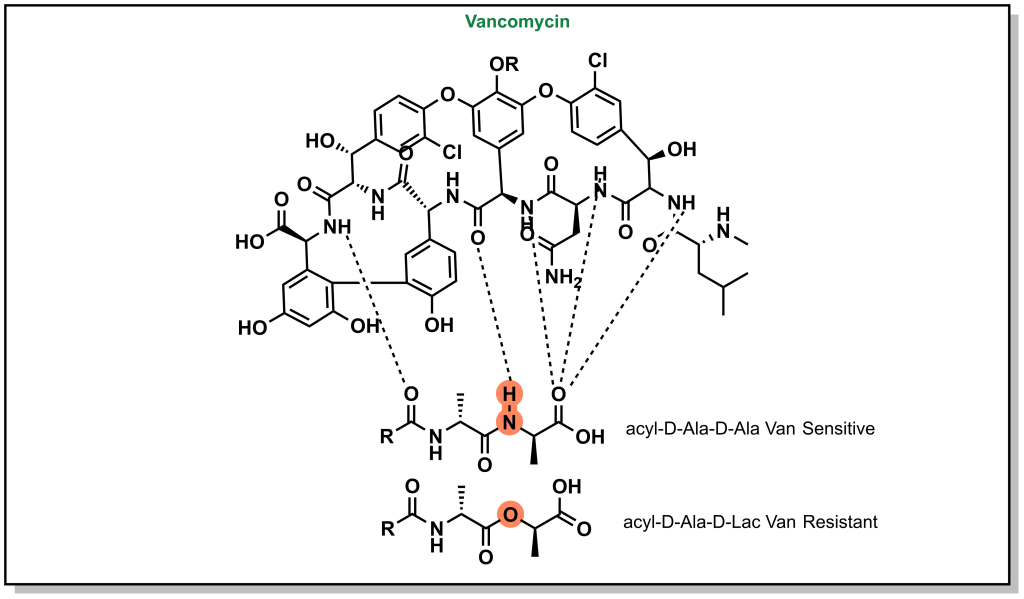
3.3.3 Steric Interactions – Van der Waals Interactions
Van der Waals interactions take place between non-polar molecules over very short distances (decay according to 1/r6). These molecules do not have a significant electrostatic attraction, but can result in transient dipoles, which can induce dipoles in other hydrophobic species. These are weak attractive forces (–2 kJ/mol), although the cumulative sum of these forces can be significant. Van der Waals interactions are common between the aliphatic groups of the molecule and also occur during aggregation of non-polar molecules in water or other polar solvents. There are also specific types of hydrophobic interactions that can occur in a subset of functional groups.
3.3.3.1 π-π Effects
Phenyl rings are found in 45% of all currently marketed drugs, partially because of the range interactions they can engage with, but also the known exit vectors of substituents, which allows for programmable optimization of the molecular structure. The electron density around an aromatic ring is delocalized and stabilized over the entire ring via resonance. The area above and below the plane of the ring is considered electron-rich and the movement of electrons through this system creates a quadrupole moment that can interact with cations (cation-π effect) and is shown to occur with positive charged residues (Lys/Arg) or aromatic residues (Phe/Tyr/Trp).
Alternatively, different types of π-π stacking can occur between closely positioned aromatic rings conformations. There are different types of π-π interactions, including sandwich, edge-to-face, and displaced π-π stacking. Although it is not a strict requirement, the highest attraction in π-π stacking occurs when the one of the π systems has an electron-donating substituent and another π system has an electron-withdrawing substituent (which creates pseudo-dipole interactions). Modern drug molecules nearly always harbour aromatic rings leading to critical π effects that are often stronger than conventional Van der Waals interactions. For example, crystallography analysis of an inhibitor library generated to target soluble epoxide hydrolase (sEH) reveals that introduction of an additional phenyl ring improves potency by 100-fold as a result of a new π – π interaction with His524 of the protein (Figure 3.10).
