03. Planning an IS Audit
03.05. Effective Audit Procedures – Sampling
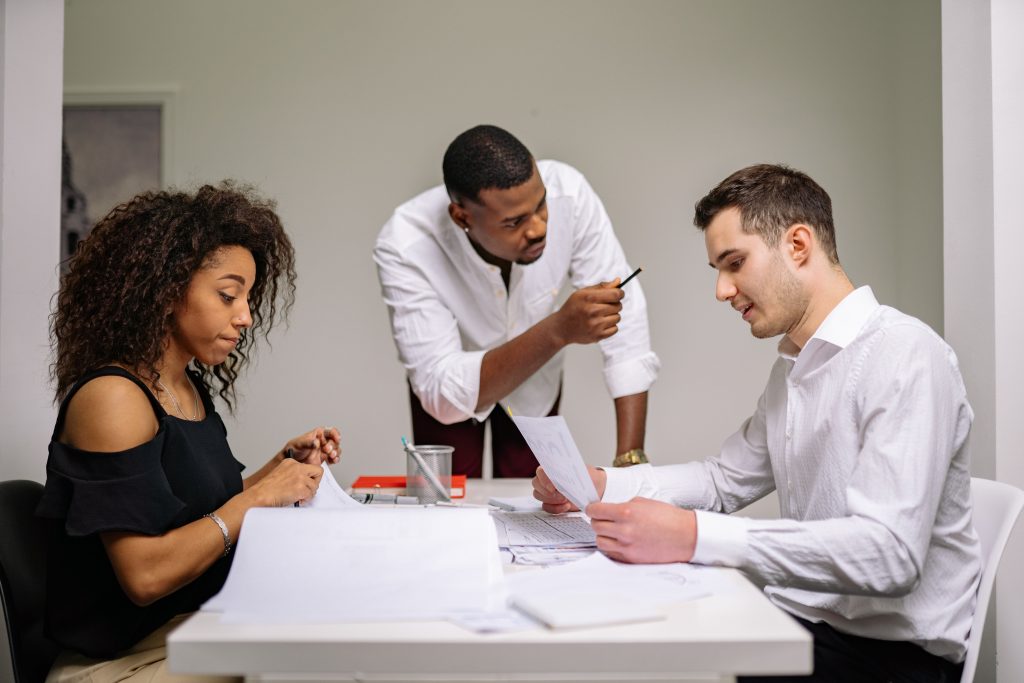
Briefly reflect on the following before we begin:
- Why do auditors often use sampling methods to gather evidence during audits?
- What are some common risks involved in selecting samples during an audit?
- How would an IS Auditor go about selecting samples during an audit?
In this section, we will explore the diverse range of sampling techniques available to IS auditors. We will do so by differentiating between statistical and non-statistical sampling methods, highlighting their respective advantages and appropriate contexts of use. We will explore judgmental sampling, a critical method where the auditor’s professional judgment plays a pivotal role in sample selection; random sampling techniques, which are fundamental to reducing bias and ensuring representativeness in the audit findings; as well as stratified sampling, showcasing how it enhances audit efficiency by categorizing data into relevant strata. Lastly, we will review the importance of software and tools in modern IS auditing, emphasizing how technology aids auditors in executing more precise and effective sampling strategies.
Next, we will discuss the key principles that help determine sufficient sample sizes in IS audits. We will also cover the concept of confidence intervals, an essential statistical tool that helps auditors understand the range within which the true value of the population parameter lies. We will cover the interplay between sample size, risk, and materiality, and how these factors influence the auditor’s decision-making process. Lastly, we will address the challenges and implications of sampling errors in IS audits, such as selection and measurement errors. We will also discuss the strategies for mitigating sampling errors that enable IS Auditors to reduce the likelihood and effect of these errors in their audit work. In doing so, evaluating and reporting sampling errors will be emphasized, highlighting the auditor’s responsibility to communicate these aspects transparently.
Sampling Methods in IS Auditing
Audit sampling emerges as a vital tool in IS auditing, where data volumes can be massive and resources are limited. It is a systematic technique used to examine a subset of data or transactions within a population to conclude the entire dataset. It allows auditors to assess the effectiveness of controls, identify anomalies, and detect errors or irregularities without the need to examine every single transaction or piece of data. This is especially crucial when dealing with extensive datasets that would be impractical to review. By selecting a representative sample, auditors can focus on areas of higher risk or greater significance, optimizing resource allocation. This ensures auditors can conduct thorough audits while efficiently managing time and resources.
