6.5 Levels of AI Integration in Learning and Assessment
Levels of AI Integration in Learning and Assessment
Based on your analysis of learning outcomes and the potential impact of Generative AI on each outcome, you should be able to determine the appropriate level of AI Integration for different learning activities and assessments. Your choice may range from No AI Allowed to full AI Exploration.
|
Level of AI Integration |
Description |
1 |
No AI |
The assessment is completed entirely without AI assistance in a controlled environment, ensuring that students rely solely on their existing knowledge, understanding and skills. |
2 |
AI Planning |
AI may be used for pre-task activities, such as brainstorming, outlining, and supporting initial research. Assessments emphasis the ability for students to develop and refine AI-generated ideas independently. |
3 |
AI Collaboration |
AI may be used to help complete the task, including pre-task activities (as above), drafting, feedback, and refinement. Students are responsible for evaluating and modifying the AI outputs. Students must document their use of AI. |
4 |
AI Exploration |
AI should be used creatively to complete the task. Part of the assessment outcomes is developing AI Literacy, or the ability to use AI tools effectively and critically. |
Once you’ve determined the appropriate level of AI integration, based on the intended learning outcomes of an assessment or task, there are two key considerations in supporting your choices:
- Providing clear messaging on acceptable or expected use of Generative AI for the assessment or task.
- Making deliberate design choices that reflect the acceptable use of Generative AI selected.
No AI: Creating AI-resistant Activities and Assessments
Using generative AI to accomplish some course Learning Outcomes may prevent students from learning how to independently demonstrate those outcomes. Once you have identified these outcomes, it’s important to be explicit in the assessment instructions (and syllabus) that AI is not to be used. Explaining why the decision was made not to allow the use of generative AI tools and the effect of using them on students’ learning will also discourage students from using these tools when they shouldn’t be.
Below we will examine different approaches to creating AI-resistant assessments.
This section uses the following terms and definitions:
- Authentic assessment: assessment tasks that reflect real-world applications of knowledge.
- Alternative assessment: assessment designs that differ from traditional standardized assessments and encompass a wide variety of methods, formats, and tools.
Authentic Assessment
Authentic assessment is one approach to designing AI-resistant assignments. Authentic assessments typically ask learners to apply key course concepts to real-world (“authentic”) situations or tasks.
Inherently, these tasks:
- are more messy or complex than traditional assessments
- lack one clear “correct” answer
- require a range of skills and knowledge
- are iterative and approach learning as a process
- are situated in a specific context
- mirror authentic conditions, tasks, or requirements of professional workflows
For more information on Authentic Assessment, see this resource from Indiana University Bloomington.
Recall from that the limitations of current generative AI models means that they will not easily be able to accomplish complex, situated, and iterative tasks without user intervention.
Using Authentic Assessment to Reduce Academic Misconduct
In addition to presenting learners with tasks that aren’t ideal for generative AI use, the use of authentic assessment also increases learner motivation and interest, which can reduce the urge to engage in academic misconduct.
- By focusing on authentic tasks, learners will see relevance to future employability and the development of skills for success in their careers
- By focusing on process rather than a final product, learners can see iterative improvements and focus on the academic skills being developed at each stage of the process. This also means that authentic assessments can be ideal for scaffolding.
For more information see Assessment Strategies to Reduce Academic Misconduct.
Traditional VS Authentic Assessment
The following table provides a clearer comparison between traditional assessment and authentic assessment and highlights how different characteristics of authentic assessment make it more AI resistant than traditional designs. This can be helpful in guiding how you make changes to your own assessments.
