2.5 Bias & Misinformation
Bias-In, Bias-Out
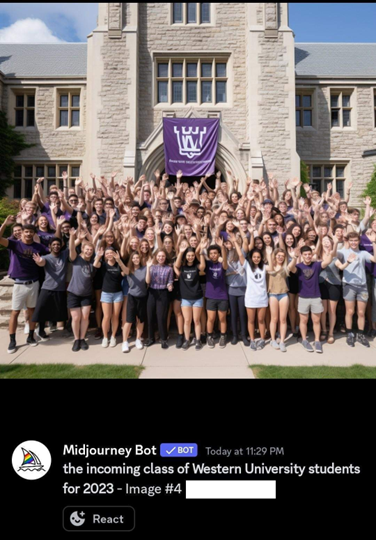
Many AI models are trained on data where social biases are present. These biases are then encoded into the patterns, relationships, rules, and decision-making processes of the AI and have a direct impact on the output.
Biased data can be easy to spot, such as in this AI generated image which shows a predominantly white class of 2023 at Western, but it can also be more invisible. AI-generated text will reflect dominant ideologies, discourses, language, values, and knowledge structures of the datasets they were trained on. For example, Large Language Models may be more likely to reproduce certain dominant forms of English, underrepresenting regional, cultural, racial, or class differences (D’Agostino, 2023 ).
The ethical issue is twofold: first, the information generated by Generative AI is more likely to reflect dominant social identities, meaning that students who use AI will not be exposed to certain worldviews or perspectives, and some students may not feel that their experiences and identities are reflected in the output. Second, the use of Generative AI to produce knowledge will continue to reinforce the dominance of these ideologies, values, and knowledge structures, contributing to further inequities in representation.
As an instructor, it’s important to be aware of this limitation of AI tools. If you ask your students to use these tools, it’s also important to teach them critical AI literacies to similarly be able to identify and reflect on these issues of representation, bias and equity.
Some Generative AI companies have taken steps to correct for biases in the training data by establishing content policies or other guardrails to prevent generating biased or discriminatory output. However, these guardrails are inconsistent and can be subject to the ethical standards of each Generative AI company.

Misinformation & Deception
The generation of fake, inaccurate, or misleading information through Generative AI could be unintentional or deliberate. AI can be used to generate fake news stories, fake datasets, or otherwise employed in attempts to deceive.
One of example of this is the use of text-to-image and text-to-video Generative AI tools to produce visual media for the purposes of (malicious or not) deception. A deepfake is the product of Generative AI that creates a believable but fake video, audio, or image. They often feature real people saying or doing something that they didn’t really say or do. Deepfakes do have potential benefits for the arts, for social advocacy, for education and for other purposes, but they do present ethical issues because often permission has not been received to use the person’s likeness and because it has the potential to spread misinformation or to mislead people.
Ethical Case Study: Misinformation & Deception
One of your course assignments asks students to produce a piece of speculative fiction reflecting on the future if immediate action isn’t taken in response to Climate Change. One student creates a video of a news report showing the world in crisis. Within the video, they have deep fakes of several world leaders justifying their lack of action over the past 10 years.
What ethical considerations are there around this use of AI?
Feedback
Deepfakes present a few important ethical issues, particularly with regards to misrepresentation, intention to deceive, and politics and political agendas. In this case, the student wasn’t necessarily attempting to deceive viewers, but it’s important to help students understand the ethics of Generative AI and the potential harms if you allow or encourage AI use in your courses.