Chapter 12. Advanced Internal Auditing Topics: Analytics, Agile Auditing, and Continuous Auditing
12.01. Leveraging Analytics in Internal Auditing
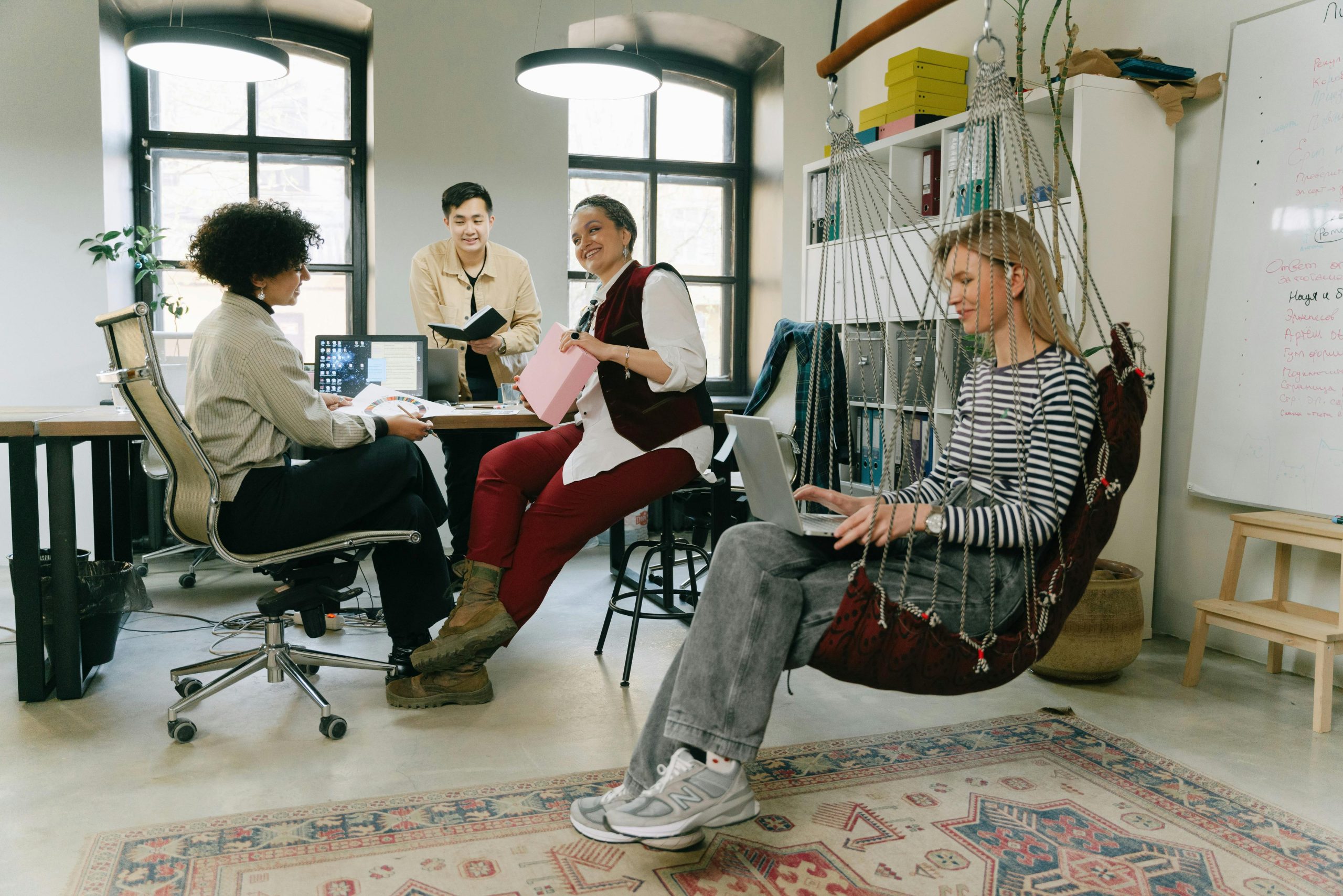
Key Questions
Briefly reflect on the following before we begin:
- How can data analytics transform the internal audit process and its outcomes?
- What types of analytics (descriptive, diagnostic, predictive, and prescriptive) are most valuable for internal auditors, and in what scenarios?
- What challenges do internal auditors face when integrating analytics into their audit processes, and how can these be overcome?
- How can auditors ensure the data used in analytics is reliable and valid for audit purposes?
In the rapidly evolving landscape of internal auditing, leveraging analytics has become increasingly indispensable for organizations seeking to enhance the effectiveness and efficiency of their audit processes. Analytics encompasses systematic data analysis to derive valuable insights and is essential in modern auditing practices. As such, internal auditors progressively turn to data analytics to augment their audit capabilities and address emerging complexities in business environments.
Understanding the fundamental concepts of data analytics is essential for internal auditors aiming to harness its potential. Data analytics encompasses various types, including descriptive, diagnostic, predictive, and prescriptive analytics, each serving distinct purposes in the audit context. From identifying patterns and trends to predicting future outcomes and prescribing optimal courses of action, analytics equips auditors with valuable tools for extracting actionable insights from vast volumes of data. Auditors must leverage various tools and technologies tailored to their specific audit objectives and organizational contexts to implement analytics effectively.
Integrating analytics into traditional audit processes represents a paradigm shift in internal auditing, enabling auditors to conduct more comprehensive and insightful assessments. However, this transition has challenges, ranging from data quality issues and resource constraints to skill gaps and technological complexities. Overcoming these hurdles requires auditors to adopt innovative approaches and collaborate closely with organizational stakeholders. Despite these challenges, numerous success stories abound, showcasing how organizations have transformed their audit functions and achieved remarkable outcomes through the strategic application of analytics.