Traditional Assessment |
Authentic Assessment |
What Makes it Authentic |
What Makes it AI Resistant |
Requires right answer |
Requires high-quality performance or product, along with justifications of decisions. |
Students must be able to think through why they made decisions that resulted in the final product. |
Requires application of knowledge, not just recall, including reflections on choices made |
Questions must be unknown to students in advance |
Instructions/questions/purpose must be known to students in advance. |
Tasks that are to be judged should be known ahead of time. Rubrics should be provided. |
Emphasis moved from memory recall and content reproduction to discussion and application of knowledge |
Disconnected from the real world |
Tied to real-world contexts and constraints. Requires students to solve realistic problems. |
Task is similar to what would be encountered by a real-life practitioner. |
When students see the need for the skill or knowledge for their future success, they are more likely to participate and not use generative AI; generative AI can also struggle to situate responses within specific contexts |
Isolation of skills, focus on facts |
A range of skills/knowledge need to be integrated in order to solve a problem. |
Tasks are multi-step and multifaceted. |
Generative AI is about product not process so its utility is lessened when steps are emphasized over grading the outcome |
Easily scored |
Includes complex tasks for which there may not be a right answer. |
Meaningful assessment and feedback is emphasized. |
When creation and feedback are personalized greater value for individual completion is communicated and AI becomes less appealing; AI may be able to act as a scaffold in these takes, aiding rather than impeding learning |
“One shot” approach |
Iterative in nature. |
Knowledge and skills are used in more than one way. |
AI faces difficulty in handling iterative, evolving tasks that require adaptability and varied approaches. |
Given a score |
Opportunity to provide diagnostic feedback. |
Designed to give practical experience and improve future performance. |
Feedback can provide learners with specific, actionable steps for improvement, encouraging them to be more engaged in the learning process |
This table has been adapted from Toronto Metropolitan University’s resource on Alternative Assessment
Alternative Assessment: Other AI Resistant Approaches
Authentic Assessment is just one type of alternative assessment. There are other approaches to assessment that can also minimize generative AI use by creating conditions that makes the use of generative AI ineffective or by reducing stressors that lead to academic misconduct.
LIVE ASSESSMENTS
Student communication and content knowledge can be assessed by having learners present content live in a room or on a video conferencing platform when access to Generative AI tools is limited. Examples: presentations, in-class groupwork, oral exams, live discussions, seminars, proctored exams, etc.
COLLABORATIVE ASSIGNMENTS
Having students work together to compile a resource on a specific topic may also reduce the use of generative AI because the differences in voice would be difficult to replicate. Other dialogic or collaborative assessment designs, such as the use of forums, social annotation tools, or group projects, may also reduce the use of generative AI, especially if groups are asked to document their assignment progress and process.
REFLECTION
Asking students to engage in reflective practices, such as writing learning journals reflecting on their own learning or engagement in a task or making connections to their own experiences, makes the learning more meaningful to learners. AI tools may be able to generate generic reflection type outputs but would struggle to make specific connections to a learners’ own experience without a lot of context and careful prompt engineering.
Authentic assessments help limit the use of generative AI as the complex, situated, and iterative nature of many authentic assessments make generative AI an inadequate tool for completing these tasks. These types of assessments also result in higher student engagement and lower instances of academic misconduct because they offer a continuous feedback loop and the opportunity to develop real world knowledge and skills.
Other alternative assessment techniques can also be used to reduce the utility of generative AI by:
- shifting assessment from lower-level cognitive tasks to more complex production and application tasks
- using alternative formats that are not conducive to the use of generative AI
- inviting personalization in assessment through reflection and narrative
AI Planning
Generative AI can help support learners in planning their approach to assessments. It can be useful for:
- managing their time by helping them break down complex tasks into manageable chunks
- identifying a topic or refining ideas to an appropriate scope
- generating research questions
- identifying useful resources to support research
Generative AI can be helpful to support executive functioning, including setting goals and project and time management. For example, students could use Generative AI to create a project plan for larger assignment, to break process-based assignments into manageable tasks, or to help with setting short term goals. These tasks could be a barrier for some learners if they are not explicitly part of the assignment objectives or if they have not been taught.
In the example below, CoPilot generated a step-by-step guide to writing a research paper.
