Chapter 08. Performing the Audit: Approach, Techniques, and Tools
08.03. Sampling Methods and Statistical Analysis
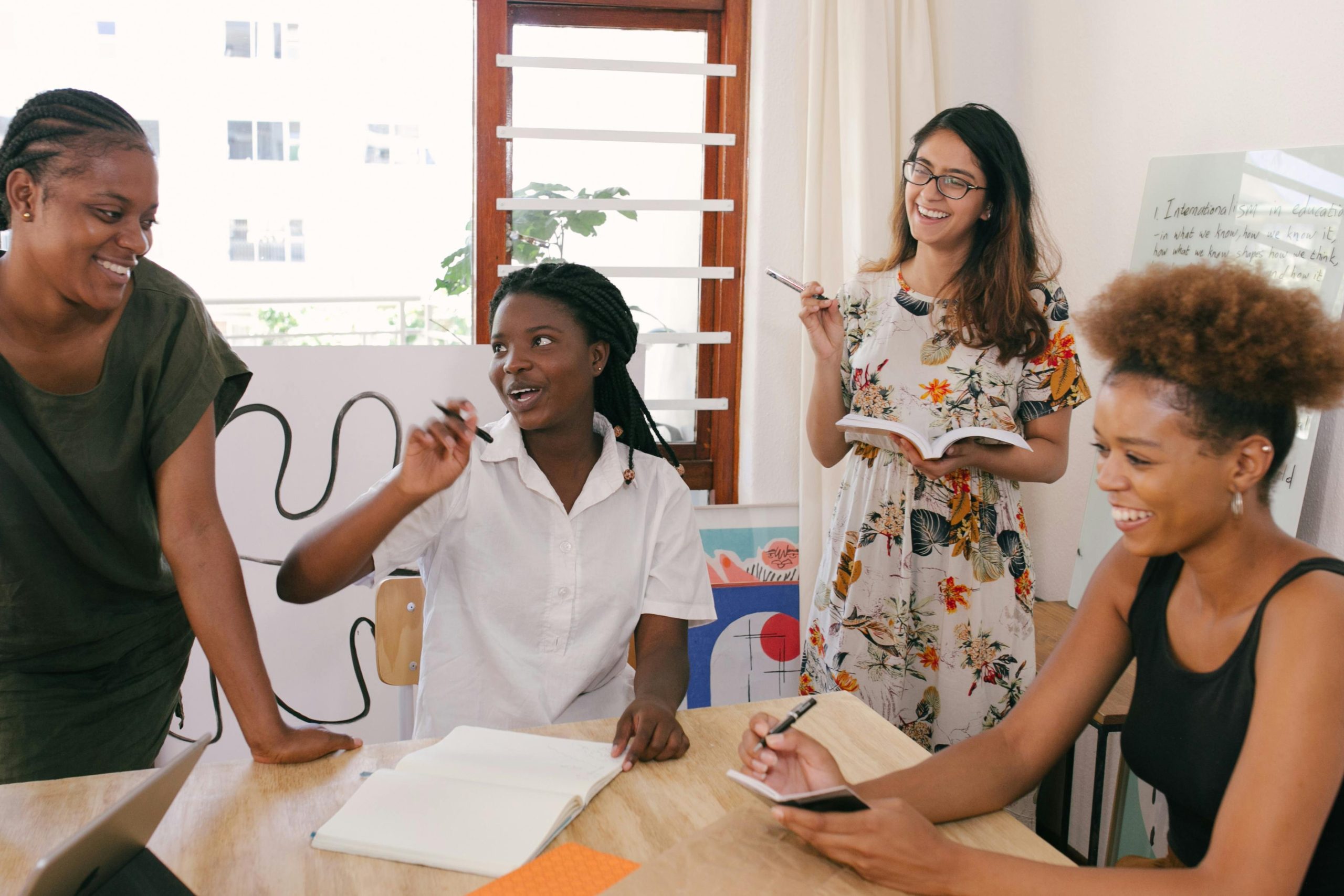
Key Questions
Briefly reflect on the following before we begin:
- Why is sampling used in auditing, and what are the key types of audit samples?