Internal Audit in Action
Background
Carter Tech, a leading technology firm, has experienced significant growth, bringing new complexities and risks to its operations. The internal audit department recognized the need to adopt audit analytics to enhance its ability to identify, assess, and manage risks.
Challenge
Transitioning from traditional audit methods to an analytics-driven approach posed several challenges, including acquiring the right analytics tools and technologies, training the audit team in data analytics skills, and integrating analytics into existing audit processes.
Action Taken
- Introduction to Data Analytics: Carter Tech’s audit team initiated the transformation by participating in intensive training programs on data analytics, focusing on understanding its importance and learning how to apply it in auditing.
- Types of Analytics Adopted: The team implemented a range of analytics types, from descriptive analytics to understand past audit findings to predictive analytics to anticipate future risks and trends.
- Adopting Tools and Technologies: Carter Tech invested in advanced analytics tools and software, enabling the audit team to collect and analyze large volumes of operational data efficiently.
- Developing an Analytics-Driven Audit Approach: The team restructured their audit approach to integrate analytics at every stage, from planning through execution to reporting, ensuring that data-driven insights informed all audit activities.
- Overcoming Challenges: The initial challenges of skill gaps and resistance to change were addressed through continuous training and demonstrating the value added by analytics through quick wins and success stories.
Outcome
Audit analytics transformed Carter Tech’s internal audit function, significantly enhancing its effectiveness. Analytics allowed the team to uncover previously undetected risks and inefficiencies, leading to more targeted audit recommendations and improved management decision-making.
Reflection
Carter Tech’s journey to leveraging analytics in internal auditing exemplifies the transformative potential of audit analytics. By overcoming initial challenges and integrating analytics into audit processes, the audit function can significantly enhance its strategic value to the organization, moving from a traditional compliance-focused role to a proactive, risk-focused advisory role.
Introduction to Data Analytics and Its Importance in Auditing
Data analytics has revolutionized many fields, including internal auditing. In the context of auditing, data analytics involves using advanced analytical tools and techniques to examine large volumes of data. This allows auditors to identify patterns, anomalies, and trends that might not be visible through traditional audit methods. Adopting data analytics in auditing marks a significant shift from conventional, sample-based auditing to a more comprehensive, data-driven approach. The importance of data analytics in auditing must be considered. It enhances the efficiency and effectiveness of audits by enabling auditors to analyze entire data sets rather than relying on samples. This comprehensive analysis increases the likelihood of detecting errors, fraud, and non-compliance issues. For example, an auditor using data analytics can quickly identify unusual transactions or patterns in financial data that may indicate fraudulent activities.
Where organizations are increasingly required to comply with stringent regulatory standards, data analytics helps ensure compliance and transparency. Internal auditors must adhere to standards set by bodies such as the Chartered Professional Accountants of Canada (CPA Canada) and the Office of the Superintendent of Financial Institutions (OSFI). Data analytics tools can help auditors meet these standards by providing deeper insights into financial statements and operational processes, ensuring they adhere to regulatory requirements. Data analytics also allows auditors to perform continuous monitoring of transactions and controls. This real-time oversight helps in the early detection and remediation of issues, reducing risks and enhancing the overall governance framework. For instance, in a large corporation, continuous monitoring through data analytics can help detect discrepancies in payroll processing or procurement activities, allowing for timely interventions. Another critical advantage of data analytics in auditing is the ability to provide more detailed and actionable insights. Traditional audit methods often result in high-level findings that may not fully address underlying issues. In contrast, data analytics can pinpoint specific areas of concern, enabling auditors to make more targeted recommendations. For example, if an analysis reveals a consistent pattern of delayed supplier payments, the auditor can recommend specific process improvements to enhance payment efficiency.
The role of data analytics in risk management is also significant. By analyzing historical data, auditors can identify risk patterns and predict future risks. This predictive capability is valuable in dynamic and complex environments like financial institutions or large multinational corporations. For example, a bank can use data analytics to predict potential credit risks in its loan portfolio, allowing it to take proactive measures to mitigate them. Moreover, data analytics fosters a culture of continuous improvement within organizations. Data analytics encourages organizations to continuously refine their processes and controls by providing detailed insights and identifying areas for enhancement. This improvement aligns with principles of good governance and accountability, which are essential in the public and private sectors.
However, the integration of data analytics into auditing is challenging. Auditors must possess specific technical expertise and be familiar with analytical tools. Training and development programs are essential to equip auditors with the necessary skills. Furthermore, organizations must invest in robust IT infrastructure to support data analytics initiatives. This includes secure data storage, advanced analytical software, and data protection measures to ensure the confidentiality and integrity of data. The shift toward data-driven auditing also raises questions about the role of human judgment. While data analytics can process and analyze vast amounts of data, interpreting results and decision-making require professional judgment. Auditors must balance analytical tools with their expertise and intuition to provide a comprehensive assessment.
Types of Analytics: Descriptive, Diagnostic, Predictive, and Prescriptive
In internal auditing, leveraging data analytics—descriptive, diagnostic, predictive, and prescriptive—can significantly enhance the audit process. Each type of analytic serves a specific purpose, providing unique insights that help auditors perform their roles more effectively and efficiently. Let’s explore these techniques further.
Descriptive Analytics
Descriptive analytics is the most basic form of data analytics. It involves summarizing historical data to understand what has happened over a given period. In an audit context, descriptive analytics can help auditors identify trends, patterns, and anomalies in financial and operational data. For example, using descriptive analytics, an auditor can analyze a company’s financial statements over the past five years to identify revenue, expenses, and profit trends.