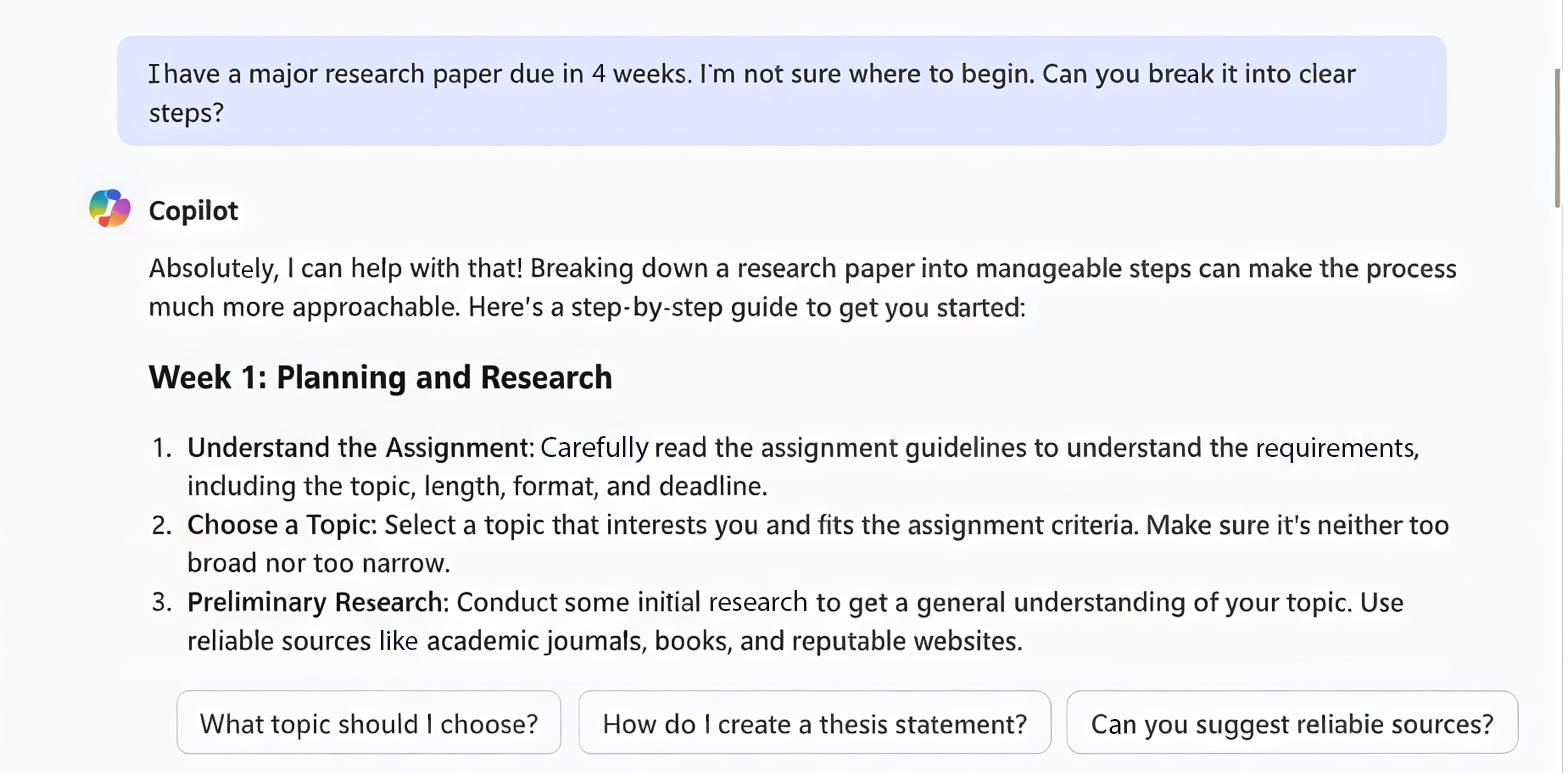
Idea generation can present a barrier early on in a process-driven assessment. Students may spend too much time trying to decide on a topic, have too many ideas to explore all of them, or just need a place to start so that they can move on to later stages of the process. Since generative AI tools are trained on large data sets, they can often be used for initial ideation when approaching a task. Using them in this way can provide students with content to respond to and refine rather than generating ideas from scratch. Students can develop ideas generated by LLMs into more robust or context-appropriate topics. In the example below, CoPilot narrowed down the broad topic of “Social Determinants of Health” to more specific topics appropriate for a 10-minute presentation.