- How do auditors decide on the most appropriate sampling method for a particular audit?
- What statistical analysis techniques are commonly used in auditing, and for what purposes?
- How can auditors ensure their sampling and statistical analyses are robust and reliable?
This section delves into the fundamentals of audit sampling, which involves selecting a subset of data from a larger population to make conclusions about the entire population. Understanding the purpose and types of audit sampling is essential, as it enables auditors to determine the most appropriate sampling approach based on the objectives and characteristics of the audit engagement. Whether random, stratified, or judgmental sampling, each technique has unique advantages and applications in different audit scenarios.
Designing audit samples requires careful consideration of various factors, including the population size, homogeneity, and desired level of precision. Random sampling involves selecting items from the population without bias, while stratified sampling involves dividing the population into homogeneous subgroups to ensure adequate representation. On the other hand, judgmental sampling relies on the auditor’s expertise and judgment to select items based on their significance or risk. Furthermore, this section explores the application of statistical analysis techniques and tools in auditing, allowing auditors to analyze sample data, estimate population characteristics, and evaluate sampling risks and errors. Leveraging software for statistical analysis enhances the efficiency and accuracy of auditors, enabling them to conduct complex analyses and derive meaningful insights from audit samples.
Internal Audit in Action
Background
Rochdale Bank, a national banking institution, initiated an audit to detect potential fraud in its loan processing system after noticing irregularities in loan approval rates. With thousands of transactions processed monthly, the internal audit team needed to employ sampling methods and statistical analysis to effectively identify potentially fraudulent activities.
Challenge
The challenge was to design a sampling strategy that could accurately identify instances of fraud within the vast number of loan transactions, ensuring the audit was efficient and effective.
Action Taken
- Risk-based Sampling Design: The team employed a risk-based sampling approach, selecting a stratified random sample of transactions based on risk indicators such as loan amount, approval speed, and overrides of standard approval processes.
- Applying Statistical Analysis: Using statistical analysis tools, the auditors analyzed patterns and anomalies within the sampled transactions that could indicate fraudulent activity, such as unusually high approval rates for certain loan officers or patterns of overrides.
- Estimating Population Characteristics: The auditors extrapolated the characteristics of the broader population of transactions, including the likely prevalence of fraudulent activity, by analyzing the results gleaned from the sample.
- Evaluating Sampling Risks and Errors: The team carefully considered the risks of Type I (falsely identifying an issue where none exists) and Type II (failing to identify an actual problem) errors in their analysis, adjusting their approach to minimize these risks.
- Utilizing Software for Analysis: Specialized audit software was used to facilitate the statistical analysis, enabling more sophisticated analysis techniques and visualization of findings.
Outcome
Rochdale Bank’s audit successfully identified several instances of potential fraud that were not apparent without using risk-based sampling and statistical analysis. The audit’s findings led to a review and strengthening of the loan approval process, reducing the risk of fraud and ensuring the system’s integrity.
Reflection
This scenario highlights the power of combining sampling methods with statistical analysis in auditing, particularly for detecting fraud within large datasets. By employing a risk-based sampling approach and leveraging statistical tools, Rochdale Bank’s internal audit team was able to identify and address critical issues efficiently and effectively, thereby showcasing the essential role of these techniques in modern audit practices.
Basics of Audit Sampling: Purpose and Types
Sampling is a fundamental technique to gather evidence and draw conclusions about the entire population being audited. Audit sampling aims to obtain sufficient, relevant, and reliable evidence to support audit conclusions while optimizing time and resources. By understanding the basics of audit sampling and the various sampling methods available, auditors can tailor their sampling approach to meet the specific needs of each audit engagement and ensure the reliability and validity of their findings.