Descriptive analytics can be beneficial for compliance reporting. Auditors can use it to generate summaries of financial transactions, compliance activities, and operational performance, providing stakeholders with clear and concise overviews. This helps ensure transparency and accountability, which are critical in maintaining public trust and meeting regulatory requirements.
Diagnostic Analytics
While descriptive analytics tells us what happened, diagnostic analytics delves into why it happened; this type of analytics involves deeper data exploration to identify the causes of trends and anomalies identified by descriptive analytics. Diagnostic analytics often involves drill-down, data mining, and correlation analysis.
For instance, if descriptive analytics reveals a significant drop in sales during a particular quarter, diagnostic analytics can help auditors investigate the underlying reasons. This might involve analyzing customer feedback, market conditions, and internal processes to pinpoint the factors contributing to the decline. Diagnostic analytics can aid auditors in understanding complex compliance issues and operational inefficiencies, providing actionable insights for corrective measures.
Predictive Analytics
Predictive analytics uses historical data to forecast future events. It employs statistical models and machine learning algorithms to predict future outcomes based on past trends. Predictive analytics can anticipate potential risks and opportunities in auditing, enabling proactive decision-making.
For example, an auditor might use predictive analytics to forecast cash flow for the upcoming year based on historical financial data, seasonal trends, and market indicators. In a public sector organization, predictive analytics can help forecast budget needs, identify potential financial shortfalls, and plan for future resource allocation. This forward-looking approach enhances the audit function’s ability to support strategic planning and risk management.
Prescriptive Analytics
Prescriptive analytics predicts future outcomes and recommends actions to achieve desired results. It combines data, algorithms, and machine learning to provide actionable advice. Prescriptive analytics is particularly valuable in decision-making processes, helping auditors and management teams determine the best action.
Prescriptive analytics might optimize resource allocation across various departments in an audit scenario. For instance, if predictive analytics forecasts a potential budget deficit, prescriptive analytics can suggest cost-cutting measures or alternative revenue strategies. In the healthcare sector, prescriptive analytics can guide decisions on resource distribution, staffing levels, and patient care strategies to improve efficiency and outcomes.
Integrating the Different Types of Analytics
Integrating these four types of analytics into the auditing process requires a strategic approach. Auditors must start with descriptive analytics to understand current status and then use diagnostic analytics to investigate underlying issues. Predictive analytics helps forecast future scenarios, and prescriptive analytics provides actionable recommendations. For example, in a manufacturing company, auditors might start with descriptive analytics to summarize production data and identify trends in output and quality. Diagnostic analytics can then explore the causes of production issues, such as machine breakdowns or supply chain disruptions. Predictive analytics can forecast future production levels and identify potential bottlenecks, while prescriptive analytics can recommend optimal maintenance schedules and supply chain strategies to enhance efficiency.
Descriptive, diagnostic, predictive, and prescriptive analytics are vital in modern auditing. By leveraging these different types of analytics, auditors can gain comprehensive insights into past performance, understand underlying issues, anticipate future challenges, and receive actionable recommendations. This holistic approach enhances the effectiveness and efficiency of audits and supports better governance, risk management, and strategic planning in organizations. As the field of internal auditing continues to evolve, integrating these advanced analytical techniques will be crucial in driving continuous improvement and delivering more excellent value to stakeholders.
Integration of Analytics—Common Challenges
While the benefits of integrating analytics into auditing are significant, there are also challenges to consider. These include data quality and accessibility, the need for advanced analytical skills, and the integration of analytics tools with existing audit processes. Auditors must ensure that data is accurate, complete, and reliable to draw meaningful conclusions. Additionally, ongoing training and professional development are essential to equip auditors with the necessary skills to leverage these advanced analytical techniques. Where regulatory standards and stakeholder expectations are high, successfully adopting analytics in auditing can enhance compliance, improve operational efficiency, and support strategic decision-making. Organizations must invest in robust IT infrastructure, advanced analytical tools, and continuous training to fully realize the potential of data analytics in auditing.
Tools and Technologies for Implementing Analytics in Auditing
Implementing analytics in auditing requires a combination of software, platforms, and methodologies designed to handle complex data analysis tasks efficiently. These tools enhance the accuracy and depth of audits and enable auditors to perform their duties more effectively and confidently.
Integrating advanced tools and technologies in audit analytics enhances the internal audit function by providing deeper insights, improving accuracy, and enabling continuous monitoring. From data extraction and visualization to advanced statistical analysis and machine learning, these tools empower auditors to perform their roles more effectively. As organizations navigate an increasingly complex regulatory landscape, leveraging these technologies is essential for ensuring compliance, improving governance, and delivering more excellent value through the audit process.
Common Tools Used for Audit Data Analytics
Data Extraction and Preparation Tools
Data extraction and preparation is the first step in any data analytics process. Tools like Microsoft Excel, Python, and R are commonly used. With its powerful data manipulation capabilities and user-friendly interface, Excel remains a staple in audit analytics. Python and R, on the other hand, offer more advanced data processing capabilities. Python, with libraries like Pandas and NumPy, and R, with its extensive packages for statistical analysis, allow auditors to handle large datasets and perform complex calculations efficiently. These tools are handy in organizations where auditors often deal with vast financial and operational data.
Data Visualization Tools
Visualizing data effectively is crucial for identifying patterns, trends, and anomalies. Tools like Tableau, Power BI, and QlikView are popular choices for data visualization in auditing. Tableau offers robust features for creating interactive and shareable dashboards, making it easier for auditors to present their findings to stakeholders. Integrated with Microsoft’s ecosystem, Power BI provides seamless data connectivity and powerful visualization capabilities. QlikView is known for its associative data model, which helps auditors explore data from multiple angles. These tools enable auditors to convert complex data into easily understandable visual formats, enhancing transparency and facilitating better decision-making.