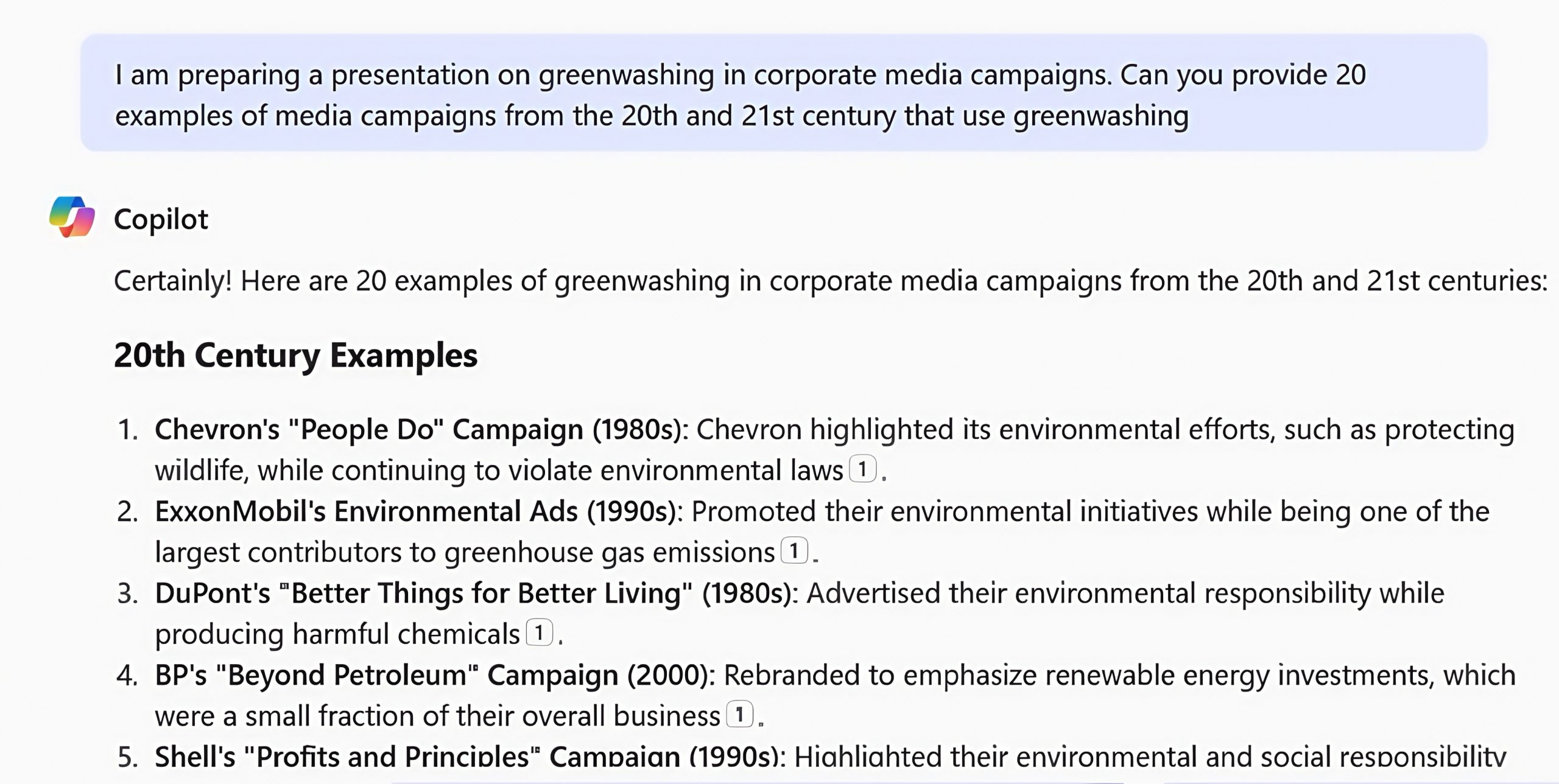
These are just some examples of how Generative AI can help learners get started on an assignment, removing unnecessary barriers in the planning process. However, it’s important to keep constructive relevance in mind when deciding if Generative AI can be used in this way. If learners use Generative AI to complete any of these planning tasks, are they still achieving the intended learning outcomes?