There are various audit sampling methods, each serving specific purposes based on the audit objectives and characteristics of the population under review. The primary purpose of audit sampling is to provide auditors with a representative subset of data from the larger population. This subset, known as the sample, is selected and analyzed to draw inferences about the entire population. By examining a sample rather than the whole population, auditors can save time and resources while obtaining meaningful insights into the population’s characteristics. There are two main types of audit sampling: statistical sampling and non-statistical (judgmental) sampling.
Statistical sampling involves using mathematical and statistical techniques to select samples and analyze the results. This method allows auditors to quantify sampling risks and errors and draw statistically valid conclusions about the population. On the other hand, non-statistical sampling relies on auditors’ judgment and experience to select samples based on factors such as risk, materiality, and relevance.
Designing audit samples is a critical aspect of the auditing process, as it determines the subset of data that will be analyzed to make conclusions about the entire population being audited. There are three main techniques for designing audit samples: random, stratified, and judgmental. Each technique has its own advantages and considerations.
In practice, auditors often use a combination of the three sampling techniques based on the specific characteristics of the population, the audit objectives, and the resources available.
Random Sampling
Random sampling is a method where each item in the population has an equal chance of being selected for the sample. This technique is beneficial when auditors want to ensure that the sample is representative of the entire population and minimize bias. Random sampling helps achieve statistical validity and allows auditors to generalize the findings from the sample to the whole population with a known confidence level. However, random sampling may not be feasible or practical in certain situations, especially when the population is large or heterogeneous.
Stratified Sampling
Stratified sampling involves dividing the population into distinct subgroups or strata based on specific characteristics relevant to the objectives of the audit. Using random or systematic sampling techniques, samples are selected independently from each stratum. Stratified sampling allows auditors to ensure adequate representation of different population segments. It may improve the efficiency of the sampling process by focusing resources on areas of higher risk or importance. This method is beneficial when significant variations within the population need to be accounted for in the audit analysis.
Judgmental Sampling
Judgmental sampling, or purposive or non-probability sampling, involves the subjective selection of samples based on the auditor’s judgment and expertise. In judgmental sampling, auditors intentionally select samples they believe most likely provide relevant and meaningful information for the audit objectives. This technique is commonly used when auditors have prior knowledge of specific areas of concern or want to focus on high-risk or material items within the population. While judgmental sampling can be efficient and targeted, it may introduce bias into the audit process if not carefully executed, and the results may not be statistically representative of the entire population.
Applying Statistical Analysis in an Audit: Common Techniques and Tools
Applying statistical analysis in auditing involves using various techniques and tools to analyze audit data and draw meaningful conclusions about the population being audited. These techniques help auditors identify patterns, trends, and anomalies within the data, allowing them to assess the reliability and integrity of financial information and detect potential errors or irregularities.
By applying these standard statistical analysis techniques and tools, auditors can enhance the effectiveness and efficiency of their audit procedures, identify areas of risk or concern, and provide valuable insights to stakeholders about the financial performance and integrity of the audited entity.
Some standard statistical analysis techniques and tools used in auditing include the following:
Descriptive Statistics
Descriptive statistics are used to summarize and describe the main features of a dataset. Measures such as mean, median, mode, standard deviation, and range provide insights into the central tendency, dispersion, and distribution of data. Descriptive statistics help auditors understand the characteristics of the population and identify any outliers or unusual patterns that may require further investigation.
Regression Analysis
Regression analysis is a statistical technique to explore the relationship between two or more variables. In auditing, regression analysis can help auditors identify correlations between financial variables and assess the impact of independent variables on dependent variables. For example, auditors may use regression analysis to examine the relationship between sales revenue and advertising expenses to determine the effectiveness of marketing campaigns.
Benford’s Law Analysis
Benford's Law, also known as the first-digit law, states that in many naturally occurring datasets, the leading digits of numbers are not evenly distributed but follow a logarithmic pattern, with smaller digits occurring more frequently than larger ones. Auditors can apply Benford’s Law analysis to financial data, such as invoice amounts, to detect potential anomalies or fraudulent activities. Deviations from the expected distribution may indicate the presence of irregularities that warrant further investigation.