Statistical Analysis Tools
SAS, SPSS, and Stata are invaluable for more sophisticated statistical analysis. SAS (Statistical Analysis System) is widely used for advanced, multivariate, and predictive analytics. SPSS (Statistical Package for the Social Sciences) is particularly popular in social science research but is also applicable in auditing for performing complex statistical tests. Stata is another powerful tool that provides comprehensive data management and statistical capabilities. These tools help auditors conduct detailed analyses, identify correlations, and test hypotheses, which are crucial for thorough audit investigations.
Audit Management Software
Audit management software like TeamMate, ACL Analytics, and CaseWare IDEA are essential to streamline the audit process and integrate analytics seamlessly. TeamMate provides a comprehensive suite of tools for planning, executing, and reporting audits. ACL Analytics (now part of Galvanize, a Diligent brand) offers robust data analysis capabilities designed explicitly for audit purposes, including anomaly detection and risk assessment. CaseWare IDEA is known for its ability to handle large datasets and perform various audit functions, from data analysis to visualization. These platforms facilitate data analytics and enhance overall audit management, ensuring audits are conducted efficiently and effectively.
Big Data and Machine Learning Platforms
Big data platforms like Hadoop and Apache Spark have become crucial in audit analytics as data volumes grow. Hadoop’s distributed storage and processing capabilities allow auditors to handle massive datasets that traditional tools cannot manage. Apache Spark offers fast in-memory processing and is particularly useful for iterative machine-learning tasks. Machine learning platforms like TensorFlow and Scikit-learn enable auditors to develop predictive models and uncover insights from complex data patterns. These technologies are particularly relevant in sectors like finance and healthcare, where internal auditors often deal with extensive and intricate datasets.
Cloud-Based Analytics
Cloud computing has revolutionized data analytics by providing scalable and flexible solutions. Cloud platforms like Amazon Web Services (AWS), Microsoft Azure, and Google Cloud Platform (GCP) offer various analytics services, including data storage, processing, and machine learning. AWS provides tools like Redshift for data warehousing and SageMaker for building machine learning models. Microsoft Azure offers services like Azure Machine Learning and Power BI for comprehensive analytics solutions. Google Cloud Platform provides BigQuery for large-scale data analysis and AutoML for creating custom machine learning models. These cloud-based tools allow auditors to scale their analytics efforts quickly and access advanced technologies without significant upfront investment.
Blockchain and AI Technologies
Emerging technologies like blockchain and AI are also making inroads into audit analytics. Blockchain offers a secure and transparent way to record transactions, which can be particularly useful for verifying the integrity of financial data. AI technologies, including natural language processing (NLP) and computer vision, can automate routine audit tasks, such as document review and anomaly detection. For instance, AI-powered tools can scan large volumes of contracts and financial statements, extracting relevant information and identifying inconsistencies much faster than manual methods.
Use of Audit Data Analytics Tools—Common Challenges
While these tools and technologies offer significant advantages, their implementation comes with challenges. Data privacy and security are paramount in areas where regulations like the Personal Information Protection and Electronic Documents Act (PIPEDA) impose strict data protection requirements. Auditors must ensure that data analytics tools comply with these regulations to safeguard sensitive information. Moreover, successfully adopting these technologies requires ongoing training and skill development. Auditors need to be proficient in using these tools and understanding their outputs. Organizations must invest in continuous professional development to keep their audit teams updated on the latest advancements in audit analytics.
Developing an Analytics-Driven Audit Approach
Developing an analytics-driven audit approach involves integrating data analytics into the entire audit process, transforming how audits are conducted and enhancing their effectiveness and efficiency. This approach leverages the power of data to provide deeper insights, identify risks more accurately, and offer more robust recommendations. For internal auditors, adopting an analytics-driven approach is crucial for meeting regulatory requirements, improving transparency, and adding value to the organization.
Objectives, Scope, and Data Collection
The first step in developing an analytics-driven audit approach is clearly defining the objectives and scope of the audit. This involves understanding the specific goals of the audit, the key areas to be examined, and the expected outcomes. For example, the audit might focus on evaluating the effectiveness of internal controls, assessing compliance with regulatory standards, or identifying potential areas of financial fraud. By clearly defining the objectives and scope, auditors can determine the types of data required and the appropriate analytical techniques. Once the goals and scope are established, the next step is data collection and preparation. This involves gathering relevant data from various sources within the organization, such as financial records, operational reports, and compliance documents. Data preparation includes cleaning and organizing the data to ensure accuracy and consistency. Tools like Microsoft Excel, Python, and data preparation platforms can help automate this process, saving time and reducing errors. Internal auditors must also ensure that data collection and preparation comply with data privacy regulations, such as the PIPEDA.
Analytical Tools and Techniques
Choosing the appropriate analytical tools is crucial for an effective analytics-driven audit. The choice of tools depends on the complexity of the data and the specific analytical requirements. Commonly used tools include Tableau and Power BI for data visualization, SAS and SPSS for statistical analysis, and ACL Analytics and CaseWare IDEA for audit-specific data analysis. These tools enable auditors to analyze large datasets, identify patterns and anomalies, and visualize results in an easily interpretable format. Auditors can apply various analytical techniques to extract insights with the data prepared and the tools selected. Descriptive analytics helps summarize historical data, clearly showing past performance and identifying trends. Diagnostic analytics explores the underlying causes of observed patterns and anomalies. Predictive analytics uses historical data to forecast outcomes, allowing auditors to anticipate potential risks and opportunities. Prescriptive analytics provides actionable recommendations based on the analysis, helping organizations make informed decisions.
Integrating data analytics into traditional audit procedures involves embedding analytical techniques throughout the audit process. During the planning phase, auditors can use analytics to identify high-risk areas and prioritize audit activities. For instance, predictive models can highlight transactions or accounts that are more likely to contain errors or fraud.