Audit sampling is closely linked to risk assessment and materiality considerations as it enables IS auditors to assess the level of risk within a dataset and determine whether errors or irregularities are material enough to impact the overall audit conclusions. High-risk areas may warrant larger sample sizes or more intensive testing, while lower-risk areas may require less extensive sampling. As we know, materiality is a measure of the significance of an error or omission and guides auditors in determining how much evidence is needed. The riskier the audit area, the more evidence we require, leading to larger sample sizes. Conversely, in areas with lower risk, smaller samples may suffice. This relationship is crucial in tailoring the audit to the specific context of the audited entity.
The first method we encounter is statistical sampling. This approach relies on probability theory, ensuring that each element in the population has a known chance of being selected. Its beauty lies in its ability to provide auditors with a quantifiable measure of sampling risk. This risk, the probability that the sample may not represent the population accurately, is a fundamental concept in auditing. The three primarily used statistical sampling approaches include:
Random sampling stands on the principle of equal chance, where every item in the population is equally likely to be selected, ensuring a bias-free approach. Tools and software are often employed to aid this process, bringing in precision and efficiency that manual methods cannot match. Random sampling’s strength lies in its simplicity and fairness, making it a widely accepted method in IS auditing.
Stratified sampling enhances audit efficiency by dividing the population into subgroups or strata. This technique is particularly effective when dealing with heterogeneous populations as it ensures that each stratum is adequately represented in the sample, providing a more accurate view of the entire population.
Systematic sampling, in which an interval (i) is first calculated (population size divided by sample size), and then an item is selected from each interval by randomly selecting one item from the first interval and selecting every ith item until one item is selected from all intervals. Efficiency is a significant advantage, especially when auditing extensive datasets, as it enables auditors to review a sample while maintaining a structured approach. Systematic sampling assumes a uniform data distribution without patterns or anomalies that could skew results and is not an ideal method to use when data exhibits systematic patterns or clustering.
Contrastingly, non-statistical sampling, often used in IS auditing, does not involve this statistical theory. Here, the auditor’s professional judgment is paramount. While this method may not provide a quantifiable measure of risk, it allows for flexibility and adaptability in diverse auditing environments. It’s particularly useful when dealing with complex information systems where specific risks are identified through auditor expertise. The three primarily used non-statistical sampling approaches include the following:
- Judgmental sampling heavily relies on the auditor’s experience and knowledge. In situations where certain aspects of the system are deemed more critical, this method allows auditors to target these areas specifically. It’s an approach where intuition, honed by years of experience, plays a key role. However, auditors must remain vigilant to avoid biases that can skew the audit results.
- Block sampling begins with IS auditors partitioning the dataset into distinct blocks or groups based on specific criteria such as transaction types, periods, or data categories. Rather than randomly selecting individual items or transactions, auditors choose entire blocks for examination. The selection is guided by auditors’ judgment, considering risk, materiality, and audit focus. It allows auditors to concentrate efforts on specific areas of interest, making it suitable for targeted reviews of critical data subsets.
- Haphazard sampling allows auditors to select items without any predetermined pattern or criteria. The selection process relies on auditors’ discretion and can involve simply picking items at random or based on convenience. This approach offers a straightforward way to gather a sample for review, especially when auditors are dealing with a limited dataset or when a formal sampling method may be unnecessary due to the nature of the audit.
It is important to remember that sampling in IS auditing provides a reliable basis for making informed decisions about the information system being audited. Hence, the choice of sampling method should align with the audit’s objectives, the nature of the population, and the specific risks involved. Moreover, as technology advances, the complexity of information systems auditing has escalated, and software tools enable auditors to handle large volumes of data efficiently and accurately. They bring sophistication to sampling methods, allowing auditors to perform more complex analyses and derive more nuanced insights.
Determining the sample size in an IS audit is a critical step that balances thoroughness with efficiency. The process begins with a clear understanding of the audit’s objectives. It’s not about choosing a large sample for comprehensiveness; it’s about choosing the right size to meet our specific IT audit objectives. Understanding confidence intervals is integral to this process. A confidence interval is a range within which we expect the true value of a population parameter to fall. It’s a concept that injects a degree of scientific rigour into our audit conclusions. The width of this interval is influenced by the sample size – larger samples generally result in narrower confidence intervals, offering greater precision. However, larger samples also mean more resources and time. Thus, the auditor must strike a balance, ensuring the sample is sufficient to provide reliable results without being unnecessarily large.
In testing controls (evaluating management’s processes), the IS Auditor applies the following guidance in determining the sample size.