Ratio Analysis
Ratio analysis involves calculating and interpreting financial ratios to assess an organization’s economic performance and position. Standard financial ratios include liquidity ratios, profitability ratios, and leverage ratios. Auditors use ratio analysis to evaluate the company’s financial health, identify trends over time, and compare performance against industry benchmarks and competitors. Significant deviations from industry norms or historical trends may signal potential areas of concern that require further scrutiny.
Sampling Theory
Sampling theory provides a framework for making inferences about a population based on a sample of data. Auditors use sampling theory to determine sample sizes, estimate population parameters, and evaluate the reliability of audit findings. Understanding sampling theory allows auditors to assess sampling risks and errors and ensure that audit procedures are appropriately designed and executed to achieve objectives.
Estimating Population Characteristics from Sample Data
Estimating population characteristics from sample data is a crucial aspect of audit sampling, allowing auditors to extrapolate conclusions about the entire population based on the information obtained from a representative sample. Several statistical techniques and formulas commonly used to estimate population characteristics include calculating the mean, the total and various proportions using the sample data.
One of the most fundamental estimators is the sample mean, which estimates the population mean or average. The sample mean is calculated by summing the values of all observations in the sample and dividing by the number of observations. The sample mean estimates the average value of the variable of interest in the population, assuming that the sample is representative and unbiased.
Another substantial estimator is the sample total, which is used to estimate the total value of a variable in the population. The sample total is calculated by summing the values of all observations. This estimate is beneficial when auditors need to estimate the total value of a financial account, such as accounts receivable or inventory, based on a sample of transactions or items.
Proportions are also commonly estimated from sample data, especially when auditors are interested in categorical variables or attributes. For example, auditors may use sampling to estimate the proportion of defective products in a manufacturing process or the proportion of transactions that comply with a specific control procedure. The sample proportion is calculated by dividing the number of observations containing the attribute of interest by the total number of observations in the sample.
Auditors can use statistical formulas and confidence intervals to calculate the margin of error or uncertainty associated with these estimators. Confidence intervals provide a range of values within which the proper population parameter will likely fall with a certain confidence level. By considering the precision and reliability of the estimates, auditors can make informed decisions and conclusions about the population characteristics based on the sample data.
Estimating population characteristics from sample data requires careful consideration of the sampling methodology, the sample size, and the data’s variability. Auditors can generate reliable and accurate estimates that support their audit conclusions and recommendations by employing appropriate statistical techniques and tools.
Evaluating Sampling Risks and Errors
Evaluating sampling risks and errors is essential in audit sampling to ensure the reliability and accuracy of audit conclusions. Sampling risks refer to the possibility that audit conclusions based on sample results may differ from what would be obtained if the entire population were examined. To evaluate sampling risks and errors, auditors must assess statistical and non-statistical factors, implement appropriate sampling methods, and exercise professional judgment throughout the audit process. There are two primary types of sampling risks: statistical and non-statistical.
Statistical Sampling Risks
Statistical sampling risk arises from the inherent variability in the sample data and the uncertainty associated with estimating population characteristics from the sample. The two main types of statistical sampling risks are:
- Type I Risk (Alpha Risk): Type I risk, also known as alpha risk or risk of incorrect rejection, occurs when auditors conclude that a material misstatement exists in the population based on the sample results when, in fact, it does not. This risk is controlled by setting the acceptable level of risk, typically denoted as alpha (α), which represents the probability of incorrectly rejecting a true null hypothesis.
- Type II Risk (Beta Risk): Type II risk, also known as beta risk or risk of incorrect acceptance, occurs when auditors fail to detect a material misstatement in the population based on the sample results when, in fact, it exists. This risk is controlled by determining the sample size and the level of assurance required, which affect the likelihood of detecting material errors or deviations.