Findings and Recommendations
Auditors can apply diagnostic and prescriptive analytics during fieldwork to investigate identified issues and develop recommendations. Finally, in the reporting phase, data visualization tools can help present findings clearly and concisely, making it easier for stakeholders to understand and act on the results. An analytics-driven audit approach also enables continuous monitoring and real-time auditing. By integrating data analytics with the organization’s information systems, auditors can continuously monitor transactions and controls, detecting issues as they arise. This proactive approach allows for timely interventions, reducing the risk of significant undetected problems. For example, continuous monitoring of financial transactions can identify unusual patterns indicative of fraud, enabling immediate investigation and corrective action. Real-time auditing in the public sector can help ensure ongoing compliance with regulatory standards, enhancing transparency and accountability.
Continuous Professional Development
Implementing an analytics-driven audit approach requires auditors to develop new skills and expertise. Continuous training and professional development are essential to equip auditors with the knowledge and skills to use advanced analytical tools and techniques effectively. Organizations should invest in training programs, workshops, and certifications in data analytics, statistical analysis, and data visualization. This investment not only enhances the capabilities of the audit team but also ensures that the organization can fully leverage the benefits of an analytics-driven audit approach.
Analytics-driven Audit Approach—Common Challenges
While the benefits of an analytics-driven audit approach are significant, there are also challenges. Data quality and accessibility can be major issues, as incomplete or inaccurate data can undermine the effectiveness of the analysis. Organizations must establish robust data governance practices to ensure data integrity and reliability. Another challenge is the potential resistance to change within the audit team and the broader organization. Communicating the benefits of analytics-driven auditing and involving stakeholders in the implementation process is essential to overcome this.
Integrating Analytics into Traditional Audit Processes
Integrating analytics into traditional audit processes enhances the depth and breadth of audits, making them more effective and efficient. For internal auditors, this integration is crucial for maintaining compliance, improving governance, and providing more valuable insights to stakeholders. Here, we explore the steps and considerations in seamlessly embedding analytics into the traditional audit framework.
Recognizing the Benefits of Analytics in Internal Audits
The first step in integrating analytics into traditional audit processes is recognizing the benefits. Analytics transforms data into actionable insights, enabling auditors to identify patterns, trends, and anomalies that might be overlooked using traditional methods. It allows for a more comprehensive examination of entire data sets rather than limited samples, increasing the likelihood of detecting errors and fraud. Additionally, analytics enhances the ability to perform continuous monitoring, providing real-time insights and early warnings about potential issues. Integrating analytics begins at the audit planning stage. Traditional audit planning involves assessing risks and determining the scope of the audit. With analytics, this process becomes more data-driven. Auditors can use descriptive and diagnostic analytics to analyze historical data, identifying areas with high-risk patterns. Predictive analytics can forecast potential risks, allowing auditors to prioritize areas requiring more attention. For instance, in a financial institution, analytics can help identify accounts or transactions with a higher likelihood of fraud based on past data trends.
Data Collection, Preparation, and the Application of Analytics
Data collection and preparation are critical for the effective integration of analytics. Traditional audits rely heavily on manually collected data samples, which can be time-consuming and prone to errors. By using data extraction tools and technologies such as Extract, Transform, Load processes or ELT processes, auditors can automate data collection from various sources within the organization. This ensures a more comprehensive and accurate data set. In Canada, where data privacy laws such as PIPEDA are stringent, auditors must ensure that data collection complies with legal requirements and protects sensitive information. Once data is collected and prepared, auditors can apply various analytical techniques throughout the audit process. Descriptive analytics summarizes historical data, helping auditors understand past performance and identify trends. Diagnostic analytics delves deeper into the data, identifying the root causes of any anomalies or issues. Predictive analytics forecasts future trends and risks, allowing auditors to anticipate problems and take proactive measures. Prescriptive analytics goes a step further by recommending actions based on the data analysis, guiding auditors in making informed decisions.
Sufficient and Appropriate Evidence Gathering
During fieldwork, analytics can significantly enhance the evidence-gathering process. Traditional methods often involve the manual testing of a small sample of transactions. Auditors can test entire data populations with analytics, increasing the chances of detecting irregularities. For example, in auditing payroll processes, analytics can examine all payroll transactions over a period, identifying discrepancies or unusual patterns. This comprehensive approach not only improves the accuracy of the audit but also saves time and resources. Effective communication of audit findings is crucial, and data visualization plays a vital role. Traditional audit reports can be lengthy and complex, making it challenging for stakeholders to grasp the key points. Auditors can present their findings in a more engaging and understandable format using data visualization tools like Tableau or Power BI. Visual representations such as graphs, charts, and dashboards make it easier to highlight significant issues and trends for readers. For instance, a bar chart can effectively show compliance issues across different departments, making it clear where the organization needs to focus its efforts.
Continuous Monitoring and Auditing
One of the most significant advantages of integrating analytics into traditional audit processes is the ability to perform continuous monitoring and real-time auditing. Traditional audits are typically periodic and conducted at specific intervals. Constant monitoring, enabled by analytics, allows auditors to track transactions and controls in real time, providing ongoing assurance and early detection of issues. This proactive approach is particularly beneficial in dynamic and high-risk environments such as financial services and healthcare, where timely intervention can prevent significant problems. Integrating analytics into traditional audit processes requires auditors to develop new skills and expertise. Traditional auditors may need training in data analytics tools and techniques to use these technologies effectively. Organizations should invest in continuous professional development programs to ensure their audit teams have the necessary skills. This training should cover various aspects of data analytics, including data collection and preparation, analytical techniques, and data visualization.