AI Collaboration
Generative AI technologies can be used to support learners at other steps in process-based assignments.
Process-based assignments require a series of tasks usually completed in a specific order. As you look at the following example of a writing process, consider what skills are required at each step in the process. Where could generative AI support learners without detracting from the intended learning outcomes of completing the final paper? Does this change if students have already successfully demonstrated the required skills for this step?
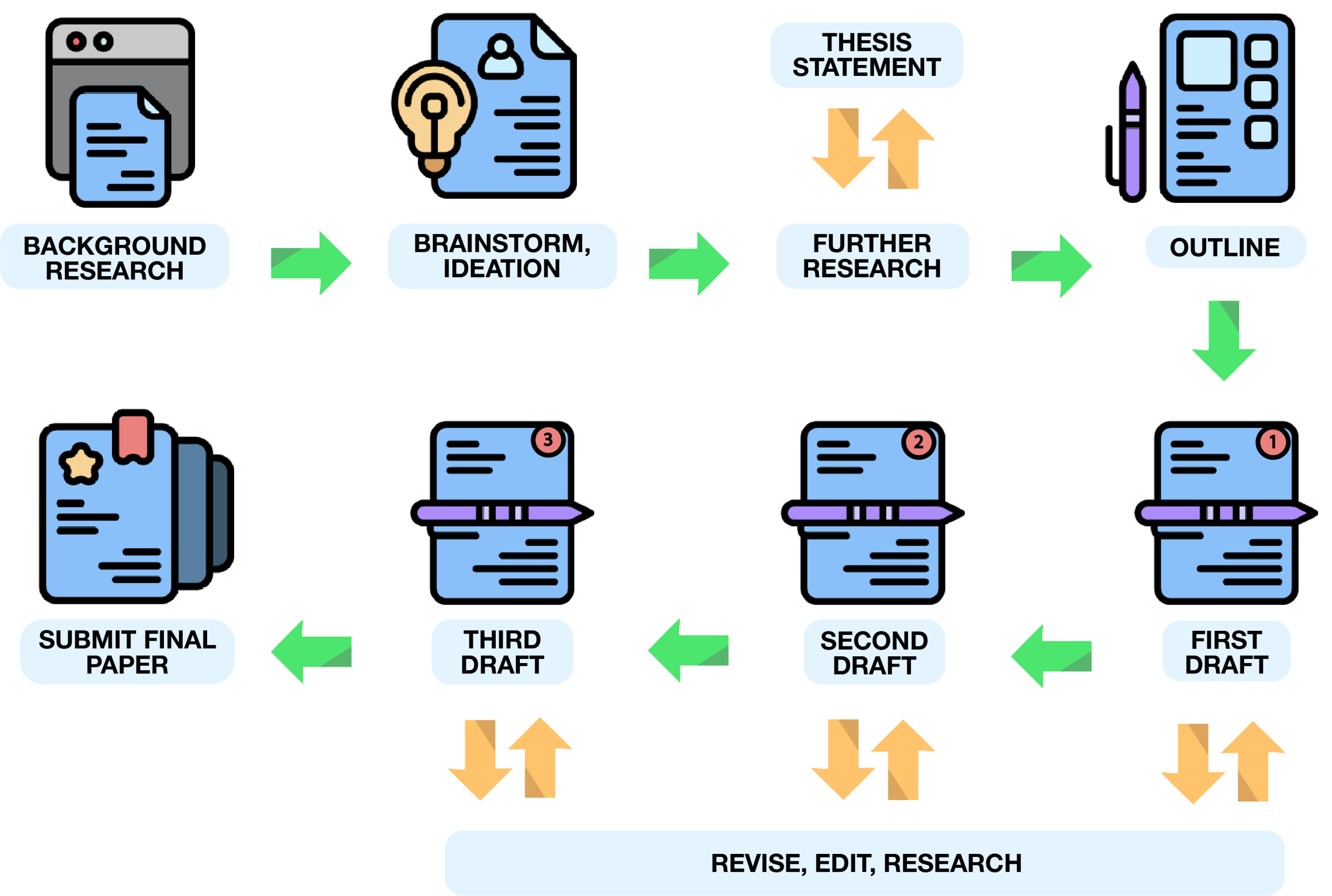
Generative AI is particularly strong at written coherence and can be a useful tool for providing feedback on writing. Rather than making the revisions (replacing learning), Generative AI can be used to identify strengths and areas for improvement and suggest changes.