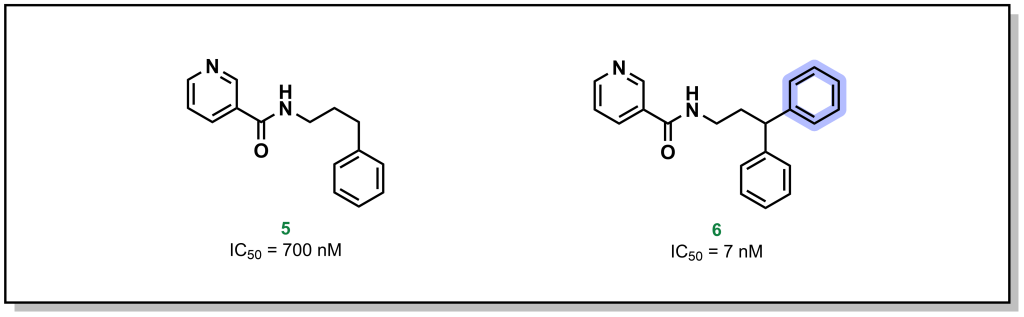
Similarly, the ACE inhibitor, donepenzil, participates in multiple aromatic interactions such as a cation-π interaction of the tertiary amine with Phe330, π-π stacking between the indole of Trp279 and the dimethoxybenzene, and π-π stacking of the Trp84 indole with the phenyl ring (Figure 3.11).
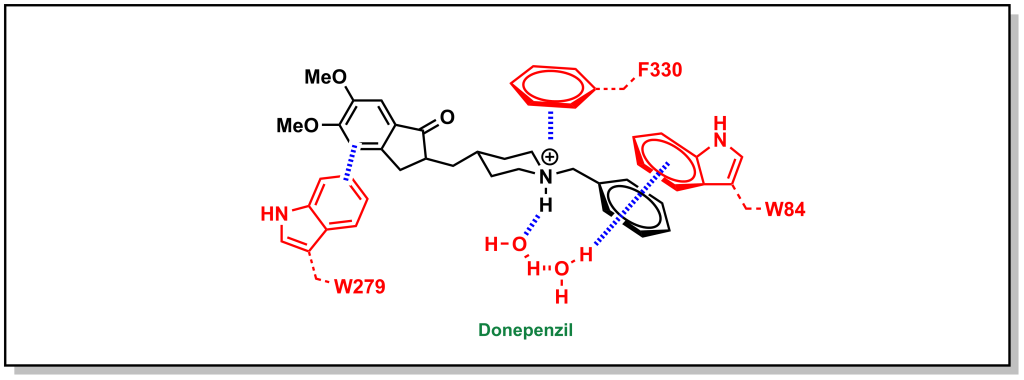
3.3.3.2 Halogen Bonding
Halogen bonding is a more exotic interaction that occurs with large, sigma-bonded halogen substituents. The sigma bond creates an asymmetry in the electron cloud focussed towards the equatorial axis of the halogen (with respect to the bond) and a partial positive charge directly opposite to the bond or a sigma hole. The sigma hole can act as an electrophilic centre and engage with electron-rich species in the trend I > Br > Cl. Importantly, fluorine is a special case and since it is quite small and electronegative, it does exhibit the properties of the sigma hole. Looking at phosphodiesterase 2 inhibitors, replacement of larger halogens demonstrated increasing potency via electrophilic interactions with the side-chain oxygen of Tyr827 (Figure 3.12).
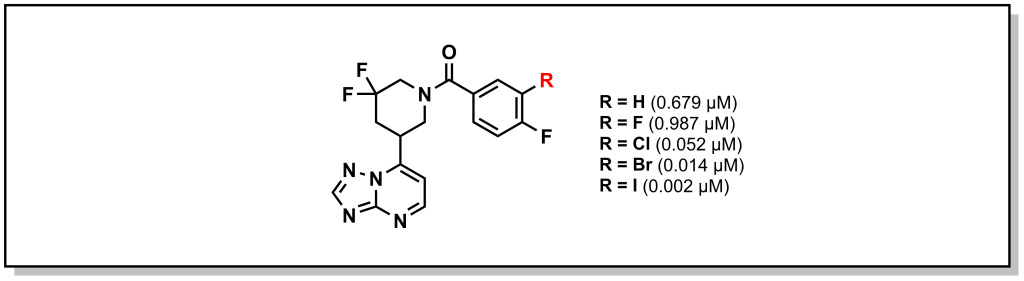
3.3.3.3 Magic Methyls
Methyl groups are small hydrophobic moieties that can drastically alter the affinity of a ligand for its target. For example, in investigating the SAR around tankyrase-2 (TNKS2) inhibitors, introduction of a single methyl group drastically increased the potency by ~50-fold (1 vs. 2; Figure 3.13a). Similar effects are observed for additional compound classes, such as PLD2 (Figure 3.13b) or S1P1 inhibitors (Figure 3.13c). The rationale for this substantial difference is often attributed to displacement of an unfavourably located water molecule in the binding site, which is both entropically favourable (water release) as well as enthalpically favourable (hydrophobic interactions of the methyl group). Additionally, methyl groups can also modify the dynamics of ligand to lock-in a favourable conformation. However, this is not a conserved effect and methyl group introduction can also lead to decreases in potency, particularly if the methyl group is not buried in a hydrophobic pocket upon protein-binding, or if an unfavourable conformation emerges.