Integration of Analytics—Common Challenges
While integrating analytics offers numerous benefits, it also presents challenges that must be addressed. One of the main challenges is ensuring data quality and reliability. Only accurate or complete data can lead to correct conclusions. Therefore, robust data governance practices are essential to maintain data integrity. Another challenge is resistance to change within the audit team. Some auditors may be hesitant to adopt new technologies and methodologies. Clear communication of the benefits and adequate training and support can help overcome this resistance.
Challenges and Solutions in Adopting Audit Analytics
Adopting audit analytics presents numerous opportunities for enhancing the internal audit function. However, transitioning from traditional audit methods to an analytics-driven approach takes time and effort. These challenges range from data quality issues and technological limitations to resistance to change within the audit team.
Data Quality, Integrity, and Accessibility
One of the primary challenges in adopting audit analytics is ensuring the quality and accessibility of data. Poor data quality can lead to inaccurate analyses and conclusions. Incomplete, outdated or conflicting data may hamper the effectiveness of analytics. Furthermore, data is often stored in disparate systems, making it difficult to consolidate and analyze comprehensively. To address data quality issues, organizations should establish robust data governance frameworks. This includes defining data standards, implementing data cleansing processes, and ensuring regular updates. Data accessibility can be improved by integrating data from various sources into a centralized data warehouse. This consolidation allows auditors to access a unified data set, facilitating more comprehensive and accurate analyses.
Technological Limitations and Integration
Another significant challenge is the technological limitations and integration issues with the analytics tools adopted by the audit team. Traditional audit teams may need more infrastructure to support advanced analytics. Integrating new analytics tools with existing systems can be complex and time-consuming, requiring significant IT support and investment. Organizations should assess their current IT infrastructure to overcome these technological challenges and identify gaps that need addressing. Investing in scalable and flexible analytics platforms can help ensure that the technology adapts to changing audit needs. It is crucial to collaborate with IT departments to ensure smooth integration of data analytic tools into existing systems. Additionally, leveraging cloud-based solutions can provide computational power and storage without significant upfront investment.
Developing Relevant Competencies
The shift to analytics-driven auditing requires auditors to develop new skills and competencies. Traditional auditors may need more expertise to use advanced analytics tools effectively. This skills gap can hinder the adoption of analytics and reduce the overall effectiveness of the audit function. Organizations can bridge this skills gap by investing in continuous training and professional development for their audit teams. Training programs should cover various aspects of data analytics, including data collection, statistical analysis, and visualization. Partnering with academic institutions and professional bodies to offer certifications and advanced courses in audit analytics can also help enhance the skills of auditors. Hiring data scientists or analytics experts to work alongside traditional auditors can also provide the necessary expertise and facilitate knowledge transfer within the team.
Resistance to Change and Tone at the Top
Resistance to change is a common challenge when adopting new technologies and methodologies. Auditors accustomed to traditional audit practices may be reluctant to embrace analytics-driven approaches. This resistance can stem from a fear of the unknown, concerns about job security, or skepticism about the benefits of analytics. Addressing resistance to change requires clear communication and strong leadership. Leaders should articulate the benefits of adopting audit analytics, emphasizing how it enhances audit quality and efficiency. Involving auditors in the planning and implementation process can also help mitigate resistance. Providing ongoing support and resources, such as training and access to analytics tools, can ease the transition and build confidence in the new approach. Highlighting success stories and quick wins where analytics has led to significant improvements can also help reinforce the value of the change.
Limited Resources
Implementing audit analytics can be resource-intensive, requiring significant financial investment and allocation of personnel. Smaller organizations, in particular, may need help with the costs of acquiring analytics tools, training staff, and upgrading IT infrastructure. Organizations can adopt a phased implementation approach to address cost and resource constraints. Starting with a pilot project can help demonstrate the value of analytics without requiring a substantial initial investment. Leveraging open-source analytics tools and cloud-based solutions can also reduce costs. Additionally, organizations can explore partnerships with external analytics service providers, which can offer expertise and technology on a pay-as-you-go basis, reducing the need for substantial upfront investment.
Data Governance and Compliance
Compliance with data privacy regulations such as PIPEDA is critical when adopting audit analytics. Ensuring that data analytics practices comply with these regulations is essential to avoid legal repercussions and maintain stakeholder trust. Organizations should develop clear policies and procedures for data governance, ensuring that data collection, storage, and analysis comply with relevant regulations. Regular audits and reviews of data practices can help identify and address compliance issues. Engaging legal and compliance experts in the planning and implementation of audit analytics can also provide valuable guidance and ensure adherence to regulatory requirements.
Data Security
Data security is another significant concern when adopting audit analytics. Sensitive financial and operational data must be protected from unauthorized access and breaches. The increased use of digital tools and platforms can introduce new security vulnerabilities. Organizations should implement robust cybersecurity measures to ensure data security, including encryption, access controls, and regular security audits. Training auditors on data security best practices and fostering a culture of cybersecurity awareness is also essential. Partnering with IT and cybersecurity experts can help develop and maintain a secure analytics environment.
Internal Audit in Action
Background
Li Senior HealthCare Group, a network of hospitals and clinics, faced challenges in detecting and preventing fraudulent activities within its billing and procurement processes. The internal audit department leveraged analytics to enhance its fraud detection capabilities.
Challenge
The primary challenge was implementing a fraud detection analytics system that could analyze complex datasets to identify potential fraud patterns and anomalies without disrupting legitimate operations.
Action Taken
- Focus on Diagnostic and Predictive Analytics: The audit team concentrated on deploying diagnostic analytics to identify the root causes of known fraud incidents and predictive analytics to spot potential future fraud.