Example Prompt: You are an advisor in the university writing centre. You will give feedback on my paper. You will provide balanced feedback, highlighting both strengths and areas for improvement. Please pay specific attention to clarity of my writing and the completeness of my arguments. You will not provide or offer revisions, but if I suggest a revision, you will offer further feedback to help me refine my writing.
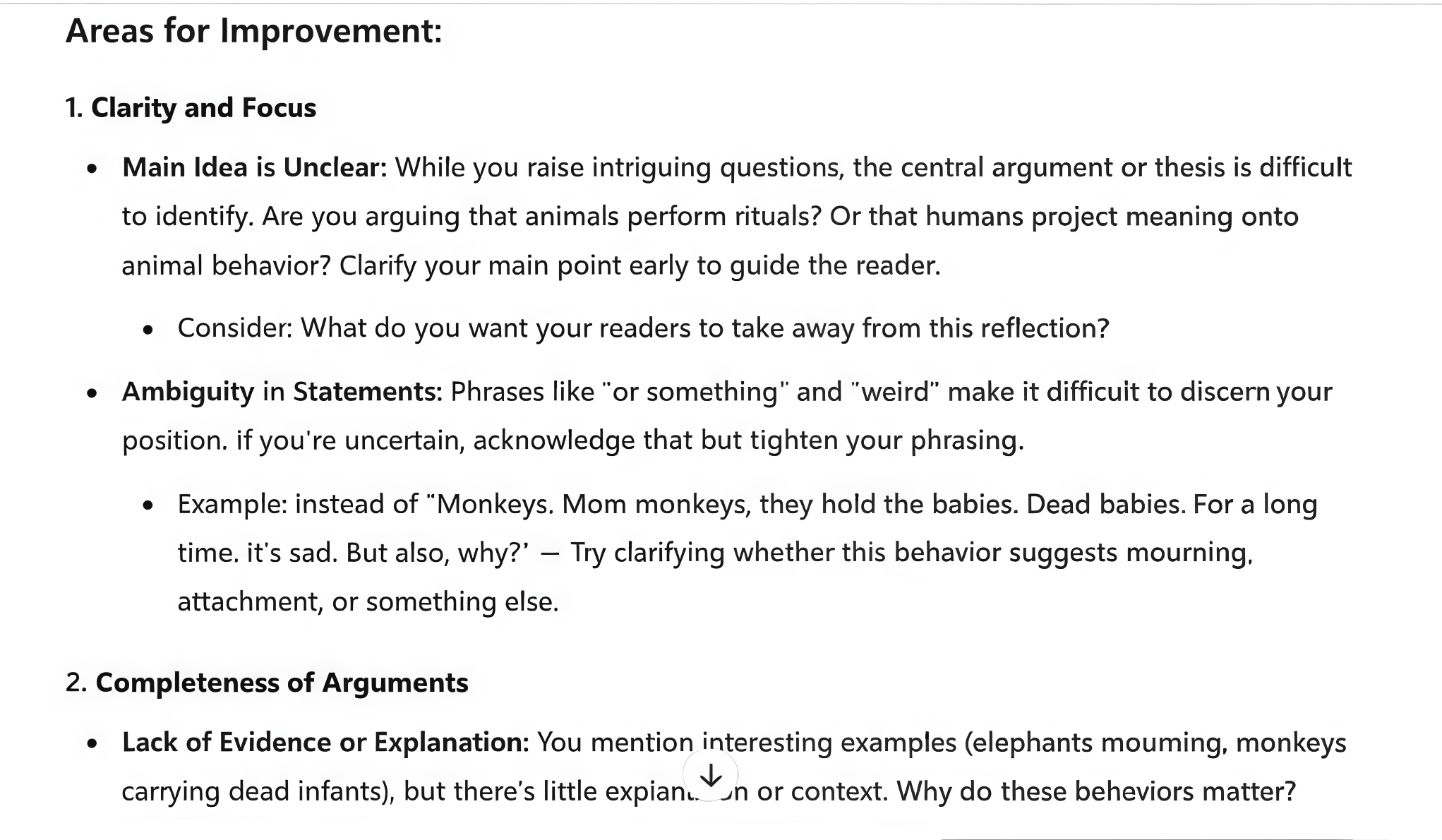
Documenting AI Use
If you allow learners to use Generative AI throughout an assignment, it is important to give clear instructions on where Generative AI can and cannot be used and how students should document and cite their use of Generative AI.
Popular citation guidelines have been updated with guidelines for citing Generative AI.
APA Citation
Name of Company/creator of generative AI Tool. (Year). Name of the generative AI program (model of program) [Large language model]. URL.
Example:
Microsoft. (2025) CoPilot (GPT-4). [Large language model]. https://copilot.cloud.microsoft
MLA Citation
Author/Creator. “Name of chatbot.” Title of platform where accessed, Full URL, Date Accessed (optional).
Example:
Microsoft. “CoPilot”. CoPilot. https://copilot.cloud.microsoft March 25, 2025.
See the University of Waterloo’s Citation Guide for more details.
You can also state requirements on how you want students to document their use of Generative AI in completing the assignment.
Consider the following questions:
- How much detail do you require?
- Do you want students to submit the full dialogue? Do you want them to indicate the types of tasks that they used Generative AI for?
The University of Waterloo has developed a tool for detailed documentation of Generative AI use. See this resource from the University of Waterloo for more information.
AI Exploration
Generative AI and other technologies are becoming more commonplace across professions and in our everyday lives. It’s important for learners to also understand how these technologies work and the limitations that they have. Otherwise, we risk normalising uninformed, and potentially harmful, use of Generative AI technologies.