Non-statistical Sampling Risks
On the other hand, non-statistical sampling risks are related to factors other than sample size and variability. These risks include:
- Selection Bias: Selection bias occurs when the sample is not representative of the population due to systematic errors or biases in the sampling process. For example, suppose auditors intentionally or unintentionally select items that are easier to audit or are more likely to contain errors. In that case, the sample results may be biased, leading to incorrect conclusions.
- Non-sampling Errors: Non-sampling errors occur during the audit process but are unrelated to sampling. These errors may arise from deficiencies in audit procedures, inadequate documentation, misinterpretation of evidence, or fraud. Non-sampling errors can affect the reliability of audit conclusions regardless of the sample size or sampling method used.
Utilizing Software for Statistical Analysis in Auditing
Utilizing software for statistical analysis in auditing enhances the efficiency, accuracy, and reliability of audit procedures, particularly in large-scale or complex audits where manual calculations may be time-consuming or prone to errors. Audit software offers various features and functionalities tailored to the specific needs of auditors, allowing them to perform sophisticated statistical analyses and interpret data more effectively.
It also enables auditors to import data from various sources, such as spreadsheets, databases, or accounting systems. The software provides tools for cleaning, validating, and preparing the data for analysis, including identifying outliers, missing values, and inconsistencies. By automating these tasks, auditors can streamline the data preparation process and ensure the accuracy and completeness of the dataset.
Audit software offers various statistical techniques and tools for data analysis and interpretation. These include descriptive statistics, hypothesis testing, regression analysis, correlation analysis, time-series analysis, and predictive modelling. Auditors can select the appropriate statistical methods based on the audit objectives, sample characteristics, and the nature of the data being analyzed.
It provides visualization tools like charts, graphs, dashboards, and heat maps to visually present audit findings and insights. These visualizations help auditors identify trends, patterns, and anomalies in the data more effectively and communicate findings to stakeholders clearly and concisely.
Additionally, audit software allows for the customization of reports and the generation of audit documentation, including audit working papers, summaries, and presentations.
One of the key benefits of using audit software for statistical analysis is automation, which reduces manual effort and increases audit efficiency. Audit software can automate repetitive tasks, such as data sampling, testing, and validation, saving auditors time and effort.
Moreover, software tools often include built-in templates, workflows, and macros that streamline audit processes and standardize procedures across engagements.
Audit software also helps ensure compliance with professional standards, regulatory requirements, and organizational policies by providing built-in controls, validations, and audit trails. The software facilitates adherence to auditing standards and best practices, such as the International Standards on Auditing (ISAs) and Generally Accepted Auditing Standards (GAAS).
Additionally, audit software supports quality assurance processes, including peer review, oversight, and continuous improvement initiatives.
Thus, utilizing software for statistical analysis in auditing enhances audit effectiveness, efficiency, and quality by enabling auditors to analyze data more comprehensively, identify risks and opportunities, and provide valuable insights to stakeholders. By leveraging advanced statistical techniques and tools, auditors can enhance their analytical capabilities, improve audit outcomes, and add value to the organizations they serve.
Internal Audit in Action
Background
TechHealth Innovations, a provider of electronic health records systems, faced regulatory scrutiny over its data privacy and security practices. The internal audit team proactively planned an audit covering thousands of patient records across multiple systems to assess compliance with health data protection regulations.
Challenge
The challenge was to ensure comprehensive audit coverage of TechHealth Innovations’ data handling practices without the impracticality of examining every patient record.
Action Taken
- Stratified Random Sampling: The audit team used stratified random sampling to select patient records for review, categorizing data by sensitivity levels and system modules to ensure all areas were proportionally represented.
- Statistical Analysis for Compliance: Analytical procedures were applied to the sampled records to identify non-compliance with data protection standards, such as unauthorized access or insufficient encryption.
- Population Inference: From the sample analysis, auditors inferred the overall compliance level of TechHealth Innovations’ data handling practices, identifying systemic issues that required attention.
- Software Utilization: Advanced audit software with statistical functions was used to automate the sampling process and facilitate the analysis, enhancing the audit’s efficiency.