- Implementation of Tools and Technology: Specialized fraud detection software was introduced, employing machine learning algorithms capable of analyzing vast transaction data.
- Integration of Analytics: The fraud detection analytics initiative was carefully integrated with existing audit processes, ensuring auditors could seamlessly use analytics insights to guide inquiries and investigations.
- Challenges and Solutions: Initial challenges, including data quality issues and false positives in fraud detection, were addressed through continuous refinement of the analytics models and close collaboration with the IT department.
- Success Stories: The initiative led to the detection of several significant fraud schemes that had previously gone unnoticed, demonstrating the value of analytics in enhancing the audit function’s effectiveness.
Outcome
Li Senior HealthCare Group’s implementation of fraud detection analytics significantly improved its ability to identify and investigate fraudulent activities, resulting in substantial cost savings and enhanced operational integrity. The initiative bolstered the organization’s fraud prevention efforts and served as a benchmark for analytics-driven auditing within the Li Senior HealthCare sector.
Reflection
This scenario highlights the decisive role of analytics in modern internal auditing, particularly in fraud detection. Li Senior HealthCare Group’s successful integration of diagnostic and predictive analytics demonstrates how targeted analytics initiatives can address specific risks, such as fraud, providing auditors with the insights needed to protect the organization’s assets and reputation.
Key Takeaways
Let’s recap the concepts discussed in this section by reviewing these key takeaways:
- Data analytics enhances audit accuracy, efficiency, and effectiveness by transforming raw data into actionable insights.
- Descriptive, diagnostic, predictive, and prescriptive analytics provide a comprehensive framework for analyzing and improving audit processes.
- Implementing advanced tools and technologies is essential for effective data collection, analysis, and visualization in auditing.
- Developing an analytics-driven audit approach involves careful planning, skill development, and integration with traditional methods.
- Real-world success stories highlight the transformative impact of analytics in various sectors, demonstrating improved fraud detection, compliance, and operational efficiency.
Knowledge Check
Review Questions
- How does data analytics enhance the effectiveness of internal auditing?
- What are the four types of analytics used in internal auditing, and what is the primary focus of each?
- What challenges might an organization face when integrating analytics into its traditional audit processes?
- How can the success of implementing audit analytics be demonstrated through real-world examples?
- Why is continuous training and professional development necessary for auditors in an analytics-driven audit environment?
Essay Questions
- How can integrating data analytics into internal auditing enhance the accuracy and efficiency of audit processes, and what are the potential limitations of relying on analytics?
- How can adopting predictive analytics transform risk management practices in internal auditing, and what are the challenges associated with implementing predictive models?
- Discuss the role of continuous auditing in improving internal controls and compliance within organizations. What are the benefits and potential drawbacks of constant auditing?
Mini Case Study
Brand Electronics Corporation is a mid-sized manufacturing company based in Canada that produces consumer electronics. The company has been experiencing rapid growth over the past few years, leading to increased complexity in its operations. Brand Electronics has decided to leverage data analytics to manage this growth effectively and enhance its internal auditing processes. The internal audit team at Brand Electronics Corporation is tasked with integrating analytics into their traditional audit practices to improve risk management, operational efficiency, and compliance. The team faces several challenges, including data quality issues, resistance to change from staff, and the need for significant investment in new technology and training.
Challenges:
- Data Quality and Accessibility:
- The audit team finds that data across different departments is inconsistent and not standardized.
- There are gaps in the data, and some historical data is missing or incomplete.
- Resistance to Change:
- Some staff members hesitate to adopt new technologies and prefer traditional auditing methods.
- There is a lack of understanding of how analytics can benefit the audit process.
- Investment in Technology and Training:
- Implementing advanced analytics tools requires a significant financial investment.
- The team needs training to develop the necessary skills to use these tools effectively.
- Integration with Traditional Audit Processes:
- The team must find ways to integrate analytics into their existing audit workflows.
- There is a need to balance traditional audit methods with new data-driven approaches.
Required:
- How should the internal audit team address data quality and accessibility issues to ensure accurate and reliable analytics?
- What strategies can the audit team use to overcome resistance to change among staff members?
- How can Brand Electronics justify the investment in new technology and training for analytics-driven auditing?
- How can the internal audit team integrate analytics into traditional processes?
The process of examining, cleaning, transforming, and modeling data to discover useful information, draw conclusions, and support decision-making.
The process of examining data sets to draw conclusions, identify patterns, and support decision-making using statistical and computational techniques.
Specific goals that an audit aims to achieve, such as evaluating the effectiveness of controls, ensuring compliance, and identifying areas for improvement.
Problems or deficiencies in data accuracy, completeness, consistency, or reliability, leading to errors, inconsistencies, or inefficiencies in data analysis, reporting, or decision-making processes within organizations.
Proficiency in using analytical tools, techniques, and methodologies to interpret and analyze data, extract insights, and make informed decisions, essential for professionals in various fields, including auditing and business analytics.
Software programs or applications used to analyze, process, and visualize data, providing capabilities for statistical analysis, data mining, visualization, and reporting to derive insights and support decision-making.
Irregularities, deviations, or unexpected patterns observed in data that do not conform to normal or expected behaviour, indicating errors, inconsistencies, or potential issues requiring further investigation or analysis.
Software applications or platforms used to analyze, process, and visualize data, providing capabilities for data mining, statistical analysis, visualization, and reporting to derive insights and support decision-making.
The ongoing process of collecting and analyzing data to detect and respond to risks, ensure compliance, and improve processes in real time or near real time.
Incorporation of data analytics capabilities, tools, or processes into organizational systems, operations, or decision-making processes to leverage data-driven insights to improve performance, innovation, and competitiveness.
Framework of hardware, software, networks, and facilities required to support the operations and activities of an organization's information technology systems and services effectively and efficiently.