Frequency of the Control | Reasonable Assurance from Test of Controls | Limited Assurance from Test of Controls |
---|---|---|
Application Controls |
1 |
1 |
Annually |
1 |
1 |
Monthly & Quarterly |
2 |
1 |
Weekly |
5 |
2 |
Between Weekly & 250 times |
10% of population (Min. of 5) |
5% of population (Min. of 5) |
Daily |
25 – 60 |
10 – 20 |
> 250 instances or Ad-hoc |
25 – 60 |
10 – 20 |
In performing substantive testing (evaluating the underlying activities instead of relying on management’s processes), the IS Auditor will generally test between 1% – 5% of the population with an upper cap of 500 samples. Sampling guidance may vary based on the IS Audit functions’ risk appetite and philosophy.
Sampling Errors and Their Impact on Audit Conclusions
Sampling errors occur when the selected sample does not accurately represent the entire population. This misrepresentation can lead to incorrect conclusions about the system being audited. The primary goal of IS Auditors is to provide accurate and reliable insights into the systems we examine. Sampling errors pose a significant risk to the integrity of the IS auditing work, and it is essential to recognize these errors and understand their potential impact.
There are various types of sampling errors, each with its characteristics and implications. One common type is the selection error, which arises when the method used to select the sample introduces bias. For example, choosing a non-random sample that warrants random selection can lead to skewed results. Another type is the measurement error, which occurs when there is a flaw in how information is collected or recorded. This type of error can significantly distort audit findings. The impact of sampling errors on audit quality and reliability cannot be overstated. When these errors are present, the audit conclusions drawn may be flawed, leading to misguided decisions by stakeholders. This outcome can have far-reaching consequences, especially in high-stakes environments where accurate and dependable audit results are crucial. Therefore, it is imperative for auditors to take steps to minimize the occurrence of these errors.
Mitigating sampling errors involves several strategies. Firstly, careful planning and designing of the sampling process are crucial. This planning includes selecting the appropriate sampling method and ensuring the sample size is adequate for the audit objectives. Secondly, auditors must apply their professional judgment and expertise in executing the sampling plan. This expertise involves being vigilant for signs of potential bias or inaccuracies during the sampling process. Similarly, evaluating and reporting sampling errors is another critical aspect. As auditors, we must identify and mitigate these errors and transparently communicate them in our audit reports. This transparency ensures that stakeholders are aware of the limitations of the audit findings and can interpret the results within the correct context.
In the Spotlight
For additional context on the role and importance of audit sampling, please read the article titled “Audit Sampling” [new tab].
Henderson, K. (2023). Audit sampling. Wall Street Oasis. https://www.wallstreetoasis.com/resources/skills/accounting/what-is-audit-sampling
Key Takeaways
Let’s recap the key concepts discussed in this section by watching this video.
Source: Mehta, A.M. (2023, December 6). AIS OER ch 03 topic 05 key takeaways [Video]. https://youtu.be/os1wvwFFtqE
Knowledge Check
Review Questions
- Explain the importance of selecting the right sampling method in IS auditing. Provide an example of a situation where you would choose a statistical sampling method over a non-statistical one, and vice versa.
- Explain the concept of sample size determination in IS auditing. How does the level of risk in an audit area influence the choice of sample size?
- What are sampling errors in IS auditing, and how can they impact audit conclusions? Provide an example of a sampling error and its potential consequences in an IS audit.
- What are the key differences between statistical and non-statistical sampling methods in IS auditing, and when would you use each?
- How does an IS auditor determine the appropriate sample size for an audit, and what role do confidence intervals play in this process?
- What is a sampling error in the context of IS auditing, and how can it affect the audit’s conclusions? Give an example.
Essay Question
Sampling methods based on auditors' judgment without the use of statistical theory.
Stratified sampling enhances audit efficiency by dividing the population into subgroups or strata. and is particularly effective when dealing with heterogeneous populations as it ensures that each stratum is adequately represented in the sample, providing a more accurate view of the entire population.
Sampling methods based on probability theory, used to provide a quantifiable measure of sampling risk.
Random sampling stands on the principle of equal chance, where every item in the population is equally likely to be selected, ensuring a bias-free approach.
Systematic sampling, in which an interval (i) is first calculated (population size divided by sample size), and then an item is selected from each interval by randomly selecting one item from the first interval and selecting every ith item until one item is selected from all intervals.
Relies on the auditor's experience and knowledge. In situations where certain aspects of the system are deemed more critical, this method allows auditors to target these areas specifically.
Block sampling begins with IS auditors partitioning the dataset into distinct blocks or groups based on specific criteria such as transaction types, periods, or data categories.
Haphazard sampling allows auditors to select items without any predetermined pattern or criteria. The selection process relies on auditors' discretion and can involve simply picking items at random or based on convenience.
The specific goals and outcomes an IT audit is designed to achieve.
Testing for the actual existence of a financial statement item to ensure its validity and correctness.
The level of risk an organization is willing to accept in pursuit of its objectives.