- Sampling Risk Management: Throughout the process, the team remained cognizant of sampling risks, ensuring that the sample size and selection methodology were adequate to provide a reliable basis for their conclusions.
Outcome
The compliance review enabled TechHealth Innovations to identify areas where data protection practices fell short of regulatory requirements and best practices. Based on the audit findings, the company implemented targeted improvements to its systems and processes, significantly enhancing data security and regulatory compliance.
Reflection
TechHealth Innovations’ use of sampling and statistical analysis exemplified how these techniques could extend audit coverage and provide insights into compliance across large data populations. By carefully designing their sampling approach and applying statistical analysis, the audit team could draw meaningful conclusions about the company’s overall compliance posture, demonstrating the value of these methodologies in assessing compliance and managing risks in complex environments.
Key Takeaways
Let’s recap the concepts discussed in this section by reviewing these key takeaways:
- Audit sampling is essential for auditors to obtain a representative subset of data from which they can infer conclusions about the entire population. It includes statistical sampling using mathematical techniques and non-statistical sampling based on auditor judgment.
- Auditors use various sampling techniques such as random sampling, which ensures each item has an equal chance of selection; stratified sampling, which divides the population into subgroups; and judgmental sampling, based on auditor discretion.
- Audit software enhances these processes through data visualization and trend analysis tools.
- Auditors use statistical methods to extrapolate sample data findings to the entire population, considering factors like sample size, variability, and confidence level to ensure accuracy.
- Understanding and mitigating sampling risks and errors, including selection bias, is crucial for the reliability of audit findings. Advanced software minimizes these risks by automating sample selection and data analysis.
Knowledge Check
Review Questions
- What is the purpose of audit sampling, and what are the two main techniques?
- Explain the difference between random and judgmental sampling techniques in audit sampling.
- Describe one common statistical analysis technique used in auditing and explain its application.
- What factors should auditors consider when evaluating sampling risks and errors?
- How does audit software enhance the efficiency and effectiveness of statistical analysis in auditing?
Essay Questions
- Describe the difference between random, stratified, and judgmental sampling techniques in auditing. Provide examples of situations where each technique would be most appropriate.
- Explain the concept of sampling risk in auditing and discuss factors that can affect its magnitude. Provide strategies that auditors can use to mitigate sampling risk effectively.
A sampling method where the auditor uses their professional judgment to select sample items based on specific criteria or knowledge.
Metrics or signs that suggest the presence of risk within an organization, helping to identify and assess potential issues that may impact objectives.
Techniques used to select a subset of a population for analysis, allowing conclusions to be drawn about the entire population.
The use of random selection and probability theory to determine the sample size and evaluate the results of an audit test.
The use of an auditor's judgment to determine the sample size and select sample items without relying on random selection or probability theory.
The threshold or magnitude of an omission or misstatement of accounting information that could influence the economic decisions of users.
A sampling method where each item in the population has an equal chance of being selected, ensuring unbiased representation of the population.
A sampling method that divides the population into subgroups (strata) based on characteristics, then randomly selects samples from each subgroup.
The process of collecting, organizing, interpreting, and presenting data to discover patterns, relationships, and trends for decision-making.
Statistical techniques used to summarize and describe the main features of a data set, including measures of central tendency and variability.
A statistical method for estimating the relationships among variables, often used to make predictions or assess the impact of one variable on another.
A mathematical principle stating that in many naturally occurring datasets, the leading digit is more likely to be small, often used in fraud detection.
The use of financial ratios to evaluate relationships between different financial statement items, assessing an organization's performance, liquidity, and solvency.
The study of how to draw conclusions about populations based on data collected from a sample, ensuring the sample represents the population accurately.
The attributes and features of a population, such as size, demographics, and behaviour, used to understand and analyze the group as a whole.
A subset of data collected from a larger population, used to make inferences about the population's characteristics or behaviour.
A range of values derived from sample data that is likely to contain the true population parameter, expressed with a certain level of confidence.
Risks that an auditor's conclusions based on samples may differ from the conclusions they would reach if the entire population were examined.