Repository or database used to store, manage, and organize data assets securely, ensuring durability, availability, and scalability to support various applications, users, and business needs effectively.
Measures and processes implemented to safeguard personal and sensitive data from unauthorized access, alteration, or destruction, ensuring data integrity and security.
Decision-making based on expertise, experience, ethics, and relevant facts, allowing auditors to assess situations, make informed conclusions, and exercise discretion in audit engagements.
Systematic approach and procedures followed in conducting audit engagements, including planning, execution, reporting, and follow-up, to assess controls, risks, and compliance in organizations effectively and efficiently.
Analysis of historical data to understand past events, trends, or patterns, providing insights into what has happened and serving as a basis for further analysis or decision-making.
Examination of data to identify causes or reasons behind past events or trends, enabling organizations to understand why certain outcomes occurred and informing corrective actions or improvements.
Analysis of historical and current data using statistical models and algorithms to forecast future outcomes, trends, or behaviours, enabling proactive decision-making and risk mitigation strategies.
A type of data analysis that not only anticipates what will happen but also recommends actions to achieve desired outcomes or to manage potential future scenarios by using algorithms, simulations, optimization techniques, etc., to suggest specific actions based on data analysis and predictions.
Allocation and distribution of resources, such as personnel, funds, or equipment, to activities, projects, or tasks based on priorities, requirements, and availability to optimize utilization and achieve objectives.
Measure of the accuracy, completeness, consistency, and reliability of data, ensuring it meets the requirements of intended uses and supports informed decision-making and analysis.
Activities and programs designed to enhance the skills, knowledge, and competencies of professionals, ensuring they remain current in their field.
Methods, procedures, or approaches used to interpret and analyze data systematically, including statistical analysis, data mining, trend analysis, or predictive modeling, to uncover patterns, trends, or relationships.
Process of retrieving or pulling data from various sources, systems, or databases for analysis, reporting, or storage purposes, ensuring accuracy, completeness, and timeliness of data.
Presentation of data in visual formats such as charts, graphs, or dashboards, enhancing understanding, interpretation, and communication of complex information for better decision-making and insights.
Microsoft's business analytics tool that enables users to visualize and analyze data from various sources, create interactive reports, and share insights for informed decision-making.
The process of collecting, organizing, interpreting, and presenting data to discover patterns, relationships, and trends for decision-making.
Software solutions designed to streamline and automate audit processes, including planning, scheduling, documentation, workflow management, and reporting, enhancing efficiency, collaboration, and compliance in audit engagements.
Data analysis software used for auditing, fraud detection, and data analytics, offering tools for data visualization, manipulation, and extraction from various sources for investigative purposes.
Audit, risk, and compliance software used for data analysis, continuous monitoring, and forensic investigation, providing tools for identifying anomalies, detecting fraud, and ensuring data integrity.
Statistical or machine learning models used to forecast future outcomes or trends based on historical data and patterns, enabling proactive decision-making and risk mitigation strategies.
Regular or recurring structures, trends, or relationships observed within datasets, indicating systematic behaviours, correlations, or associations that can be analyzed for insights or predictions.
Amazon Web Services, a cloud computing platform providing a wide range of services and solutions for computing, storage, networking, databases, machine learning, and analytics on a pay-as-you-go basis.
Technologies that simulate human intelligence, such as machine learning, natural language processing, and computer vision to perform tasks, solve problems, and make decisions autonomously.
AI technology enabling computers to understand, interpret, and generate human language, facilitating tasks such as sentiment analysis, text summarization, and language translation for various applications.
Field of AI and computer science focused on developing systems that can interpret and analyze visual information from images or videos, enabling applications such as object recognition and image classification.
The Personal Information Protection and Electronic Documents Act, a Canadian law regulating the collection, use, and disclosure of personal information by private sector organizations.
Process of acquiring or improving abilities, expertise, or competencies through training, practice, and experience, enhancing an individual's capacity to perform tasks effectively and achieve professional growth.
Activities and processes performed to organize, clean, transform, or format raw data into a structured and usable format for analysis, ensuring consistency, integrity, and compatibility with analytical tools.
Process of gathering, acquiring, or retrieving data from various sources, systems, or documents, ensuring completeness, accuracy, and relevance for analysis, reporting, or decision-making purposes.
Specific tasks and techniques used by auditors to gather evidence, evaluate controls, and test the accuracy and completeness of financial statements.
Audit approach using technology and automation to collect, analyze, and report on audit data continuously and instantly, enhancing agility, efficiency, and responsiveness in auditing processes.
Framework of policies, procedures, and controls governing the management, usage, and integrity of data assets within an organization, ensuring compliance, security, and quality standards.
The accuracy, consistency, and reliability of data throughout its lifecycle, ensuring it is not altered or corrupted and remains trustworthy.
The phase of an audit where objectives, scope, and resources are determined, and a strategy is developed to guide the audit process effectively.
Patterns, tendencies, or changes observed over time in datasets, indicating consistent movements, behaviours, or developments that can be analyzed for insights or predictions.
Results or issues identified by auditors during the audit process, including any discrepancies, risks, or weaknesses in internal controls, which are communicated to management and stakeholders.
Centralized repository or database that stores structured, organized, and integrated data from various sources, enabling analysis, reporting, and decision-making across an organization.
Sophisticated techniques and methods used to analyze complex and large datasets, including predictive modeling, machine learning, and data mining, to uncover insights, patterns, or trends for decision-making purposes.
Measures and practices implemented to protect digital data from unauthorized access, disclosure, alteration, or destruction, ensuring confidentiality, integrity, and availability of information assets.
The practice of protecting systems, networks, and programs from digital attacks to ensure data integrity, confidentiality, and availability.