1.4 Longitudinal Birth Cohort Studies for Characterizing Mental Illness
Christina Fodtchouk
LEARNING OBJECTIVES
Longitudinal Birth Cohort Studies
- Understand that a birth cohort study design falls under longitudinal study design which falls under prospective study design which falls
- What exactly a prospective longitudinal birth cohort study is
- Understanding why these study designs are popular in mental health (psychological) research
- The overall outline of the study design for the 2020 study analyzed
- Limitations of this particular longitudinal birth cohort study
- Strengths of this particular longitudinal birth cohort study
There are various study designs that are used when it comes to psychology research of mental health illness. One of the common designs that provide unique insights is longitudinal studies (Kendra Cherry, 2020). This is the case because when looking into how an individual’s mental health changes over time and if and or how it is affected by external factors, a study design that incorporates an observational technique, where the participants are followed over a particular duration of time and quantitative data is collected along the way, is a necessity (Lawrence A. Palinkas, 2015). Studying the changes in mental health over one day or a few weeks, and sticking to strict quantitative data collection is not sufficient to compile valid conclusions.
The “Child maltreatment and mental health problems in 30-year-old adults: A birth cohort study” that was conducted by Kisely et al. 2020, was a prospective longitudinal study, and a birth cohort study as well. Overall, creating this longitudinal birth cohort study design falls under the prospective research branch (Figure 1), and all of this is ideal for mental health research. Nonetheless, as beneficial as it may present itself to be, there are many limitations that follow with this method of design, as well as, this specific area of research – studying mental health decline from childhood to adulthood.
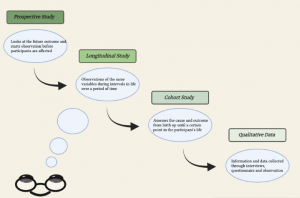
For the particular study that was published in 2020 by Kisely et al:
Limitations in the Study Design
- Due to the large sample size ( initially 7214 participants at birth) and being conducted over 30 years made it fairly expensive
- Due to it being over the duration of 30 years there is a risk of attrition
- Due to this prospective data being about 20-30 years old, there may be inconsistencies with the definitions of maltreatment
- Examined only notified abuse, did not look further for unrecorded data
- Data was recorded only at the time medical practitioners were available to report abuse, hence, could be underreported
- Prospective data of maltreatment was only collected from one university (Mater-University of Queensland Study of Pregnancy)
- Insufficient data and considerations for prenatal confounding factors that could have affected the mental health of the unborn children
- Potential inaccuracy during the particular CIDI-Auto (WHO Composite International Diagnostic Interview) protocol due to no physical practitioner assessing the individual
- Record of maltreatment throughout the years only collected from one child protection agency (Department of Families, Youth and Community Care)
- Not everyone may have easy access to technology to answer the questions from the CIDI-Auto protocol
- Lack of in-person interviews with practitioner results in no follow up questions that are thorough and specified to the individual
- There may be a participation burden which results in low recruitment and retention of participation (Zhong-Cheng Luo et al., 2011)
- A lot of personal time of the researchers needs to be allocated towards such studies
Strengths in the Study Design
- Avoids recall bias by having retrospective recall
- Used a prospective population-based survey which allows it to be more generalized to the greater population
- Periodic follow up at birth, 6 months, 5, 14, 27, and 30 years old (longer than pre-existing studies)
- Robust evidence (longitudinal), can be adjusted as time goes on
- No personal interviews may allow for less embarrassment and more honesty
- Controls for confounders (social determinants of health such as age, socioeconomic status, education, and environment)
- Differentiates between different types of child abuse (emotional/physical/sexual)
- Differentiates between different types of mental illnesses (depression/anxiety/PTSD)
- Performed statistical analysis to assess the relationship between maltreatment and psychiatric outcomes
- Performed logistic regression analysis for the association between the independent (maltreatment) variable and dependent (mental health) variable
- Performed a sensitivity and propensity analysis to adjust for baseline characteristics (includes sociodemographic variables) at birth like the gender of the child, the race of parent, maternal age, maternal educations status, family income, and maternal relationship status
- Performed sensitivity and propensity analysis to adjust for baseline characteristics for the participants at 30 years old which were their income, educational achievement, marital status, and employment
- Performed record-linkage analysis which was no personal interviews and comparison of different records from the agency and university to gather a conclusion (access to other files and documents for references)
KEY TERMS
- Attrition: slowly losing interest and dropping out over time
- Confounding Factors: may affect the variables throughout the study – age, weight, height, education, etc.
- Participation Burden: the pressure participants feel to adhere and participate in the study
- Recall Bias: the inaccuracy of past thoughts, memories, and feelings during the time of recollection
- Retrospective Recall: information from documents compiled in the past
- Robust: strong and credible
- Statistical Analysis: assessing the significance of the relationship between the different variables
- Logistic Regression Analysis: assessing the probability of a certain event occurring
- Propensity Analysis: controlling the effects certain variables may have on the data found
- Sensitivity Analysis: assessing how much of an effect certain variables had on the data found
- Record Linkage Analysis: bringing together different data from different places on the same topic