This might mean supporting the development of AI Literacy in your courses and assessments or exploring how Generative AI tools are being used in your field by professionals.
As Generative AI is being more commonplace in social and professional practices, it will be important for learners to understand these technologies, by examining the sociopolitical elements of AI development and use, learning how to use certain tools, exploring how AI models are trained, or critiquing outputs for problems of accuracy, bias, or other distortions of information. Some example activities are provided below.
Understanding Algorithmic Bias
Ask students to use Generative AI in response to a prompt and examine the output for biases, including the lack of representation of approaches, concepts, cultures, economic standing, and more. This can create an excellent launch point for many discussions on the skills and knowledge needed for literacy and process checking.
Critically Evaluating Generative AI Output
Ask students to use Generative AI tools to produce work and using the SIFT framework (shared in the section on AI Skills) or another evaluation tool, to verify the accuracy of the output.
Authentic Assessment of AI Professional Practices
Identify if/how generative AI or other AI technologies are being used in professional settings related to your discipline or field. Create an authentic assessment that mirrors these processes or applications. Ask students to not only complete the assignment but to reflect on the use of generative AI in these settings.
A subset of Deep Learning that can use learned rules or patterns to generate new content.
An intentional action taken by an individual to deceive their instructor, peers, or institution, often in an attempt to achieve a higher grade, gain course credit, or otherwise be recognized for performance not achieved.
Computational models that are trained on huge datasets of text to recognize common patterns and relationships in natural language. They can be used for generating texts that mimic human language.