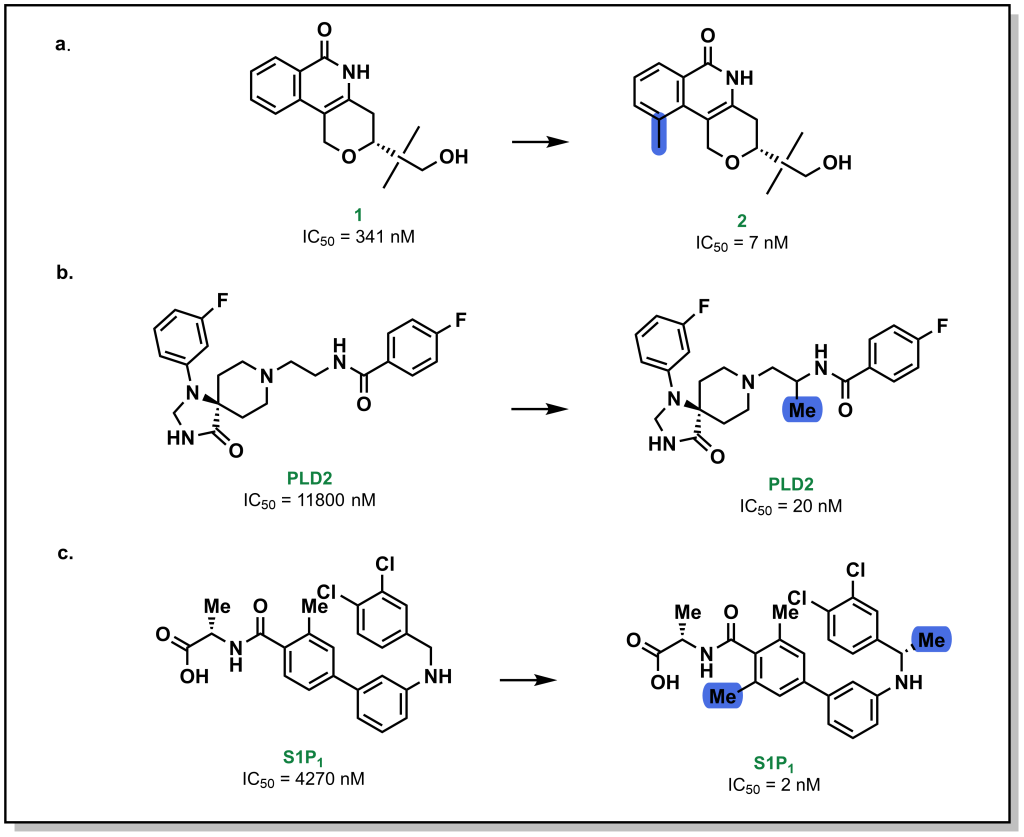
3.4 Energetics of Drug-Receptor Interactions
The interactions that are discussed above contribute to the Gibb’s free energy of binding (ΔG) for a ligand to its target. Thermodynamically, this can be explored as the enthalpy and entropy of the interactions based on the equation:
ΔG = ΔH – TΔS
From a superficial interpretation of physical chemistry, the enthalpy refers to the heat change upon binding and the entropy refers to the number of states that reaction can sample. For example, when a protein binds a ligand via dipole-dipole interactions, this is enthalpically favourable due to energy released by this interaction, but entropically unfavourable because there is one complex compared to the original two “free” species. However, monitoring energetics of these reactions can be more complex as there are multiple species in the system. For example, forming the dipole-dipole interactions may require de-solvation of the ligand or protein which would reduce the enthalpic gain of the reaction. Furthermore, binding of the ligand to the protein may release water molecules from the binding site of the protein that can lead to favourable entropic gains. These energetics are wholistically captured when determining the Kd of the interaction, and the relationship is characterized by the equation:
ΔGd = –RTlnKD
The equilibrium constant of dissociation (KD) is also related to the ratio of the rate constants for the on- and off- rates (koff/kon) for a drug which is critical for the concept of residence time. Although there are many tools to explore the energetics of compound-binding, one common theme is that if the free inhibitor is too flexible it will reduce Kd, since there will be a large entropic penalty upon protein binding. Therefore, a key part of optimizing compounds can involve reducing intrinsic conformational mobility through rigid systems (e.g. aromatic, sp2, or hetero-atoms)
3.5 Properties of Lead Compounds
Optimization of a lead compound requires strategic planning and consideration of all the potential drug-target interactions. When new compounds (hits or leads) are proposed and advanced the following questions are a useful starting point in gauging which properties of the molecule should be optimized:
What is the molecular weight of the compound?
- Large compounds (>500 Da) are susceptible to low cellular permeability.
What hetero-atoms and functional groups are present?
- Common heteroatoms include oxygen and nitrogen and include potential sites for protonation; certain functional groups can have metabolic liabilities or toxicities and can be replaced with bio-isosteres.
Are there any sp3 or stereocentres?
- Stereocentres often complicate both the synthesis and the analysis of activity, but can potentially offer higher potency and more selectivity. Introduction of sp3 sites can be metabolic soft spots.
Are there any sites for protonation or ionizable sites?
- Determining the pKa value for any sites of protonation can provide important information on the charged state of the compound in biological media.
What is the cLogP and tPSA (total polar surface area) of the compound?
- The overall polarity and lipophilicity of the compound provides information on the predicted cell permeability.
How many rotatable bonds are on the compound?
- Highly flexible molecules can be entropically penalized upon binding due to the reduced degrees of freedom and can thus reduce the binding affinity.
How many hydrogen bond donors (HBD) and acceptors (HBA) are present on the compound?
- HBD and HDA can affect the polarity and reactivity of the molecule, as well as its metabolic stability, permeability, and efflux properties.
Answering these questions can provide a solid starting point for lead optimization and highlights physical properties that could potentially be downstream liabilities. A number of these properties were collated by Christopher Lipinski (who worked at Pfizer) in 1997 and developed the rule of five (Lipinski’s rule of five) which provides guidelines from analysis of orally administered drugs. Lipinski’s rules include:
- Molecular mass of 500 Da (or less)
- A cLogP of 5 (or less) [Oral drugs have a moderate lipophilicity of 2-5]
- A maximum of 5 hydrogen bond donors (sum of OH and NH moieties)
- A maximum of 10 hydrogen bond acceptors (sum of O and N atoms)
All of the rules involve parameters which are multiples of five, and provides the basis for the name. Lipinski’s rule of five was extremely influential in organizing compounds in medicinal chemistry and provided threshold goals for drug discovery programs. Although these rules provide crucial starting points, there are multiple drugs that defy these principles including antibiotics, antifungals, and glycosides (although some of these oral drugs have proteins that facilitate their transport across membranes). With the advent of new technologies such as bivalent degraders and protein-protein interaction inhibitors there has been contemporary emphasis on developing guidelines to go beyond the rule of 5 (bRo5).
3.6 Ligand Efficiency
Ligand efficiency (LE) is an important metric in evaluating different molecules in lead discovery. Ligand efficiency attempts to address how efficient a molecule is at engaging a target. For example, if there are two different candidates that both have a Kd of 10 nM, the smaller molecule (based on molecular weight) would be considered more “ligand efficient”: the atoms of the smaller molecule are engaging in more potent interactions, whereas the larger molecule requires more atoms to achieve the same potency. This suggests that some of the additional atoms could be removed if they are not contributing to the potency and offers a starting point for medicinal chemistry iterations. The formula for calculating ligand efficiency is shown below:
LE = ΔGd / #HA
Remember that ΔGd is related to the Kd which directly reports on target-ligand binding. The term ‘heavy atom’ (HA) in this context refers to any atom that is larger than hydrogen (C, N, O, S, etc.). In general, higher values for ligand efficiency are associated with a more drug-like compound, although this is not without controversy. For example, consider the two drugs captopril (an ACE inhibitor used for the management of hypertension) and rosuvastatin (also referred to as the cholesterol lowering agent, Crestor, an inhibitor of HMG-CoA reductase). Captopril has 14 heavy atoms, whereas atorvastatin has 33 heavy atoms. The Kd of captopril to ACE is 8.5 nM and the Kd of rosuvastatin to HMG-CoA reductase is 2 nM. The ligand efficiencies for captopril and rosuvastatin are therefore 3.4 kJ/mol/HA and 1.1 kJ/mol/HA respectively. Although captopril has a higher ligand efficiency, both drugs are widely used and this provides an example of the debate in optimizing drugs based solely on ligand efficiency.